3-D Gravity and Magnetic Joint Inversion Based on Deep Learning Combined With Measurement Data Constraint
IEEE TRANSACTIONS ON GEOSCIENCE AND REMOTE SENSING(2024)
摘要
The joint inversion of gravity and magnetic data can reduce the nonuniqueness problem of potential field data inversion. We propose a gravity and magnetic joint inversion method based on deep learning (DL) combined with measurement data constraint. The framework obtains the gravity and magnetic dataset required for network training by randomly generating the underground structural consistency model and then inputs the dataset into the network for training. Moreover, we add constraints to the measurement data in the training of the network, that is, fitting the data anomalies obtained by the inversion model through forward calculation with the real anomalies, which makes the network more consistent with geophysical theory. In the test phase, the trained network can obtain the inversion results rapidly, and the inversion results of the testing dataset show that this method can obtain better results when applied to the joint inversion of gravity and magnetic fields than the conventional regularized inversion and cross-gradient joint inversion methods. In addition, our method can also distinguish the anomaly conditions in the case of structural inconsistency. Furthermore, we apply this method to actual gravity and magnetic data of Gonghe Basin, Qinghai Province, China, and predict the distribution of dry hot rock related to geothermal resources.
更多查看译文
关键词
Deep learning (DL),geothermal resources,gravity and magnetic data,joint inversion,U-Net
AI 理解论文
溯源树
样例
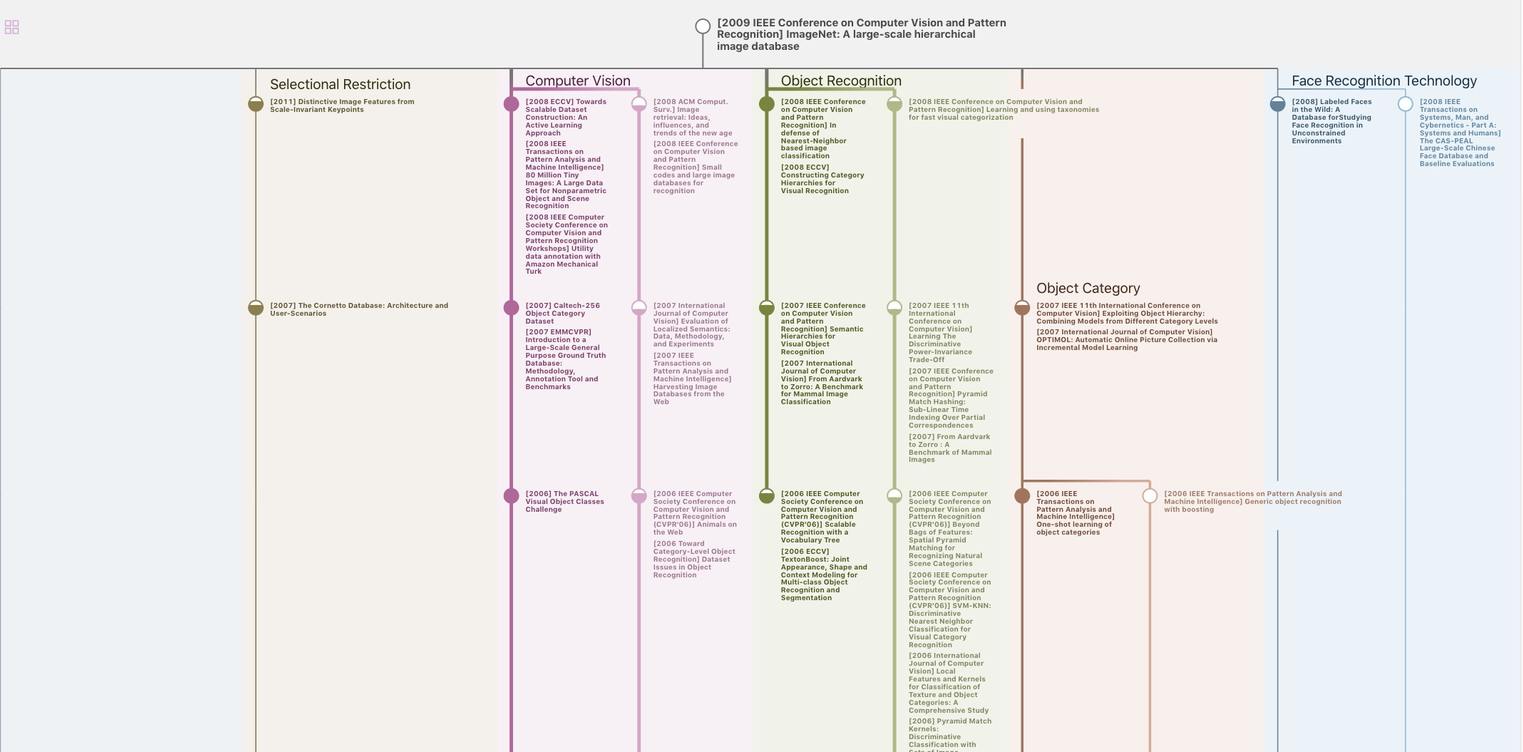
生成溯源树,研究论文发展脉络
Chat Paper
正在生成论文摘要