MolMiner: You Only Look Once for Chemical Structure Recognition.
J. Chem. Inf. Model.(2022)
摘要
Molecular structures are commonly depicted in 2D printed forms in scientific documents such as journal papers and patents. However, these 2D depictions are not machine readable. Due to a backlog of decades and an increasing amount of printed literatures, there is a high demand for translating printed depictions into machine-readable formats, which is known as Optical Chemical Structure Recognition (OCSR). Most OCSR systems developed over the last three decades use a rule-based approach, which vectorizes the depiction based on the interpretation of vectors and nodes as bonds and atoms. Here, we present a practical software called MolMiner, which is primarily built using deep neural networks originally developed for semantic segmentation and object detection to recognize atom and bond elements from documents. These recognized elements can be easily connected as a molecular graph with a distance-based construction algorithm. MolMiner gave state-of-the-art performance on four benchmark data sets and a self-collected external data set from scientific papers. As MolMiner performed similarly well in real-world OCSR tasks with a user-friendly interface, it is a useful and valuable tool for daily applications. The free download links of Mac and Windows versions are available at https://github.com/iipharma/pharmamind-molminer.
更多查看译文
关键词
molminer,chemical,recognition,structure
AI 理解论文
溯源树
样例
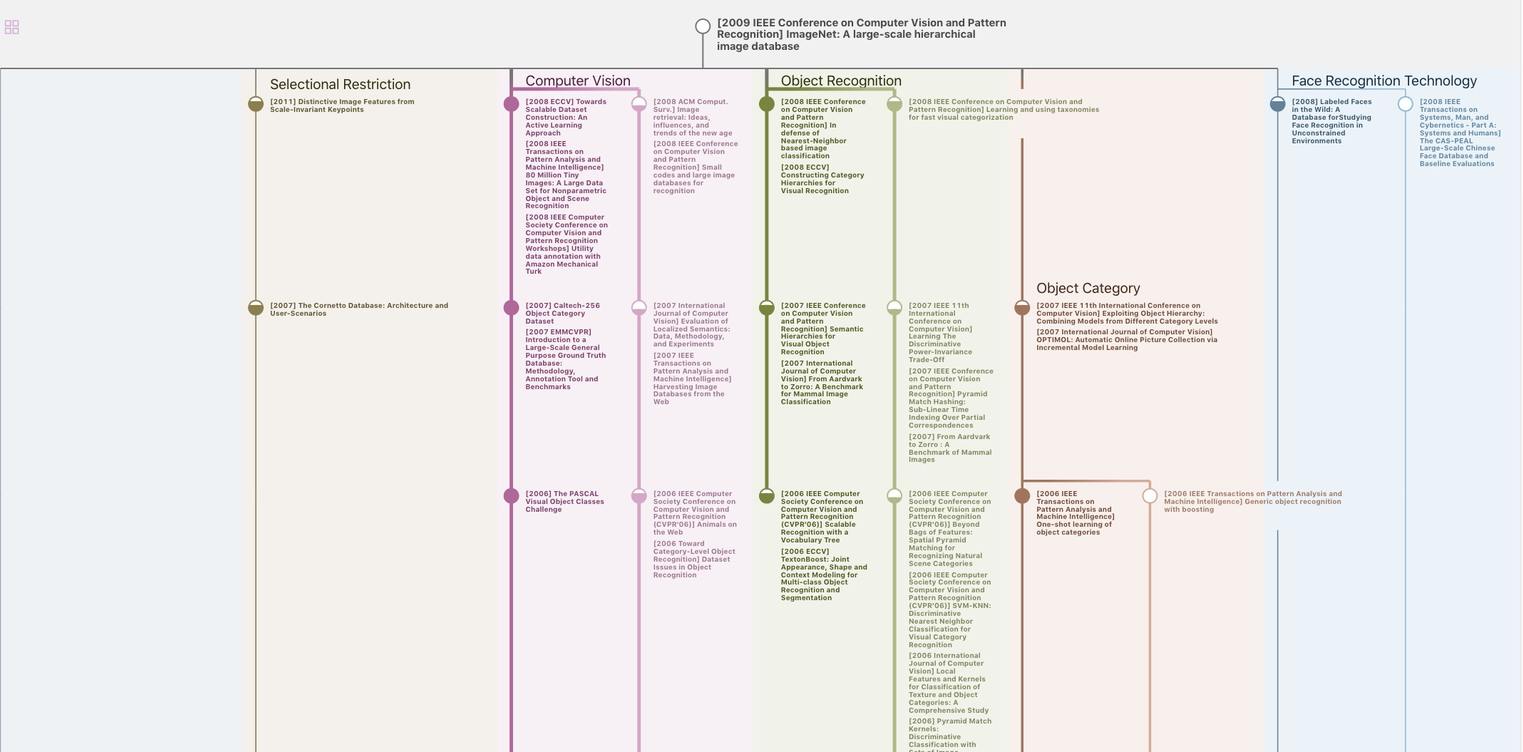
生成溯源树,研究论文发展脉络
Chat Paper
正在生成论文摘要