Premise-based Multimodal Reasoning: Conditional Inference on Joint Textual and Visual Clues
PROCEEDINGS OF THE 60TH ANNUAL MEETING OF THE ASSOCIATION FOR COMPUTATIONAL LINGUISTICS (ACL 2022), VOL 1: (LONG PAPERS)(2022)
摘要
It is a common practice for recent works in vision language cross-modal reasoning to adopt a binary or multi-choice classification formulation taking as input a set of source image(s) and textual query. In this work, we take a sober look at such an "unconditional" formulation in the sense that no prior knowledge is specified with respect to the source image(s). Inspired by the designs of both visual commonsense reasoning and natural language inference tasks, we propose a new task termed "Premise-based Multi-modal Reasoning" (PMR) where a textual premise is the background presumption on each source image. The PMR dataset contains 15,360 manually annotated samples which are created by a multi-phase crowd-sourcing process. With selected high-quality movie screenshots and human-curated premise templates from 6 pre-defined categories, we ask crowd-source workers to write one true hypothesis and three distractors (4 choices) given the premise and image through a cross-check procedure. Besides, we generate adversarial samples to alleviate the annotation artifacts and double the size of PMR. We benchmark various state-of-the-art (pretrained) multi-modal inference models on PMR and conduct comprehensive experimental analyses to showcase the utility of our dataset.
更多查看译文
关键词
multimodal reasoning,conditional inference,joint textual,premise-based
AI 理解论文
溯源树
样例
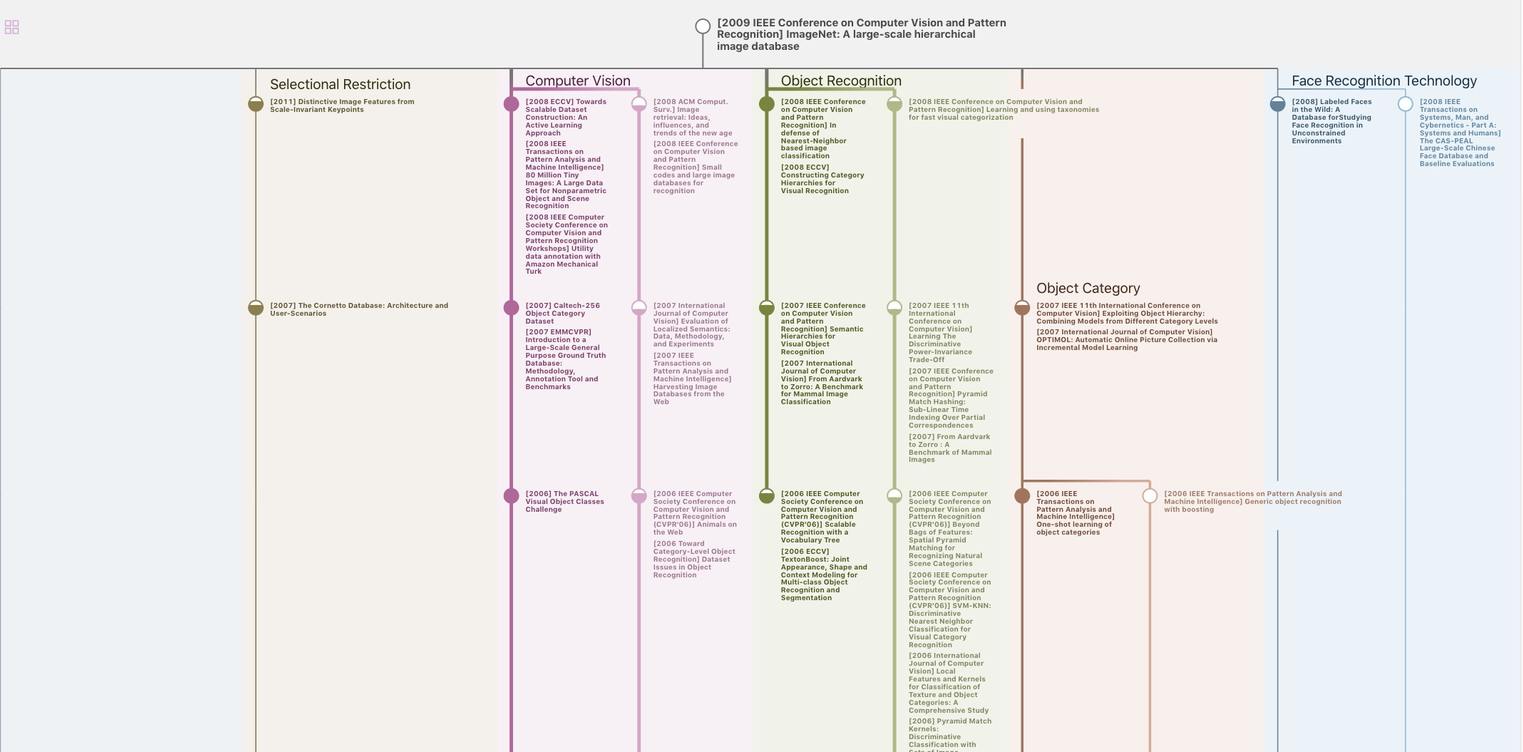
生成溯源树,研究论文发展脉络
Chat Paper
正在生成论文摘要