Neuronal Morphological Model-Driven Image Registration for Serial Electron Microscopy Sections
FRONTIERS IN HUMAN NEUROSCIENCE(2022)
摘要
Registration of a series of the two-dimensional electron microscope (EM) images of the brain tissue into volumetric form is an important technique that can be used for neuronal circuit reconstruction. However, complex appearance changes of neuronal morphology in adjacent sections bring difficulty in finding correct correspondences, making serial section neural image registration challenging. To solve this problem, we consider whether there are such stable "markers " in the neural images to alleviate registration difficulty. In this paper, we employ the spherical deformation model to simulate the local neuron structure and analyze the relationship between registration accuracy and neuronal structure shapes in two adjacent sections. The relevant analysis proves that regular circular structures in the section images are instrumental in seeking robust corresponding relationships. Then, we design a new serial section image registration framework driven by this neuronal morphological model, fully utilizing the characteristics of the anatomical structure of nerve tissue and obtaining more reasonable corresponding relationships. Specifically, we leverage a deep membrane segmentation network and neural morphological physical selection model to select the stable rounded regions in neural images. Then, we combine feature extraction and global optimization of correspondence position to obtain the deformation field of multiple images. Experiments on real and synthetic serial EM section neural image datasets have demonstrated that our proposed method could achieve more reasonable and reliable registration results, outperforming the state-of-the-art approaches in qualitative and quantitative analysis.
更多查看译文
关键词
neuronal structure, spherical deformation model, image registration, registration accuracy, serial section electron microscopy
AI 理解论文
溯源树
样例
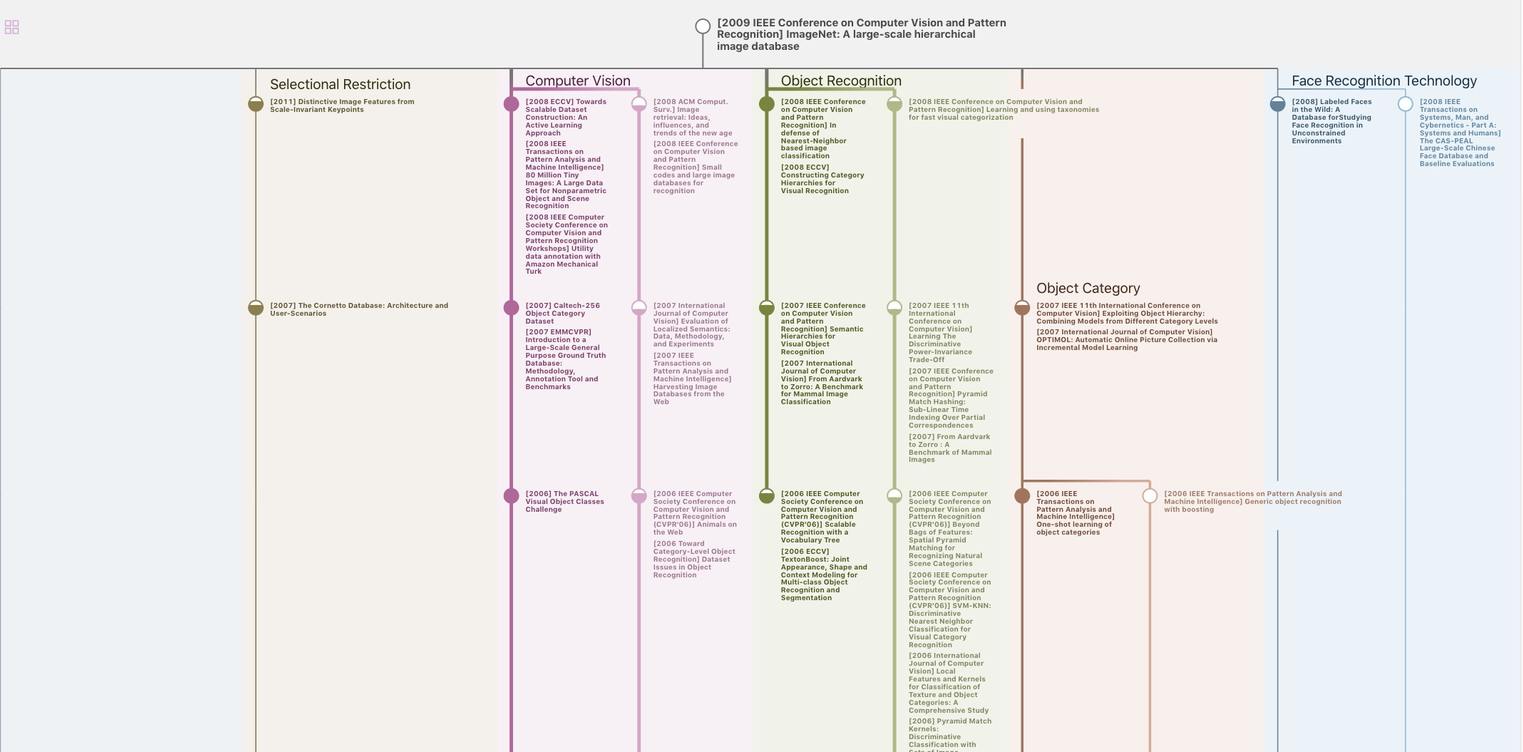
生成溯源树,研究论文发展脉络
Chat Paper
正在生成论文摘要