Data-aided Active User Detection with a User Activity Extraction Network for Grant-free SCMA Systems
GLOBECOM (Workshops)(2022)
摘要
In grant-free sparse code multiple access (GF-SCMA) system, active user detection (AUD) is a major performance bottleneck as it involves complex combinatorial problem, which makes joint design of contention resources for users and AUD at the receiver a crucial but a challenging problem. To this end, we consider an autoencoder (AE)-based joint optimization of both preamble generation networks (PGNs) in the encoder side and data-aided AUD in the decoder side. The core architecture of the proposed AE is a novel user activity extraction network (UAEN) in the decoder that extracts a priori user activity information from the SCMA codeword data for the data-aided AUD. An end-to-end training of the proposed AE enables joint optimization of the contention resources, i.e., preamble sequences, each associated with one of the codebooks, and extraction of user activity information from both preamble and SCMA-based data transmission. Furthermore, we propose a self-supervised pretraining scheme for the UAEN prior to the end-to-end training, ensuring the convergence of the UAEN which lies deep inside the AE network. Simulation results demonstrated that the proposed AUD scheme achieved a gain of 3 to 5dB at a target activity detection error rate of $10^{-3}$ compared to the state-of-the-art DL-based AUD schemes.
更多查看译文
关键词
Grant-free,sparse code multiple access,active user detection,deep-learning,autoencoder,extraction network
AI 理解论文
溯源树
样例
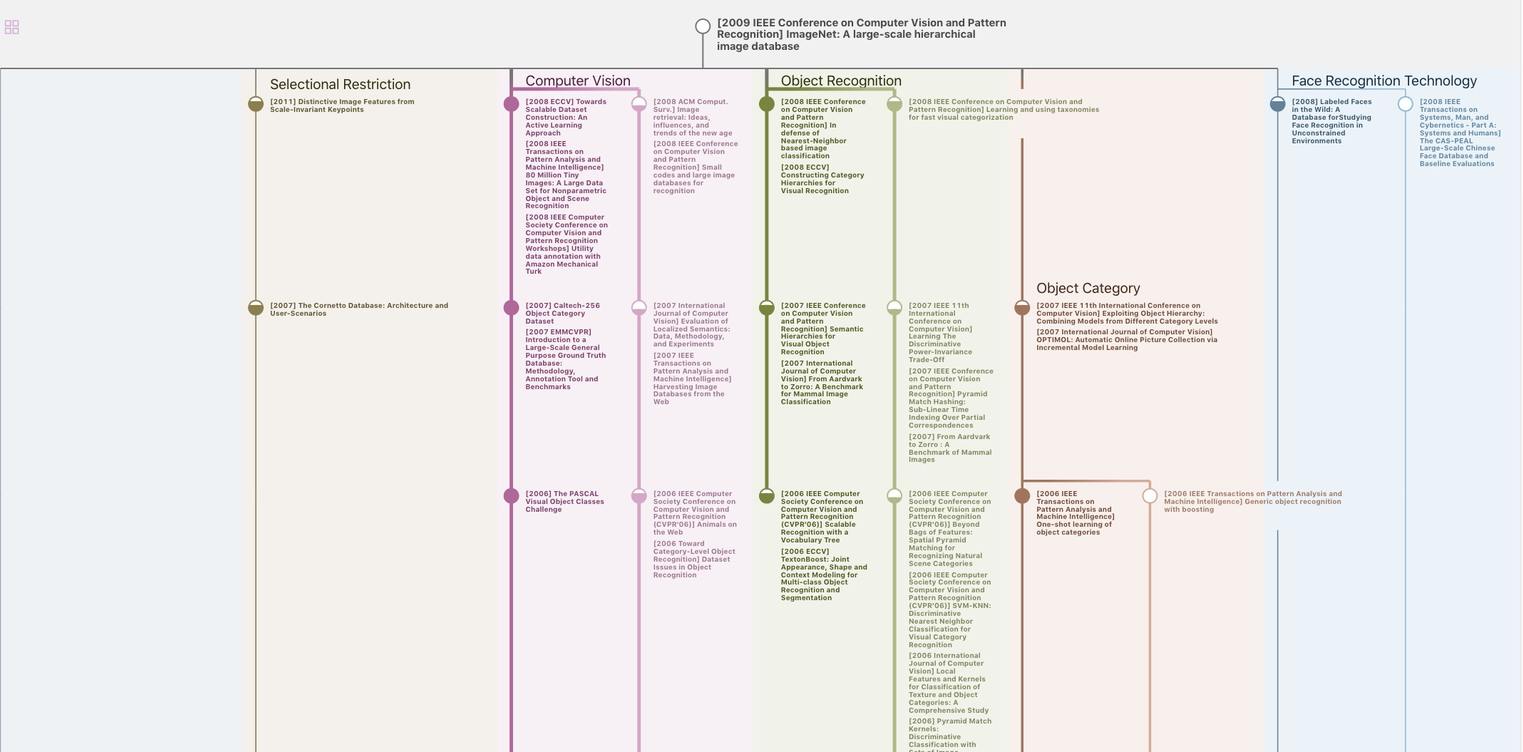
生成溯源树,研究论文发展脉络
Chat Paper
正在生成论文摘要