Physics-Informed Machine Learning for Modeling Turbulence in Supernovae
arxiv(2022)
摘要
Turbulence plays an important role in astrophysical phenomena, including core-collapse supernovae (CCSN), but current simulations must rely on subgrid models since direct numerical simulation (DNS) is too expensive. Unfortunately, existing subgrid models are not sufficiently accurate. Recently, Machine Learning (ML) has shown an impressive predictive capability for calculating turbulence closure. We have developed a physics-informed convolutional neural network (CNN) to preserve the realizability condition of Reynolds stress that is necessary for accurate turbulent pressure prediction. The applicability of the ML subgrid model is tested here for magnetohydrodynamic (MHD) turbulence in both the stationary and dynamic regimes. Our future goal is to utilize this ML methodology (available on GitHub) in the CCSN framework to investigate the effects of accurately-modeled turbulence on the explosion of these stars.
更多查看译文
关键词
turbulence,machine learning,physics-informed
AI 理解论文
溯源树
样例
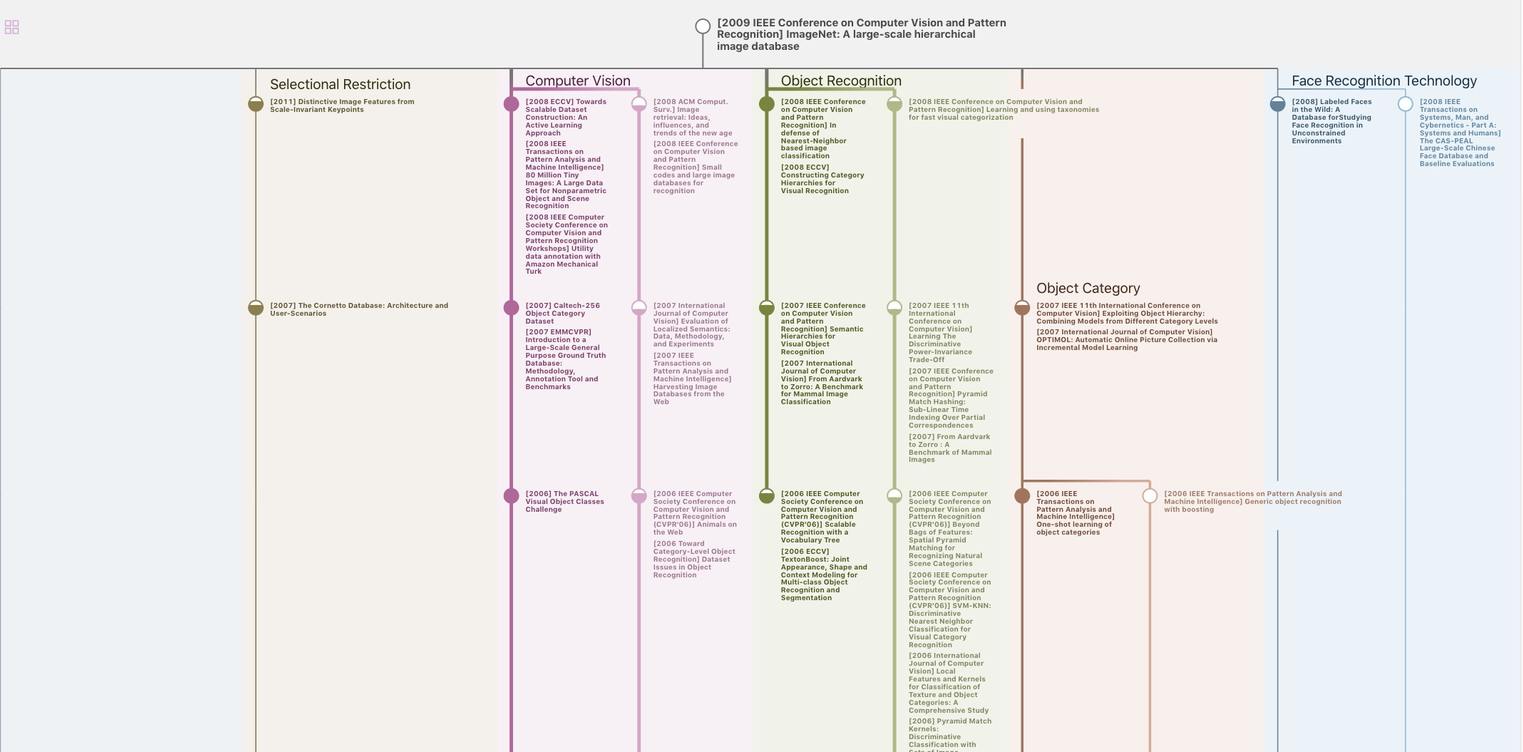
生成溯源树,研究论文发展脉络
Chat Paper
正在生成论文摘要