One Transistor One Electrolyte-Gated Transistor for Supervised Learning in SNNs
IEEE Electron Device Letters(2022)
摘要
Spiking neural networks (SNNs) are a powerful and efficient information processing approach. However, to deploy SNN on resource-constrained edge systems, compact and low power synapses are required, posing a significant challenge to the conventional silicon-based digital circuits in terms of area- and energy- efficiency. In this study, electrolyte-gated transistors (EGTs) paired with conventional transistors were used as the building blocks to implement SNNs. The one transistor one EGT (1T1E) synapse features heterosynaptic plasticity, which provides a flexible and efficient way to practice supervised learning via spike-timing-dependent plasticity. Based on this method, an SNN with spatiotemporal coding was implemented to recognize the handwritten alphabets, demonstrating 98.3% accuracy at 10% noise level with 5 fJ per synaptic transmission and 1.05 pJ per synaptic programming. These results pave the way for energy-efficiently neuromorphic computing in the future.
更多查看译文
关键词
Neuromorphic computing,electrolyte-gated transistor,SNNs,supervised learning
AI 理解论文
溯源树
样例
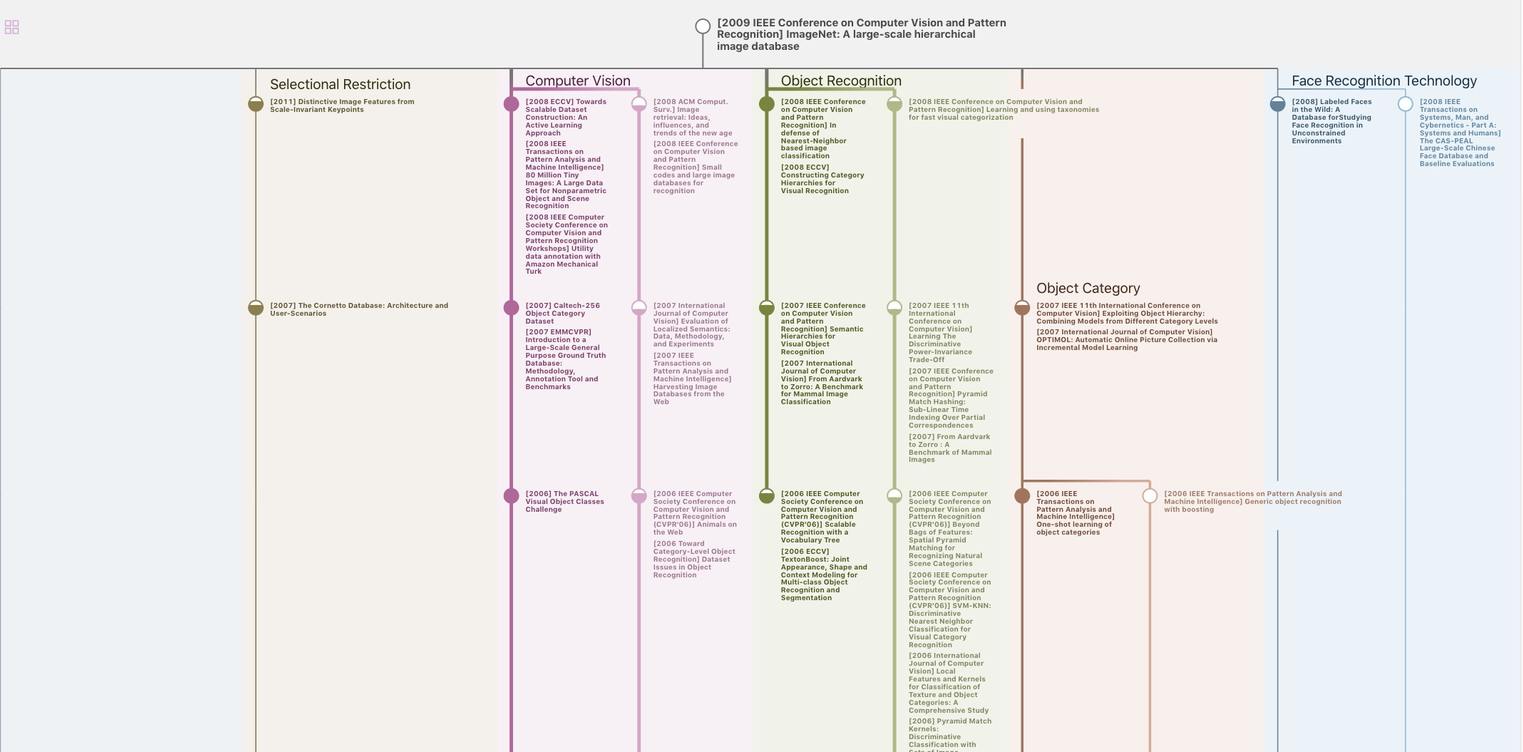
生成溯源树,研究论文发展脉络
Chat Paper
正在生成论文摘要