Optimization-based online estimation of vehicle mass and road grade: Theoretical analysis and experimental validation
MECHATRONICS(2021)
摘要
The gross vehicle mass (GVM) and the road grade are two factors that both have a substantial influence on the performance of a vehicle's powertrain. In this paper, we propose a novel model-based estimation method for the GVM and the road grade that exploits entire sequences of powertrain measurements at once and is formulated as a nonlinear program (NLP). The estimator is based on a simple model for the vehicle's longitudinal dynamics with only few intuitive vehicle parameters. By assuming the GVM to remain constant during certain sections of the trip and by describing the road grade profile in the distance domain, we achieve a separation of scales, which enhances disturbance rejection and significantly lowers the number of optimization variables. The resulting estimator is thoroughly analyzed both analytically and numerically. We show that a closed-form solution exists for the grade profile as a function of the GVM. Furthermore, if the GVM can be assumed to be constant during the journey considered, the estimation problem can be translated to a scalar NLP for finding the GVM. Although a rigorous proof is missing, our experiments show that in practice, the objective function is quasi-convex on a reasonable interval of GVM values and that thus a unique solution exists. Furthermore, robustness and sensitivity studies are conducted, where various perturbations are considered in a controlled environment, including uncorrelated and correlated noise, sensor offset, and model mismatches. Compared to two well-known recursive filters described in literature, our estimator shows significantly higher robustness with respect to all perturbations. Finally, we validate the estimator and the two recursive filters on real data from an electric city bus. The proposed estimator outperforms the recursive algorithms and achieves an average relative GVM estimation error of 3.4%. On a standard personal computer, the NLP for a driving phase of around one hour is solved in roughly 7.5 s, while the scalar NLP representing a driving phase of around 75 s is solved in roughly 12 ms. Both results indicate the real-time applicability of our algorithm.
更多查看译文
关键词
Vehicle mass estimation, Road grade estimation, Optimization
AI 理解论文
溯源树
样例
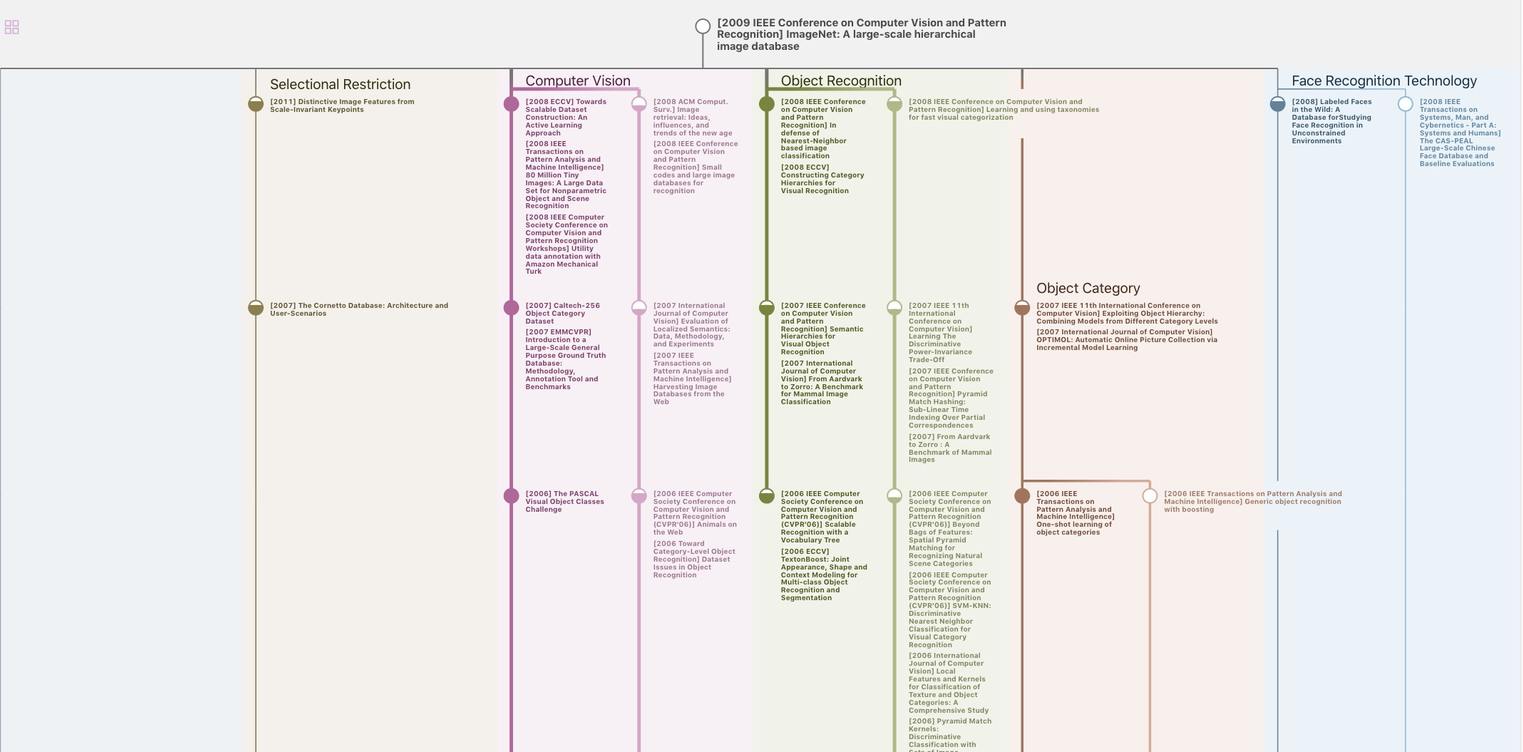
生成溯源树,研究论文发展脉络
Chat Paper
正在生成论文摘要