Permutation flow shop energy-efficient scheduling with a position-based learning effect
INTERNATIONAL JOURNAL OF PRODUCTION RESEARCH(2023)
摘要
Severe environmental problems have made green scheduling an emerging research hotspot. In this paper, a permutation flow shop energy-efficient scheduling problem that considers multiple criteria is investigated. The aim is to find the optimal job processing sequence and conveyor speed that minimise both the makespan and total energy consumption. In addition to two types of common criteria, namely, machine-based criterion (i.e. sequence-dependent setup time) and energy-based criteria (including both the transportation time control strategy and machine shutdown strategy), a human-based criterion (i.e. a position-based learning effect) is introduced. A bi-objective programming model is developed, and a multi-objective iterated greedy (MOIG) is designed to reach the Pareto front of the model. Considering that there are two types of decisions in the model (i.e. job sequence and conveyor speed), two algorithm alternatives are designed based on the job sequence and conveyor speed, respectively. Meanwhile, an acceptance criterion with advantages in terms of the convergence speed and solution diversity is proposed. Existing algorithms, including NSGA-II and MOEA/D, are introduced to evaluate the performance of the MOIG. The results emphasise the efficiency of the MOIG. Overall, the model and MOIG effectively improve the green efficiency of enterprises and can reasonably control operating costs.
更多查看译文
关键词
Permutation flow shop,energy-efficient scheduling,sequence-dependent setup time (SDST),learning effect,multi-objective iterated greedy (MOIG)
AI 理解论文
溯源树
样例
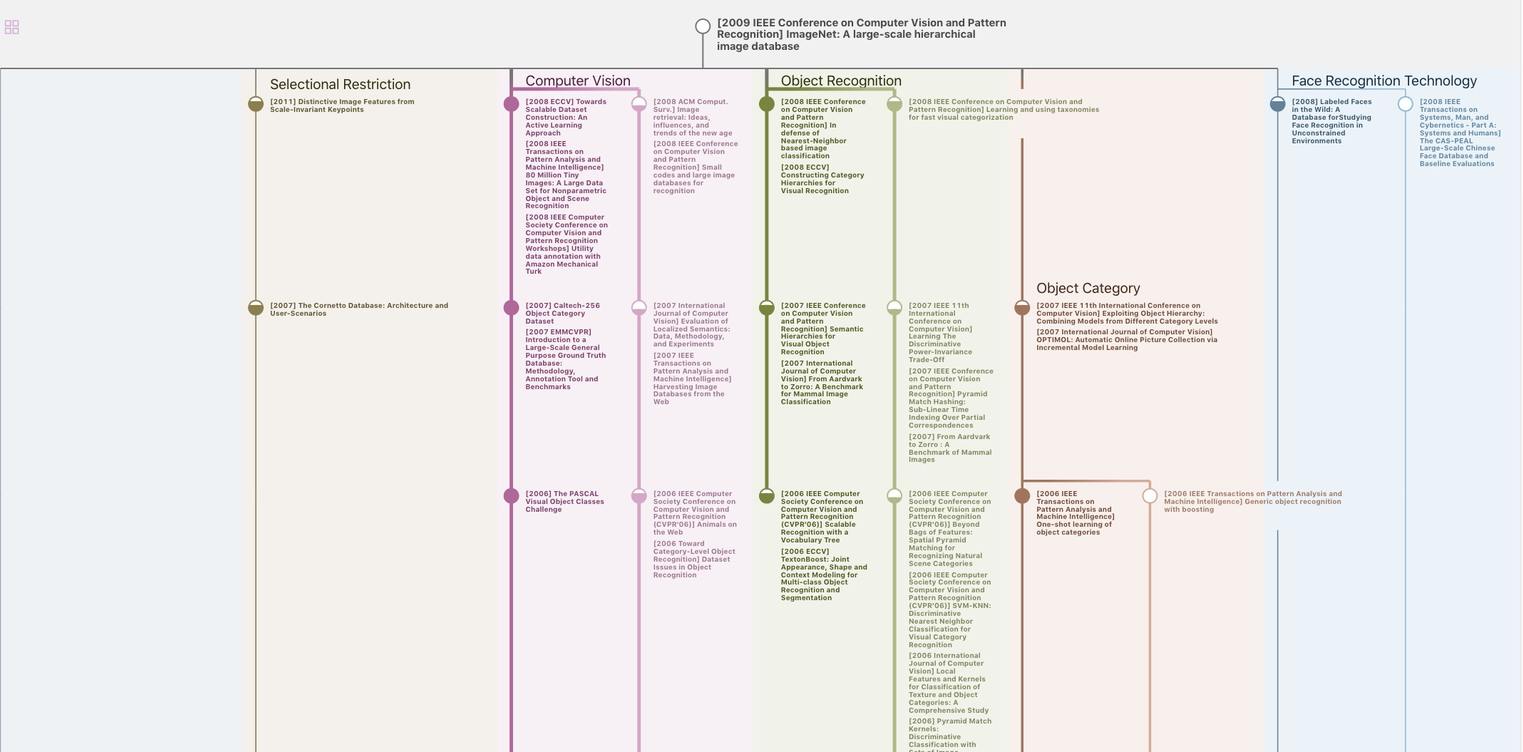
生成溯源树,研究论文发展脉络
Chat Paper
正在生成论文摘要