Defending Against Data Poisoning Attacks: From Distributed Learning to Federated Learning
COMPUTER JOURNAL(2023)
摘要
Federated learning (FL), a variant of distributed learning (DL), supports the training of a shared model without accessing private data from different sources. Despite its benefits with regard to privacy preservation, FL's distributed nature and privacy constraints make it vulnerable to data poisoning attacks. Existing defenses, primarily designed for DL, are typically not well adapted to FL. In this paper, we study such attacks and defenses. In doing so, we start from the perspective of DL and then give consideration to a real-world FL scenario, with the aim being to explore the requisites of a desirable defense in FL. Our study shows that (i) the batch size used in each training round affects the effectiveness of defenses in DL, (ii) the defenses investigated are somewhat effective and moderately influenced by batch size in FL settings and (iii) the non-IID data makes it more difficult to defend against data poisoning attacks in FL. Based on the findings, we discuss the key challenges and possible directions in defending against such attacks in FL. In addition, we propose detect and suppress the potential outliers(DSPO), a defense against data poisoning attacks in FL scenarios. Our results show that DSPO outperforms other defenses in several cases.
更多查看译文
关键词
distributed learning,federated learning,data poisoning attacks,AI security
AI 理解论文
溯源树
样例
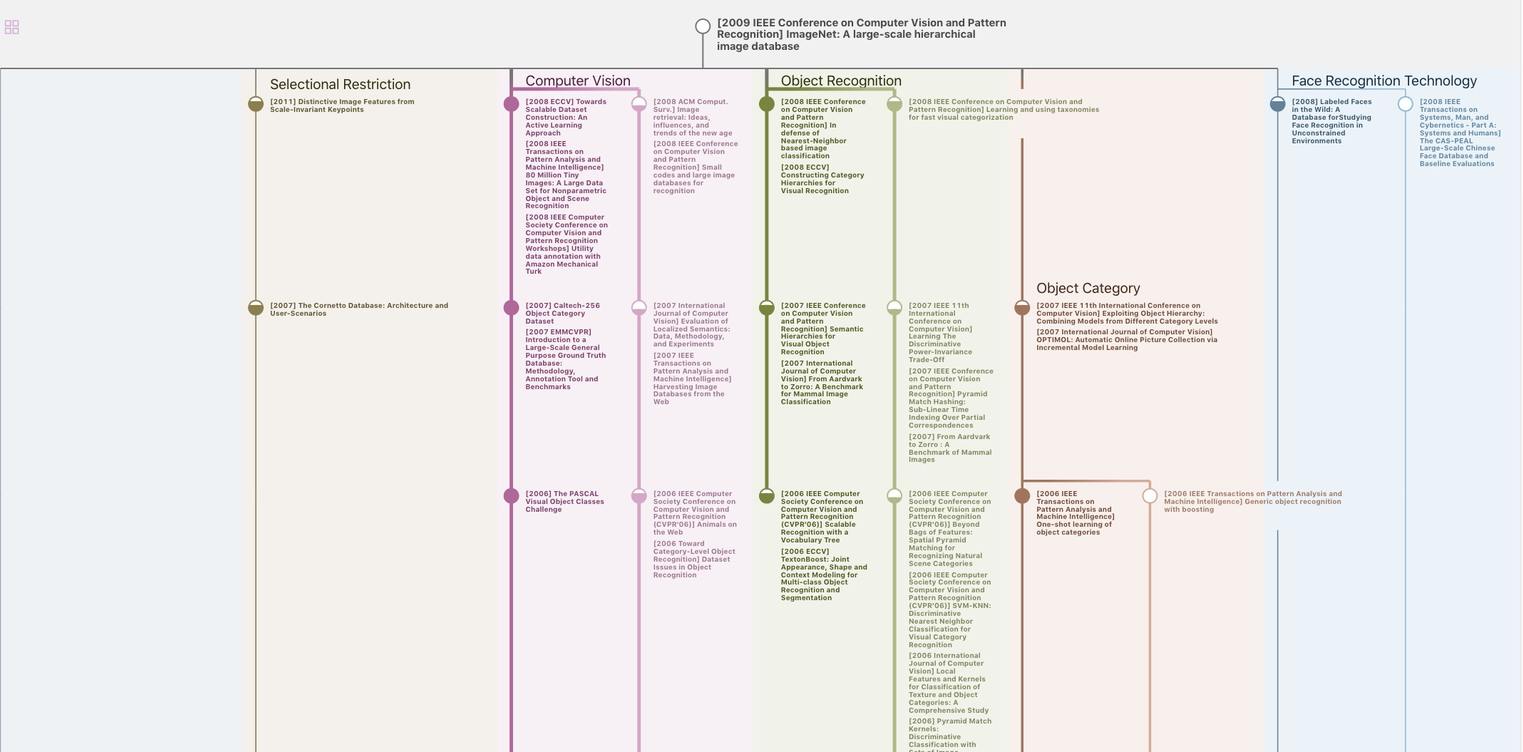
生成溯源树,研究论文发展脉络
Chat Paper
正在生成论文摘要