Swapping Semantic Contents for Mixing Images.
ICPR(2022)
摘要
Deep architecture have proven capable of solving many tasks provided a sufficient amount of labeled data. In fact, the amount of available labeled data has become the principal bottleneck in low label settings such as Semi-Supervised Learning. Mixing Data Augmentations do not typically yield new labeled samples, as indiscriminately mixing contents creates between-class samples. In this work, we introduce the SciMix framework that can learn to generator to embed a semantic style code into image backgrounds, we obtain new mixing scheme for data augmentation. We then demonstrate that SciMix yields novel mixed samples that inherit many characteristics from their non-semantic parents. Afterwards, we verify those samples can be used to improve the performance semi-supervised frameworks like Mean Teacher or Fixmatch, and even fully supervised learning on a small labeled dataset.
更多查看译文
关键词
available labeled data,data augmentation,deep architecture,global semantic content,image backgrounds,labeled dataset,labeled samples,mixed samples,mixing contents,mixing scheme,nonsemantic parents,principal bottleneck,SciMix framework,semantic contents,semantic style code,semisupervised framework performance improvement,StyleGan generator,supervised learning
AI 理解论文
溯源树
样例
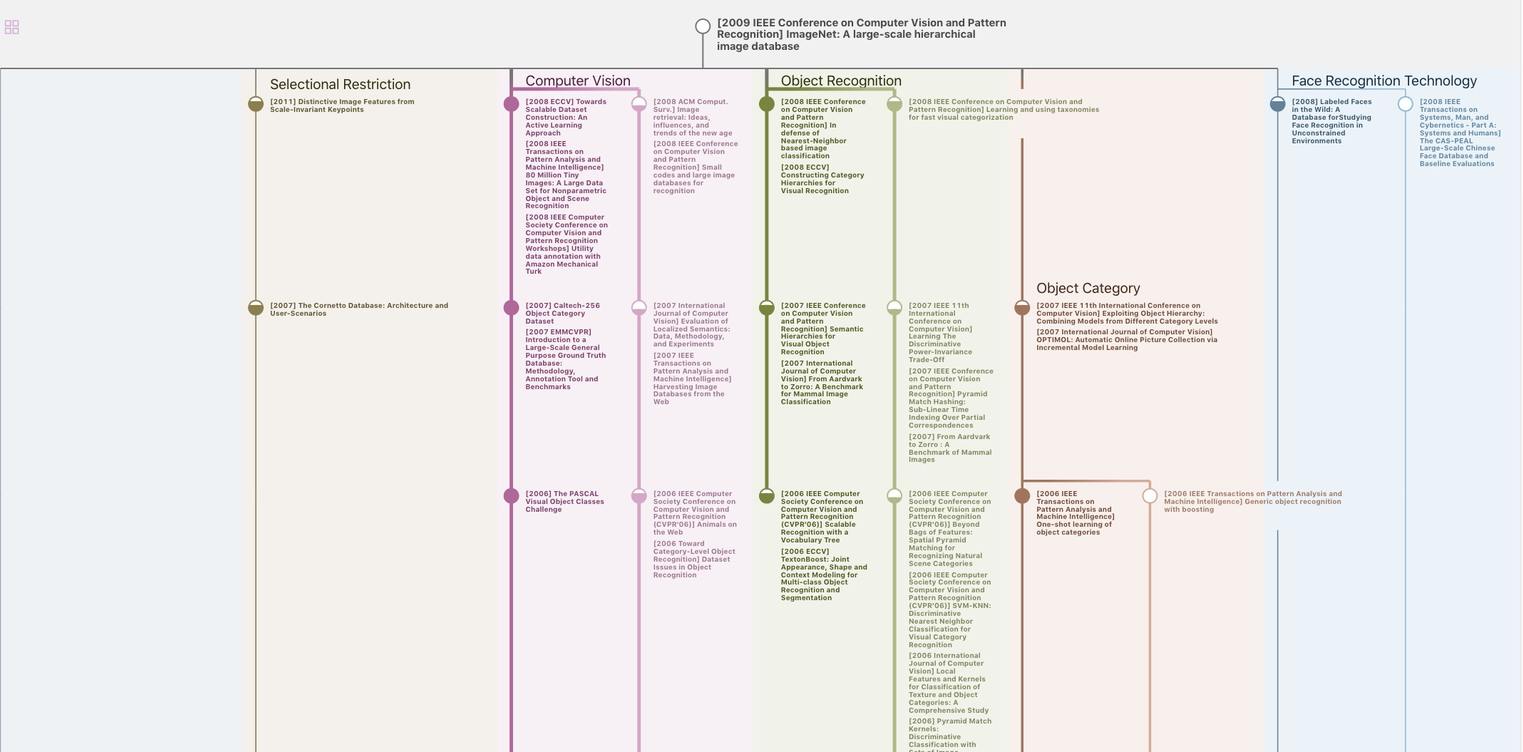
生成溯源树,研究论文发展脉络
Chat Paper
正在生成论文摘要