Leveraging clinical data across healthcare institutions for continual learning of predictive risk models
SCIENTIFIC REPORTS(2022)
摘要
The inherent flexibility of machine learning-based clinical predictive models to learn from episodes of patient care at a new institution (site-specific training) comes at the cost of performance degradation when applied to external patient cohorts. To exploit the full potential of cross-institutional clinical big data, machine learning systems must gain the ability to transfer their knowledge across institutional boundaries and learn from new episodes of patient care without forgetting previously learned patterns. In this work, we developed a privacy-preserving learning algorithm named WUPERR (Weight Uncertainty Propagation and Episodic Representation Replay) and validated the algorithm in the context of early prediction of sepsis using data from over 104,000 patients across four distinct healthcare systems. We tested the hypothesis, that the proposed continual learning algorithm can maintain higher predictive performance than competing methods on previous cohorts once it has been trained on a new patient cohort. In the sepsis prediction task, after incremental training of a deep learning model across four hospital systems (namely hospitals H-A, H-B, H-C, and H-D), WUPERR maintained the highest positive predictive value across the first three hospitals compared to a baseline transfer learning approach (H-A: 39.27% vs. 31.27% , H-B: 25.34% vs. 22.34% , H-C: 30.33 % vs. 28.33% ). The proposed approach has the potential to construct more generalizable models that can learn from cross-institutional clinical big data in a privacy-preserving manner.
更多查看译文
关键词
Computer science,Infectious diseases,Science,Humanities and Social Sciences,multidisciplinary
AI 理解论文
溯源树
样例
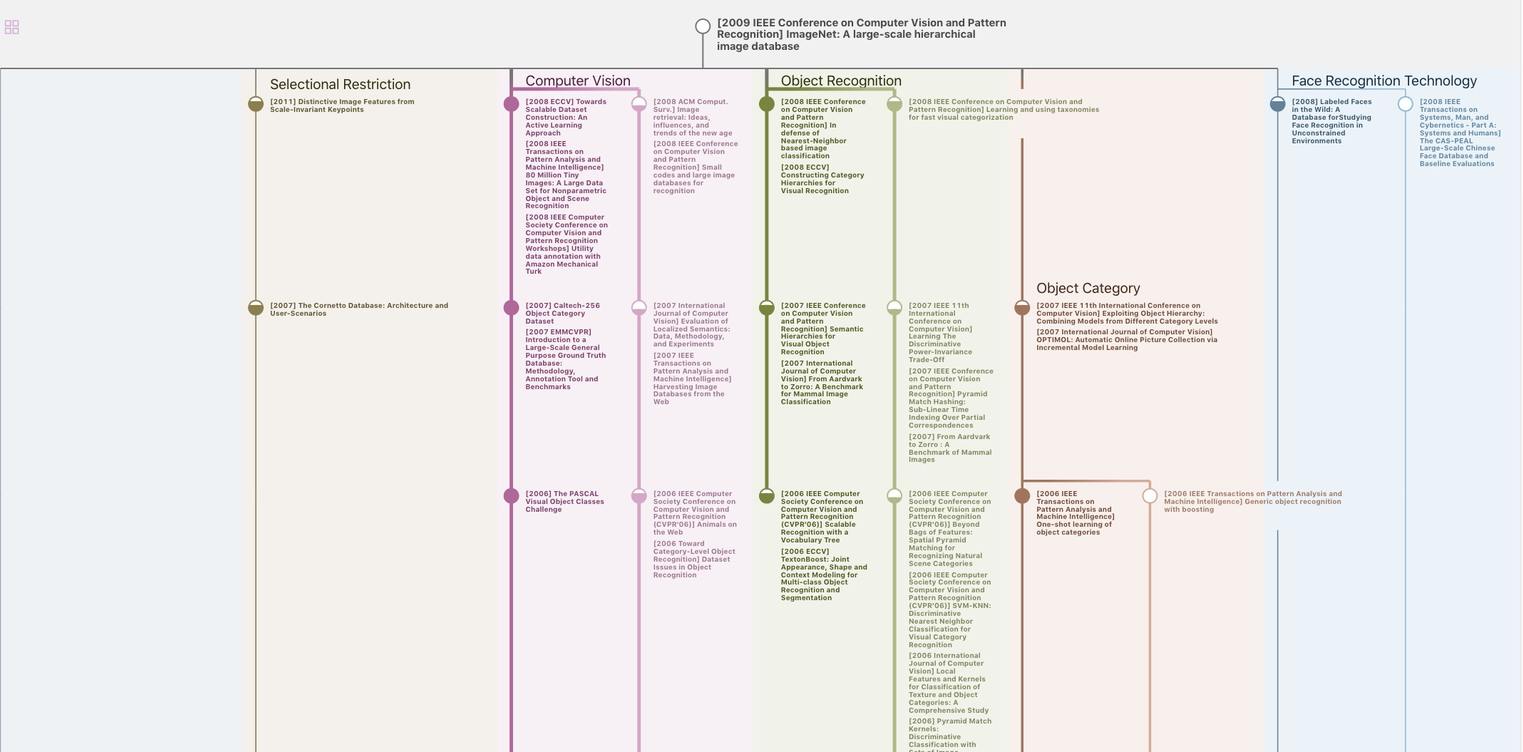
生成溯源树,研究论文发展脉络
Chat Paper
正在生成论文摘要