Topology-Aware Graph Neural Networks for Learning Feasible and Adaptive AC-OPF Solutions
IEEE TRANSACTIONS ON POWER SYSTEMS(2023)
摘要
Solving the optimal power flow (OPF) problem is a fundamental task to ensure the system efficiency and reliability in real-time electricity grid operations. We develop a new topology-informed graph neural network (GNN) approach for predicting the optimal solutions of real-time ac-OPF problem. To incorporate grid topology to the NN model, the proposed GNN-for-OPF framework innovatively exploits the locality property of locational marginal prices and voltage magnitude. Furthermore, we develop a physics-aware (ac-)flow feasibility regularization approach for general OPF learning. The advantages of our proposed designs include reduced model complexity, improved generalizability and feasibility guarantees. By providing the analytical understanding on the graph subspace stability under grid topology contingency, we show the proposed GNN can quickly adapt to varying grid topology by an efficient re-training strategy. Numerical tests on various test systems of different sizes have validated the prediction accuracy, improved flow feasibility, and topology adaptivity capability of our proposed GNN-based learning framework.
更多查看译文
关键词
Topology,Predictive models,Voltage,Load flow,Transmission line matrix methods,Network topology,Task analysis,Optimal power flow,graph neural network,feasibility regularization,topology adaptivity
AI 理解论文
溯源树
样例
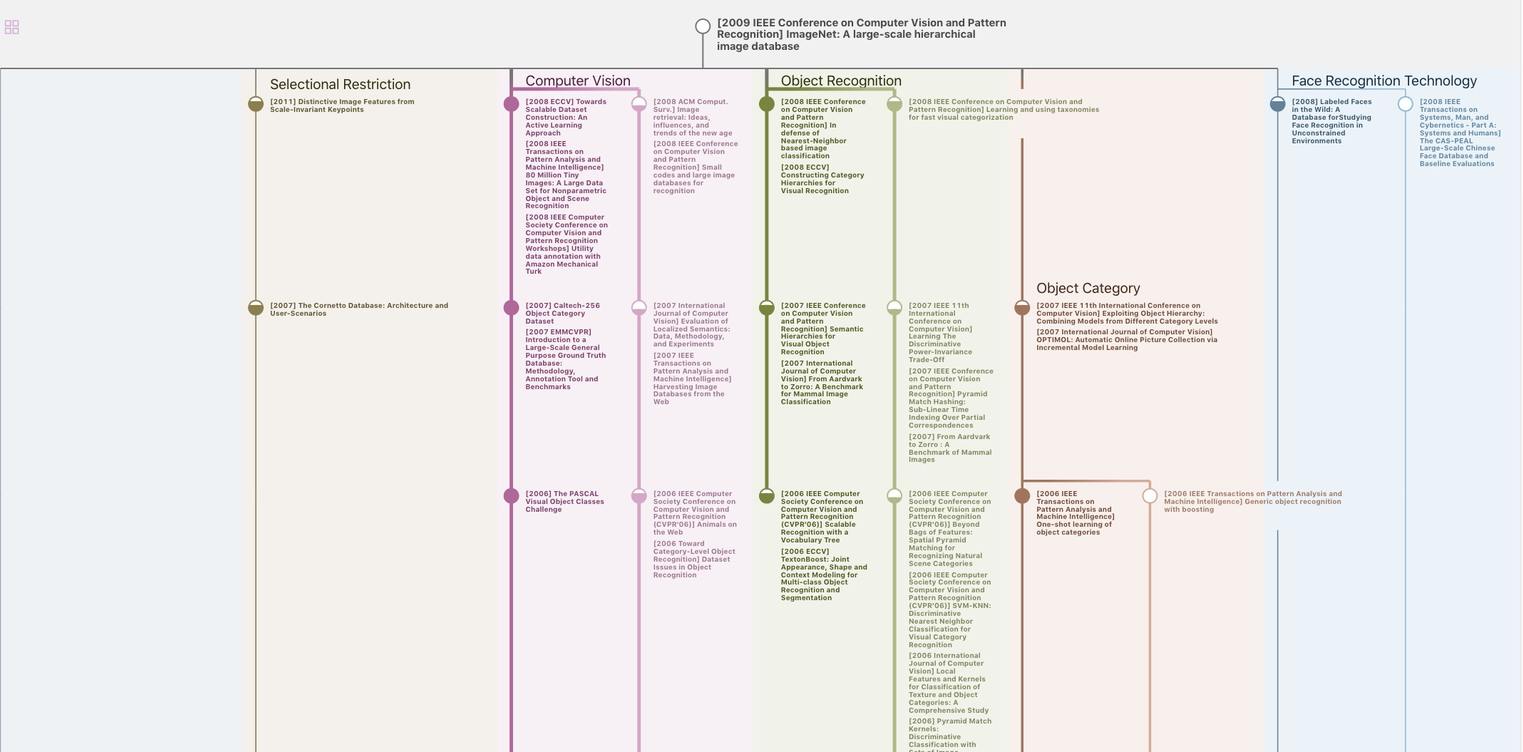
生成溯源树,研究论文发展脉络
Chat Paper
正在生成论文摘要