Joint entity structural and attribute information for knowledge graph completion
International Conference on High Performance Computing and Communication (HPCCE 2021)(2022)
摘要
Knowledge Graph Completion (KGC) aims at predicting missing information for knowledge graphs. Most methods concentrate on learning entities’ representations with structural information indicating the relations between entities, while the utilization of entity attribute information is not sufficient for KGC. How to use the complex and diverse entity attribute information for KGC is still a challenging problem. In this paper, we propose a novel joint model Entity Structural and Attribute Embedding (ESAE) for KGC, which takes advantage of the entity structural and attribute information. Specifically, we first design a novel encoder Attribute Encoder (AE), which encodes both entity attribute types and values to generate the entities’ attribute-based representations. Based on AE, we use the structure-based and attribute-based representations in ESAE. We evaluate our method on the KGC task. Experimental results on real-world datasets show that our method outperforms other baselines on KGC.
更多查看译文
AI 理解论文
溯源树
样例
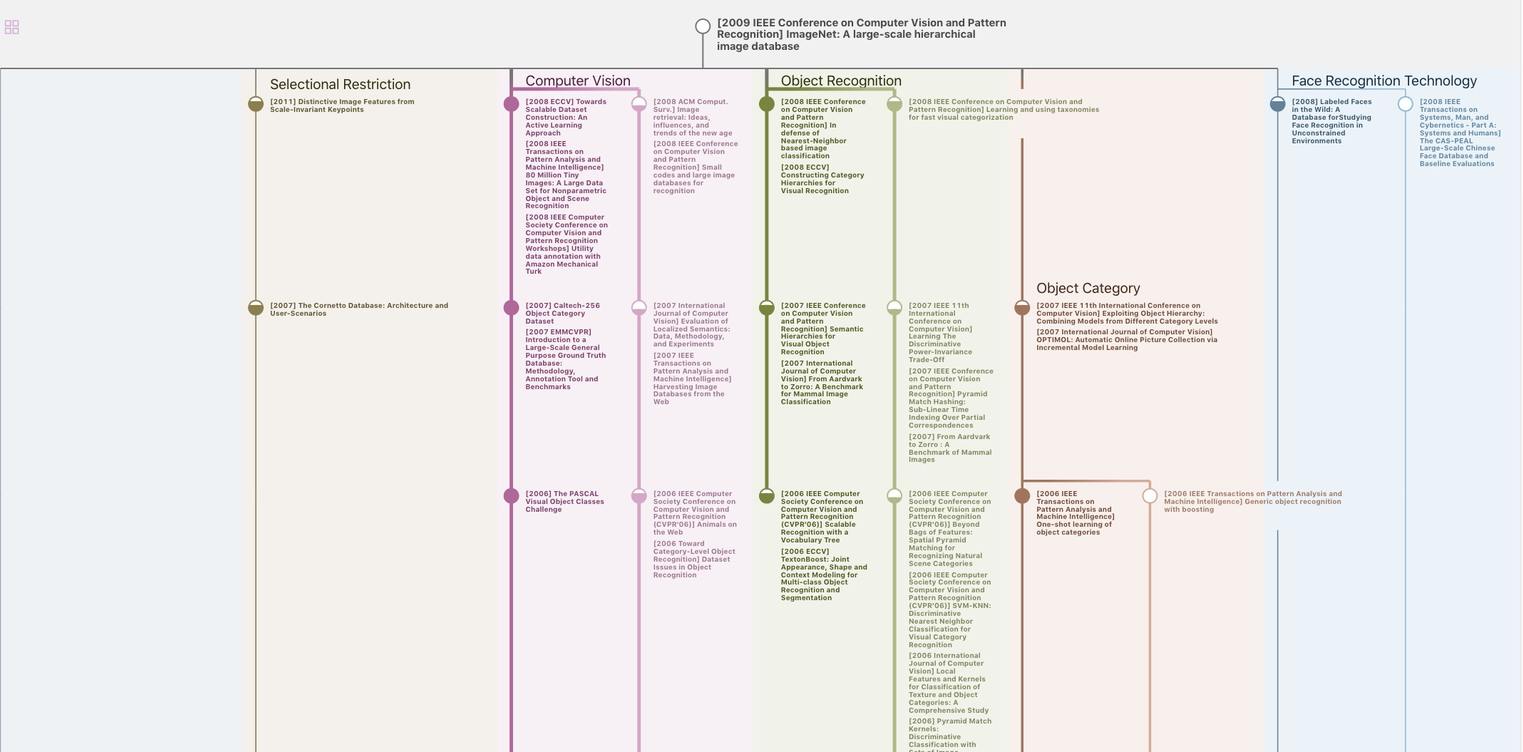
生成溯源树,研究论文发展脉络
Chat Paper
正在生成论文摘要