Towards Efficient Annotations for a Human-AI Collaborative, Clinical Decision Support System: A Case Study on Physical Stroke Rehabilitation Assessment
Intelligent User Interfaces(2022)
摘要
ABSTRACT Artificial intelligence (AI) and machine learning (ML) algorithms are increasingly being explored to support various decision-making tasks in health (e.g. rehabilitation assessment). However, the development of such AI/ML-based decision support systems is challenging due to the expensive process to collect an annotated dataset. In this paper, we describe the development process of a human-AI collaborative, clinical decision support system that augments an ML model with a rule-based (RB) model from domain experts. We conducted its empirical evaluation in the context of assessing physical stroke rehabilitation with the dataset of three exercises from 15 post-stroke survivors and therapists. Our results bring new insights on the efficient development and annotations of a decision support system: when an annotated dataset is not available initially, the RB model can be used to assess post-stroke survivor’s quality of motion and identify samples with low confidence scores to support efficient annotations for training an ML model. Specifically, our system requires only 22 - 33% of annotations from therapists to train an ML model that achieves equally good performance with an ML model with all annotations from a therapist. Our work discusses the values of a human-AI collaborative approach for effectively collecting an annotated dataset and supporting a complex decision-making task.
更多查看译文
关键词
Human Centered AI,Human-AI Collaboration,Human-In-the-Loop Systems,Clinical Decision Support Systems,Physical Stroke Rehabilitation Assessment
AI 理解论文
溯源树
样例
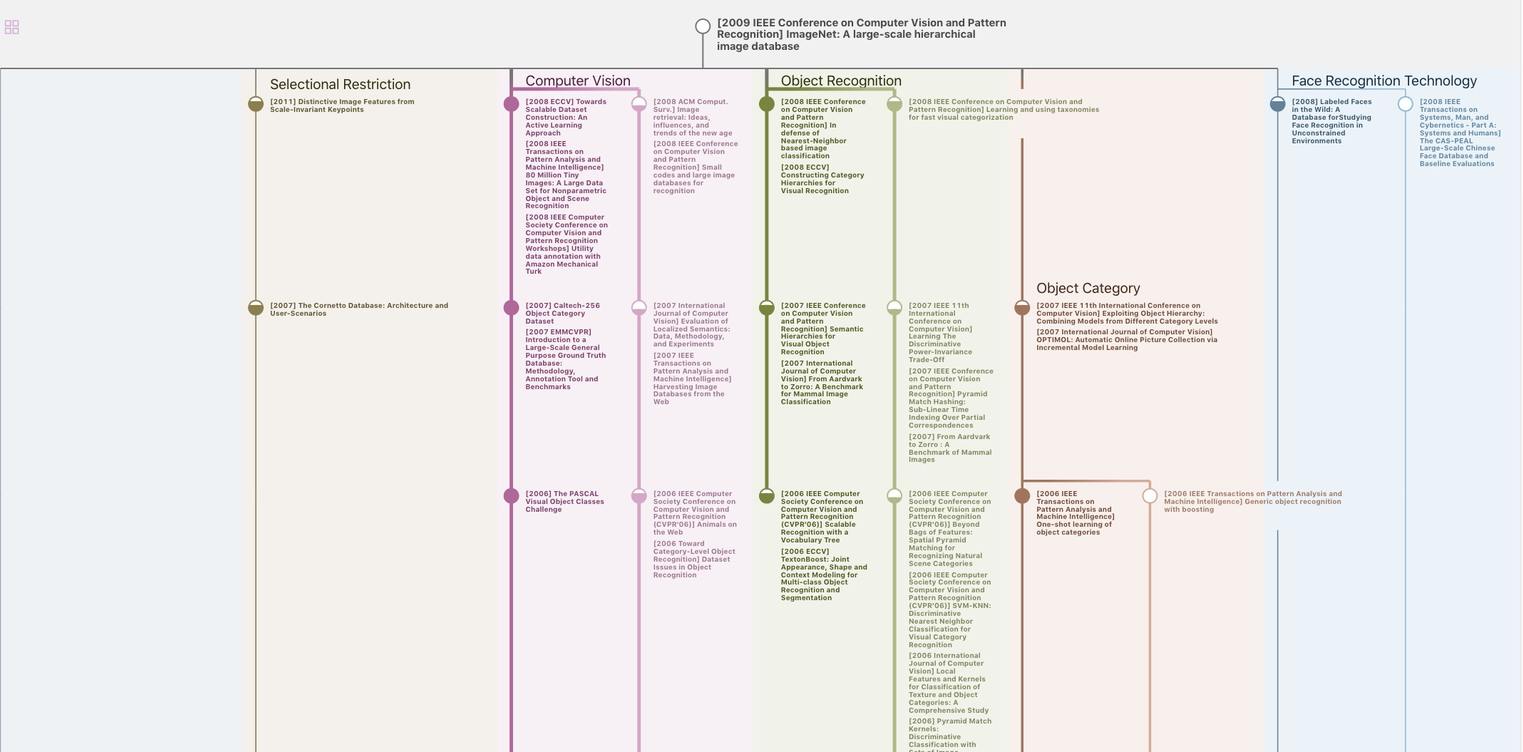
生成溯源树,研究论文发展脉络
Chat Paper
正在生成论文摘要