Upstream Mitigation Is Not All You Need: Testing the Bias Transfer Hypothesis in Pre-Trained Language Models
PROCEEDINGS OF THE 60TH ANNUAL MEETING OF THE ASSOCIATION FOR COMPUTATIONAL LINGUISTICS (ACL 2022), VOL 1: (LONG PAPERS)(2022)
摘要
A few large, homogenous, pre-trained models undergird many machine learning systems - and often, these models contain harmful stereotypes learned from the internet. We investigate the bias transfer hypothesis: the theory that social biases (such as stereotypes) internalized by large language models during pre-training transfer into harmful task-specific behavior after fine-tuning. For two classification tasks, we find that reducing intrinsic bias with controlled interventions before fine-tuning does little to mitigate the classifier's discriminatory behavior after fine-tuning. Regression analysis suggests that downstream disparities are better explained by biases in the fine-tuning dataset. Still, pre-training plays a role: simple alterations to co-occurrence rates in the fine-tuning dataset are ineffective when the model has been pre-trained. Our results encourage practitioners to focus more on dataset quality and context-specific harms.
更多查看译文
关键词
bias transfer hypothesis,language,mitigation,pre-trained
AI 理解论文
溯源树
样例
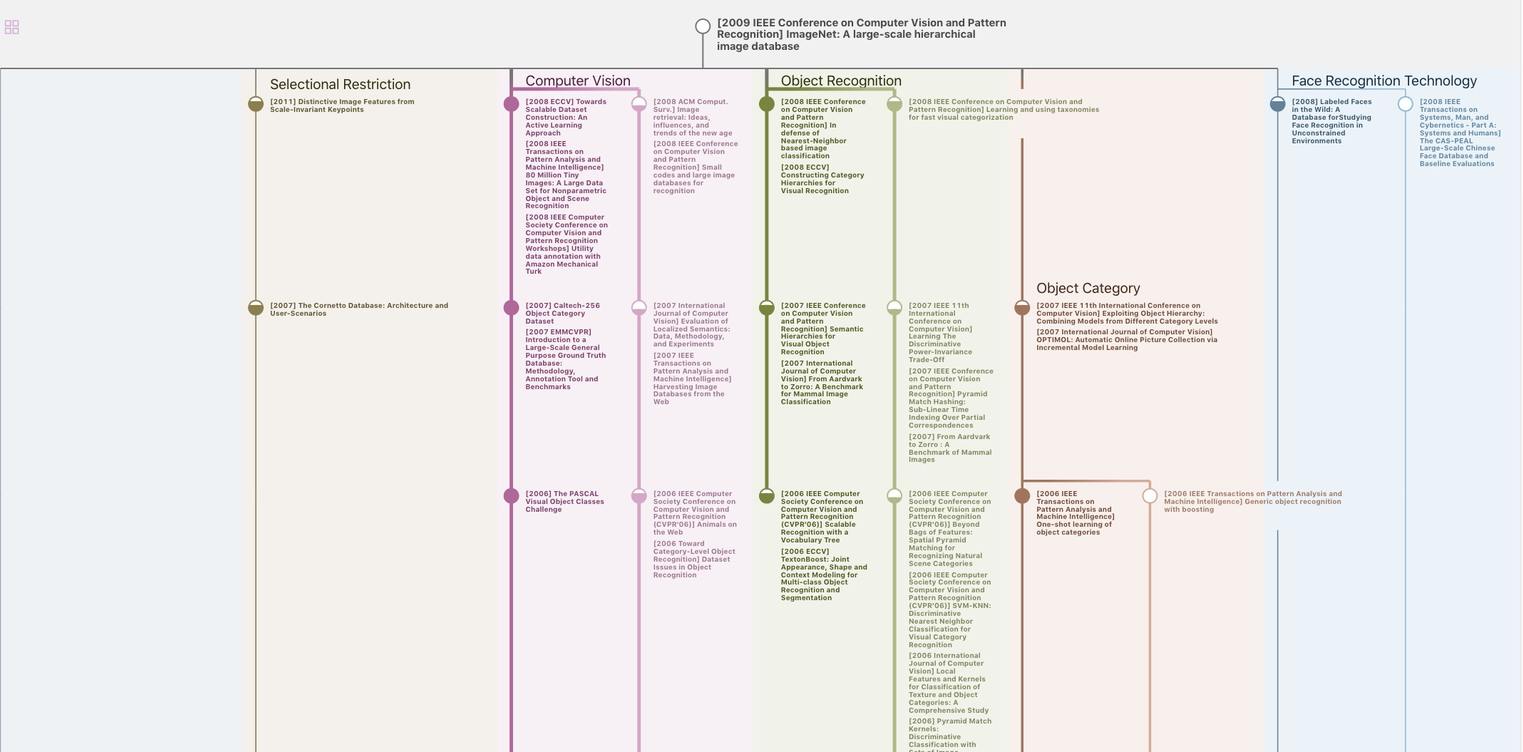
生成溯源树,研究论文发展脉络
Chat Paper
正在生成论文摘要