A 3D orthogonal vision-based band-gap prediction using deep learning: A proof of concept
Computational Materials Science(2022)
摘要
In this work, a vision-based system for the electronic band-gap prediction of organic molecules is proposed using a multichannel 2D convolutional neural network (CNN) and a 3D CNN, applied to the recognition and classification of 2D projected images from 3D molecular structure models. The generated images are input into the CNN for an estimation of the energy gap, associated with the molecular structure. The public data set used in this research was the Organic Materials Database (OMDB-GAP1). A data transformation from the descriptive information contained in the data set to three 2D orthogonal images of molecules was done. The training set is composed of 30,000 images, whereas the testing set was composed of 7500 images, from 12,500 different molecules. The multichannel 2D CNN architecture was optimized via Bayesian optimization. Experimental results showed that the proposed CNN model obtained an acceptable mean absolute error of 0.6780 eV and root mean-squared error of 0.7673 eV, in contrast to two machine learning methods reported in the literature used for band-gap prediction based on conventional density function theory (DFT) methods. These results demonstrate the feasibility of CNN models to materials science routines using orthogonal images projections of molecules.
更多查看译文
关键词
Band-gap prediction,Deep learning,Vision-based regression
AI 理解论文
溯源树
样例
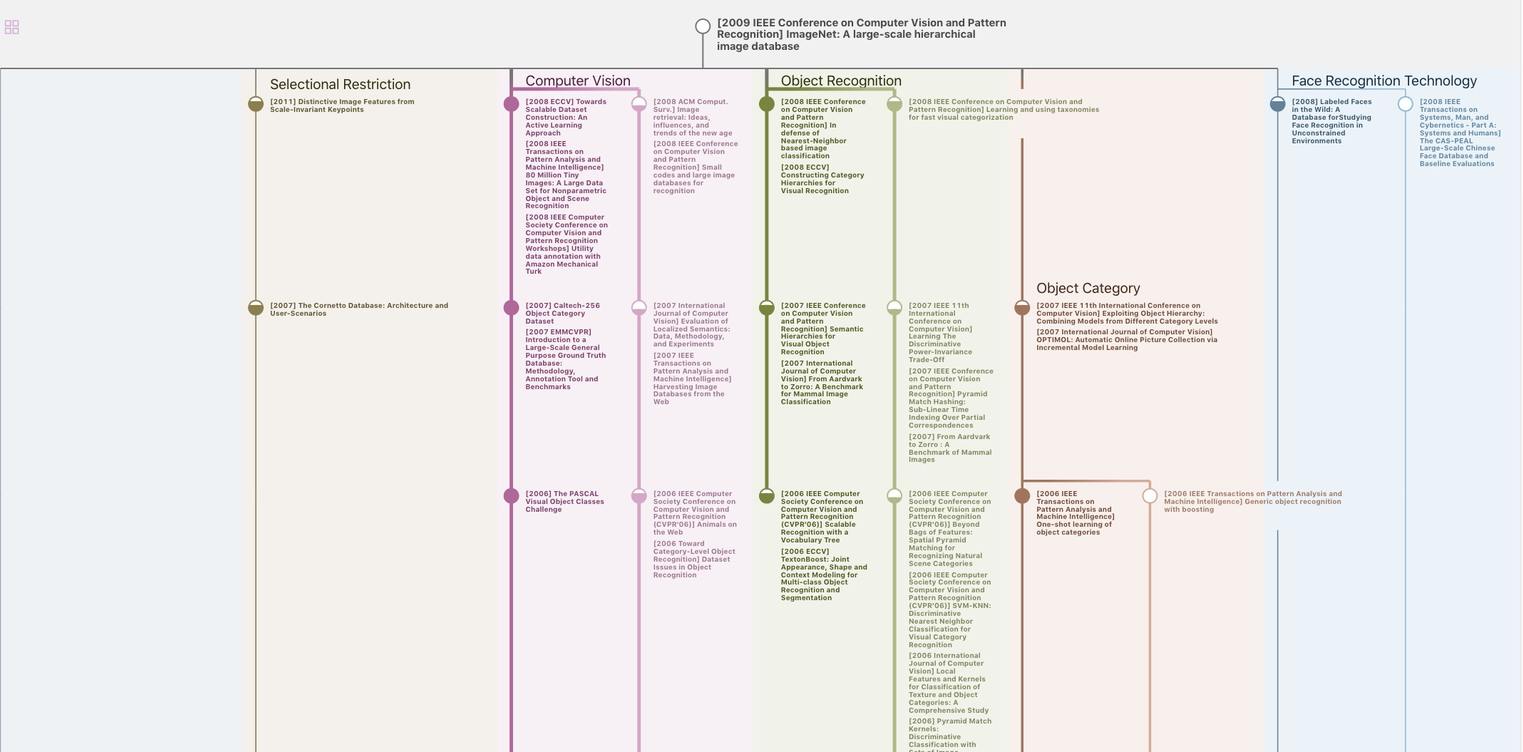
生成溯源树,研究论文发展脉络
Chat Paper
正在生成论文摘要