Planning, Execution, and Adaptation for Multi-Robot Systems using Probabilistic and Temporal Planning.
International Joint Conference on Autonomous Agents and Multi-agent Systems(2022)
摘要
Planning for multi-robot coordination during long horizon missions in complex environments need to consider resources, temporal constraints, and uncertainty. This could be computationally expensive and impractical for online planning and execution. We propose a decoupled framework to address this. At the high-level, we plan for multi-robot missions that require coordination amongst robots considering temporal and numeric constraints. The temporal plan is decomposed into low-level plans for individual robots. At the low-level, we perform online learning and adaptation due to unexpected probabilistic outcomes to achieve mission goals. Our framework learns over time to improve the performance by (1) updating the learned domain model to reduce model prediction errors and (2) constraining the robot's capabilities which in turn improves goal allocation. The approach provides a solution to planning problems that require long-term robot operability. We demonstrate the performance of our approach via experiments involving a fleet of heterogeneous robots.
更多查看译文
AI 理解论文
溯源树
样例
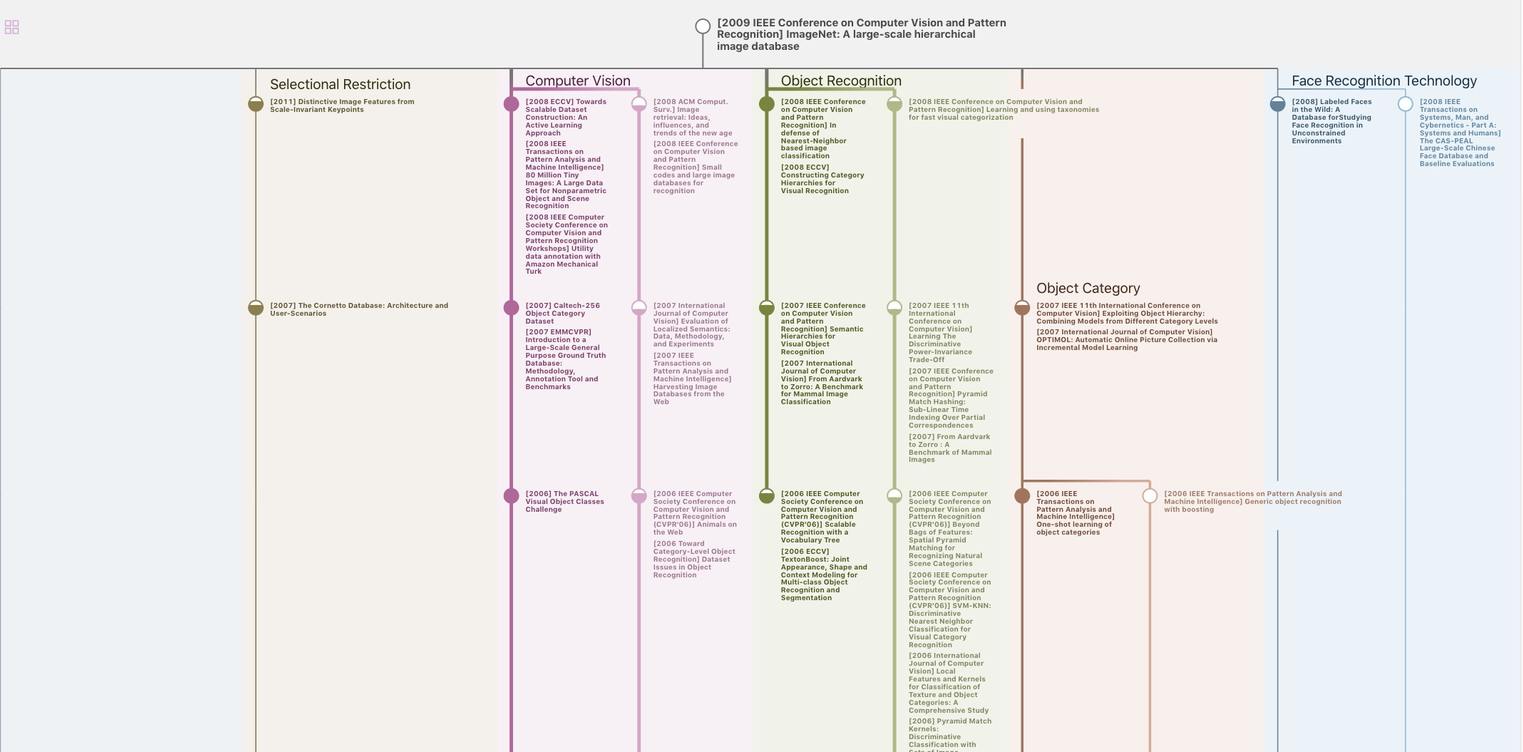
生成溯源树,研究论文发展脉络
Chat Paper
正在生成论文摘要