Hierarchical Ensemble Kalman Methods with Sparsity-Promoting Generalized Gamma Hyperpriors
arxiv(2022)
摘要
This paper introduces a computational framework to incorporate flexible regularization techniques in ensemble Kalman methods for nonlinear inverse problems. The proposed methodology approximates the maximum a posteriori (MAP) estimate of a hierarchical Bayesian model characterized by a conditionally Gaussian prior and generalized gamma hyperpriors. Suitable choices of hyperparameters yield sparsity-promoting regularization. We propose an iterative algorithm for MAP estimation, which alternates between updating the unknown with an ensemble Kalman method and updating the hyperparameters in the regularization to promote sparsity. The effectiveness of our methodology is demonstrated in several computed examples, including compressed sensing and subsurface flow inverse problems.
更多查看译文
关键词
Ensemble Kalman methods,sparsity-promoting regularization,itera-tive alternating scheme,hierarchical inverse problems
AI 理解论文
溯源树
样例
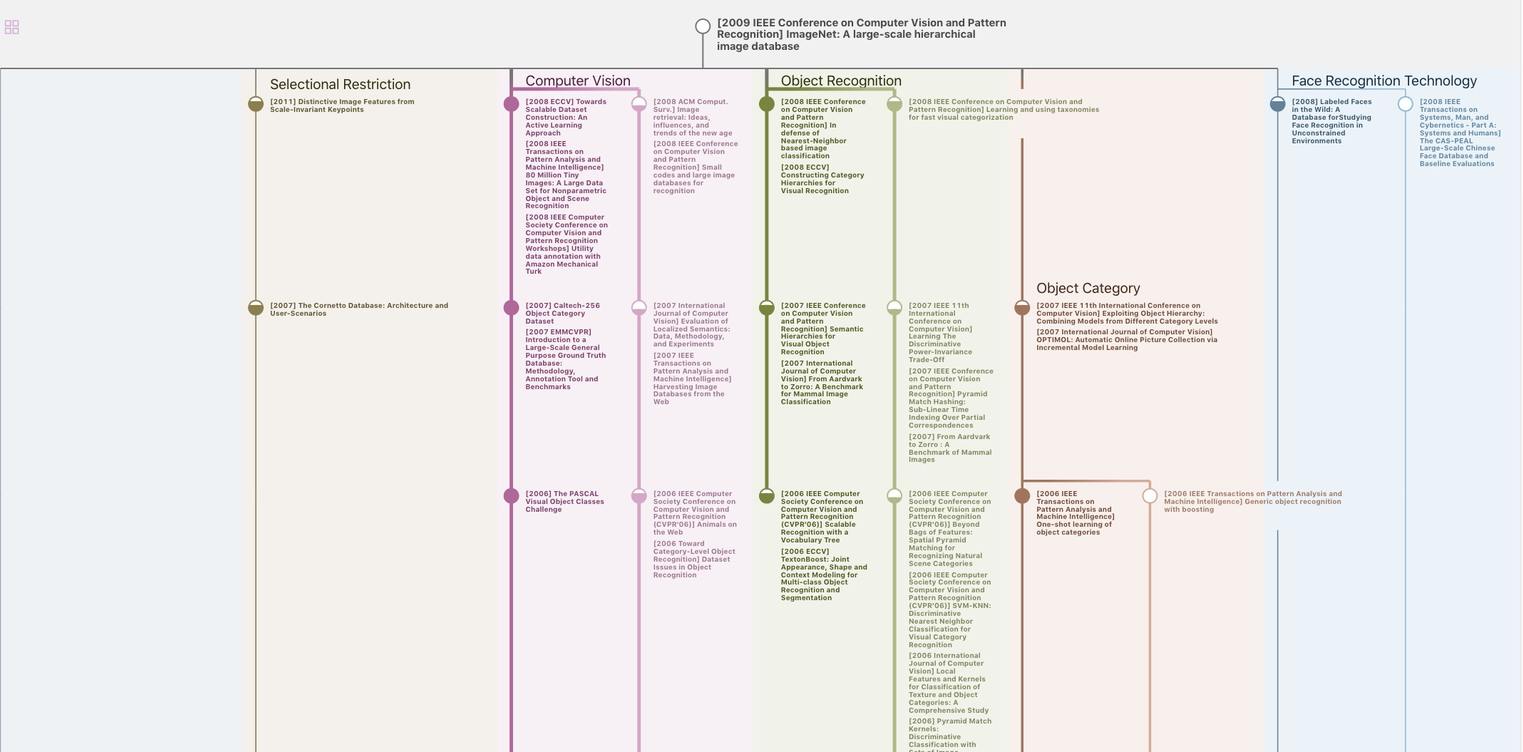
生成溯源树,研究论文发展脉络
Chat Paper
正在生成论文摘要