Transition-based semantic role labeling with pointer networks
Knowledge-Based Systems(2023)
摘要
Semantic role labeling (SRL) focuses on recognizing the predicate-argument structure of a sentence and plays a critical role in many natural language processing tasks such as machine translation and question answering. Practically all available methods do not perform full SRL, since they rely on pre -identified predicates, and most of them follow a pipeline strategy, using specific models for undertaking one or several SRL subtasks. In addition, previous approaches have a strong dependence on syntactic information to achieve state-of-the-art performance, despite being syntactic trees equally hard to produce. These simplifications and requirements make the majority of SRL systems impractical for real -world applications. In this article, we propose the first transition-based SRL approach that is capable of completely processing an input sentence in a single left-to-right pass, with neither leveraging syntactic information nor resorting to additional modules. Thanks to our implementation based on Pointer Networks, full SRL can be accurately and efficiently done in O(n2), achieving the best performance to date on the majority of languages from the CoNLL-2009 shared task.(c) 2022 Elsevier B.V. All rights reserved.
更多查看译文
关键词
Natural language processing,Computational linguistics,Semantic role labeling,Neural network,Deep learning
AI 理解论文
溯源树
样例
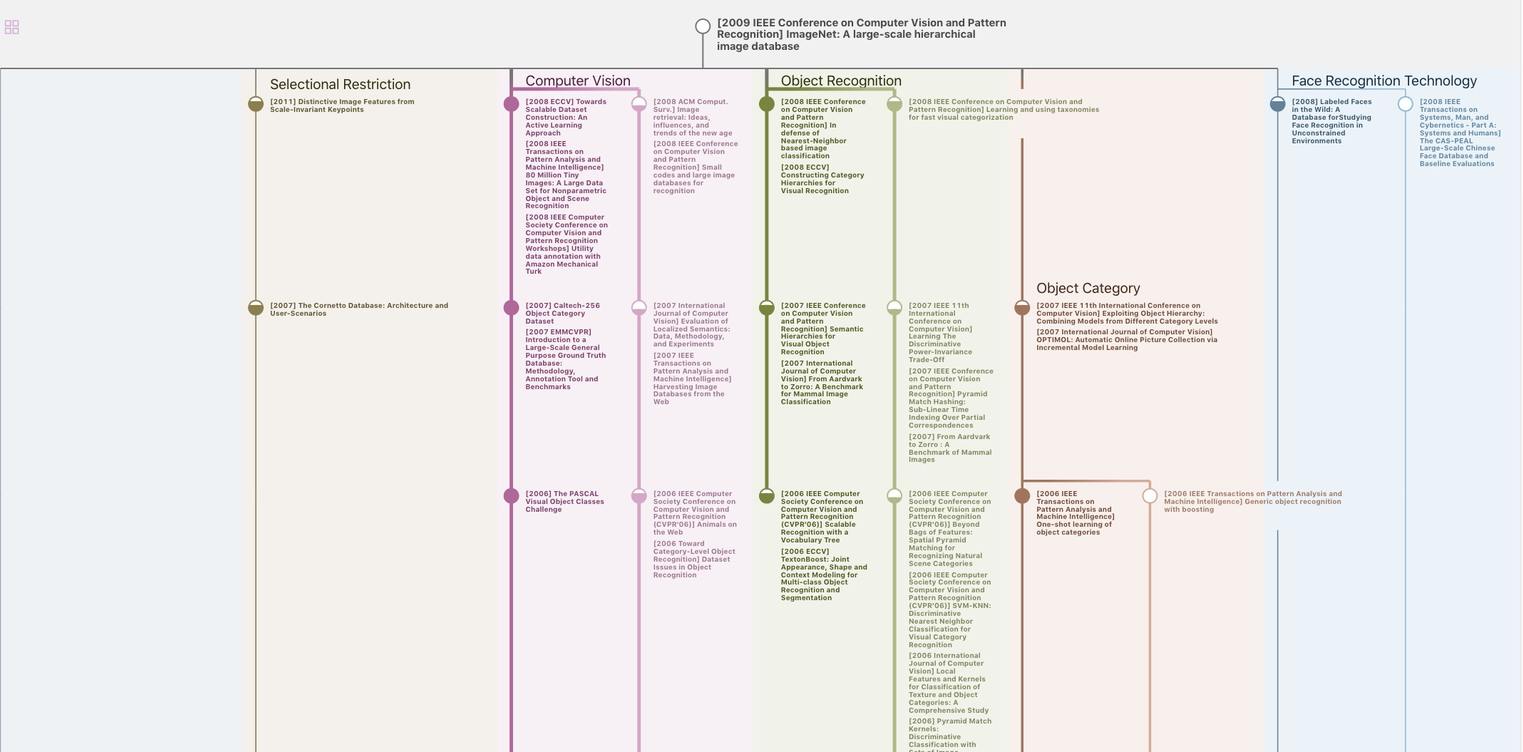
生成溯源树,研究论文发展脉络
Chat Paper
正在生成论文摘要