Predicting scalar diversity with context-driven uncertainty over alternatives
PROCEEDINGS OF THE WORKSHOP ON COGNITIVE MODELING AND COMPUTATIONAL LINGUISTICS (CMCL 2022)(2022)
摘要
Scalar implicature (SI) arises when a speaker uses an expression (e.g., some) that is semantically compatible with a logically stronger alternative on the same scale (e.g., all), leading the listener to infer that they did not intend to convey the stronger meaning. Prior work has demonstrated that SI rates are highly variable across scales, raising the question of what factors determine the SI strength for a particular scale. Here, we test the hypothesis that SI rates depend on the listener's confidence in the underlying scale, which we operationalize as uncertainty over the distribution of possible alternatives conditioned on the context. We use a T5 model fine-tuned on a text infilling task to estimate this distribution. We find that scale uncertainty predicts human SI rates, measured as entropy over the sampled alternatives and over latent classes among alternatives in sentence embedding space. Furthermore, we do not find a significant effect of the surprisal of the strong scalemate. Our results suggest that pragmatic inferences depend on listeners' context-driven uncertainty over alternatives.
更多查看译文
AI 理解论文
溯源树
样例
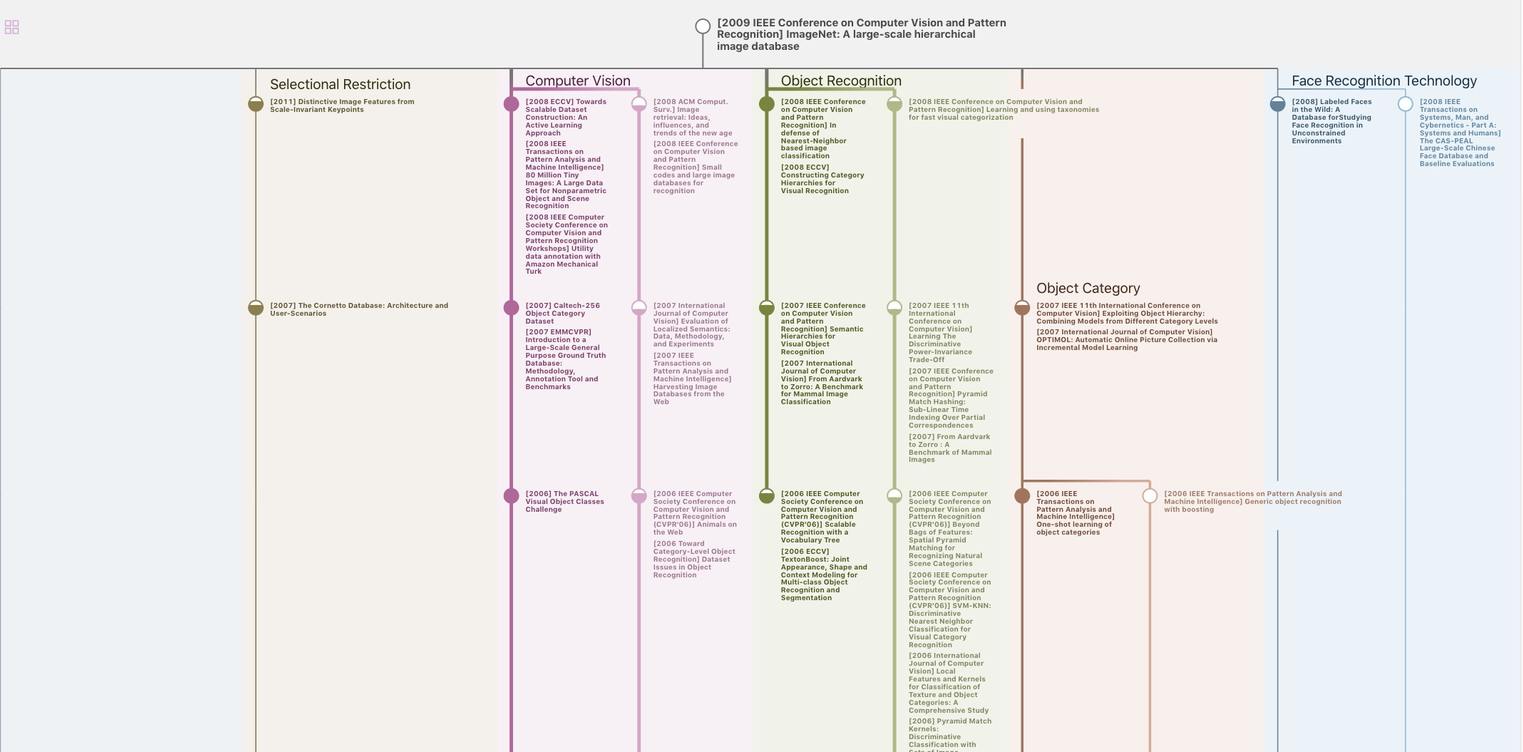
生成溯源树,研究论文发展脉络
Chat Paper
正在生成论文摘要