Energy Efficient Distance Computing: Application to K-Means Clustering
ELECTRONICS(2022)
摘要
Distance computation between two input vectors is a widely used computing unit in several pattern recognition, signal processing and neuromorphic applications. However, the implementation of such a functionality in conventional CMOS design requires expensive hardware and involves significant power consumption. Even power-efficient current-mode analog designs have proved to be slower and vulnerable to variations. In this paper, we propose an approximate mixed-signal design for the distance computing core by noting the fact that a vast majority of the signal processing applications involving this operation are resilient to small approximations in the distance computation. The proposed mixed-signal design is able to interface with external digital CMOS logic and simultaneously exhibit fast operating speeds. Another important feature of the proposed design is that the computing core is able to compute two variants of the distance metric, namely the (i) Euclidean distance squared (L2(2 )norm) and (ii) Manhattan distance (L1 norm). The performance of the proposed design was evaluated on a standard K-means clustering algorithm on the "Iris flower dataset ". The results indicate a throughput of 6 ns per classification and & SIM;2.3x lower energy consumption in comparison to a synthesized digital CMOS design in commercial 45 nm CMOS technology.
更多查看译文
关键词
distance computation, K-means clustering, approximate computing
AI 理解论文
溯源树
样例
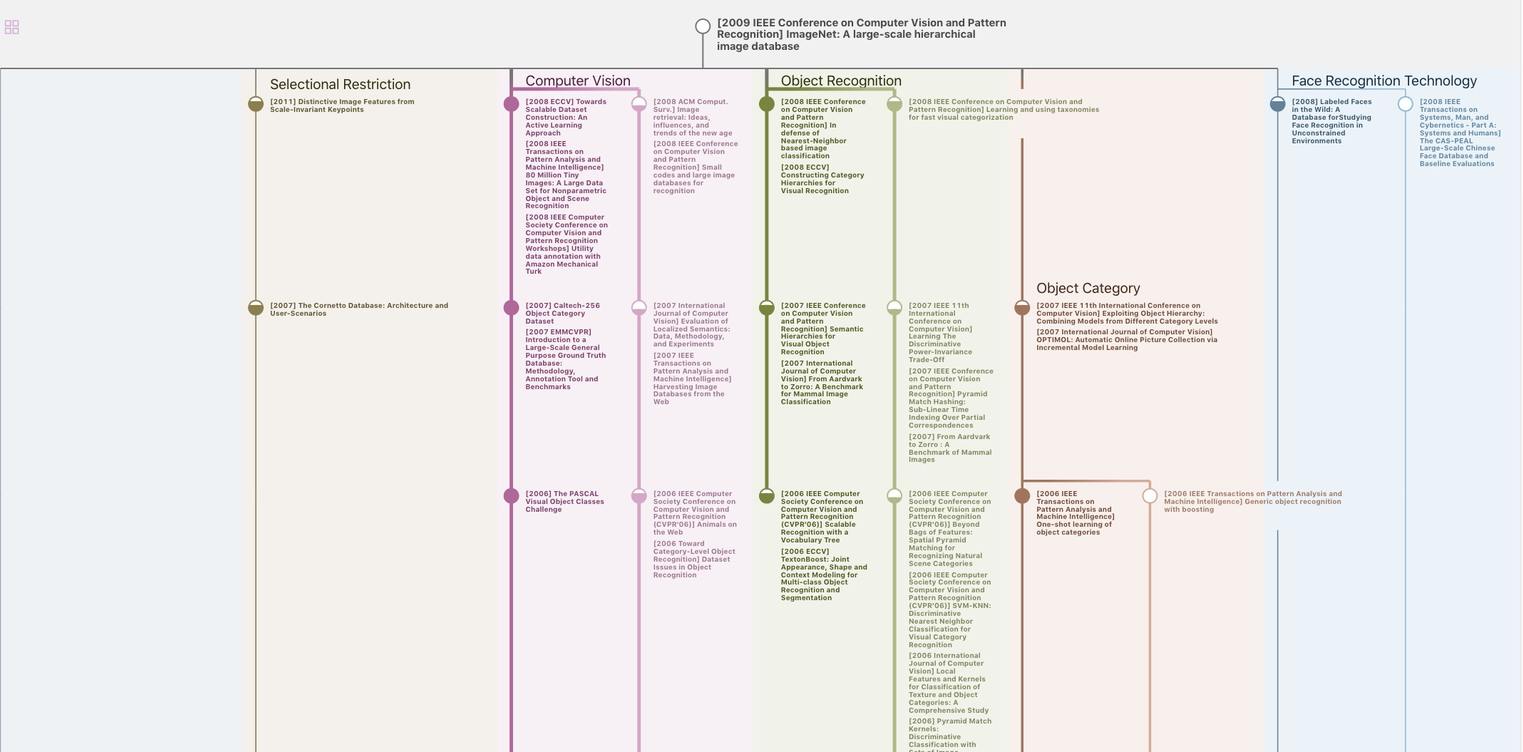
生成溯源树,研究论文发展脉络
Chat Paper
正在生成论文摘要