Stop filtering: Multi-view attribute-enhanced dialogue learning
arxiv(2023)
摘要
There is a growing interest in improving the conversational ability of models by filtering dialogue corpora. Previous filtering strategies rely on a scoring method to assess and discard samples from one perspective, enabling the model to enhance the corresponding dialogue attributes (e.g., consistency). However, the discarded samples may achieve high scores in other perspectives and can also provide regularization effects on the model learning. In this work, we propose a multi-view attribute-enhanced dialogue learning framework that can capture the attribute-related features steadily and comprehensively. Instead of filtering the raw dataset, our framework introduces adapters to learn knowledge from the attribute-related sub-sets after pre-training the model on the full dataset. Considering the variety of dialogue attributes, we further design a multi-view enhancement mechanism, including multi-view selection and inter-view fusion. It groups the high-quality samples from multiple perspectives, respectively, and enhances different attributes of responses with the corresponding sub-sets and adapters, keeping knowledge independent and allowing flexible integration. Empirical results and analysis show that our framework outperforms the state-of-the-art data filtering methods significantly in terms of enhancing dialogue attributes and fusing view-specific knowledge.
更多查看译文
关键词
Open-domain dialogue generation,Data filtering,Dialogue attributes
AI 理解论文
溯源树
样例
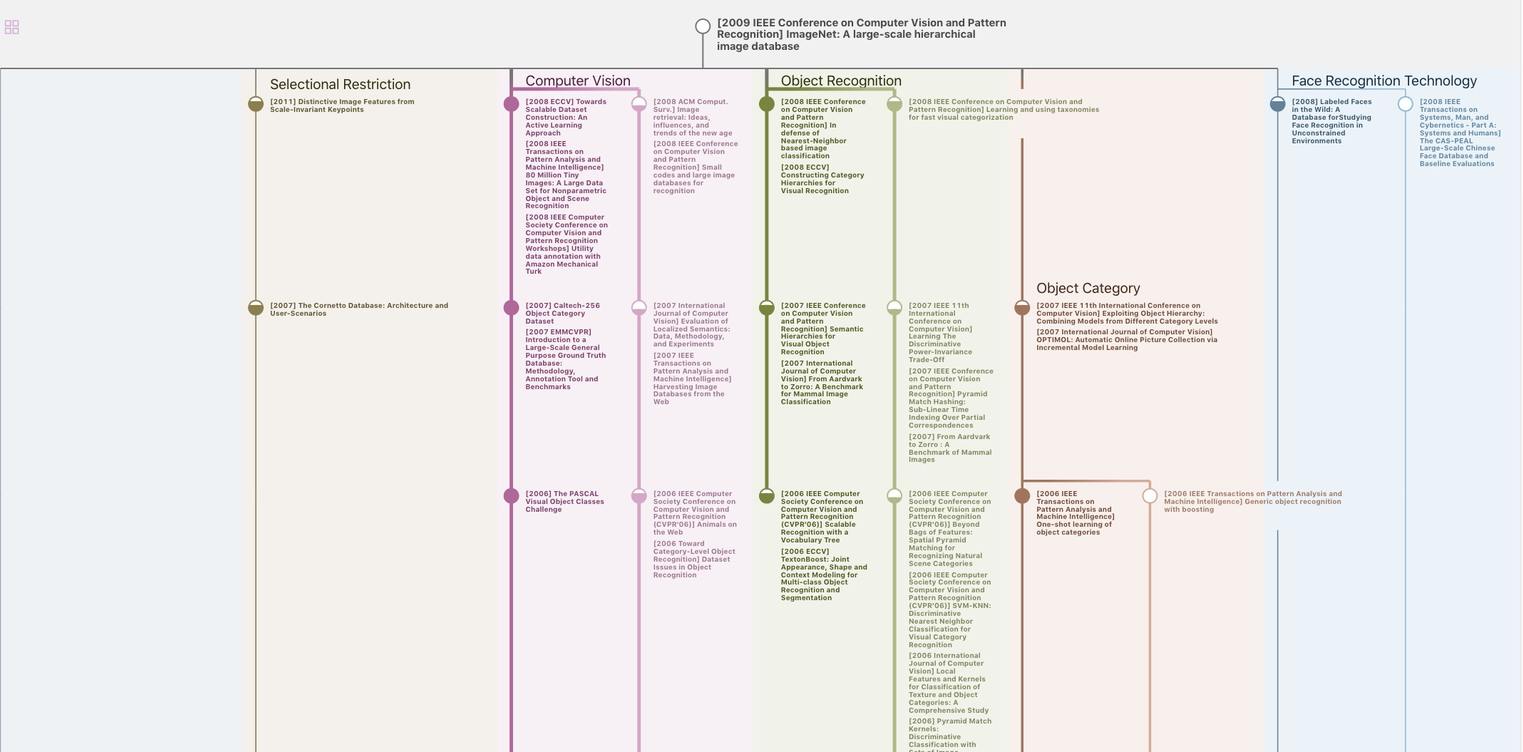
生成溯源树,研究论文发展脉络
Chat Paper
正在生成论文摘要