Multi-lag and multi-type temporal causality inference and analysis for industrial process fault diagnosis
Control Engineering Practice(2022)
摘要
Causality analysis methods play an increasingly crucial role in revealing the underlying mechanisms and evolution of process faults in industry. Indeed, in modern complex and integrated industrial systems, causation among various components usually have multi-lag and multi-type characteristics, which means that the time-delay of fault propagation between various process variables is multiple, and there exist both linear and nonlinear causal relations. Such characteristics make conventional causal inference tools ineffective. In this study, a systematic root cause diagnosis strategy is proposed. First, a modular neural network structure, termed Sparse Causal Residual Neural Network(SCRNN) is designed to concurrently extract multi-lag linear and nonlinear causal relations. By optimizing a multivariate time series forecasting objective with hierarchical sparsity constraints, the integral causal structure can be interpreted by checking the parameters of the SCRNN model. This causal structure represents the topology of fault propagation. Furthermore, an R-value metric is devised to quantify the position of each faulty variable and hence pinpoint the root cause accordingly. A numerical case, a benchmark process and a real industrial process illustrate the practicability and superiority of the proposed method.
更多查看译文
关键词
Root cause diagnosis,Causality analysis,Sparse normalization,R-value metric,Fault propagation path analysis
AI 理解论文
溯源树
样例
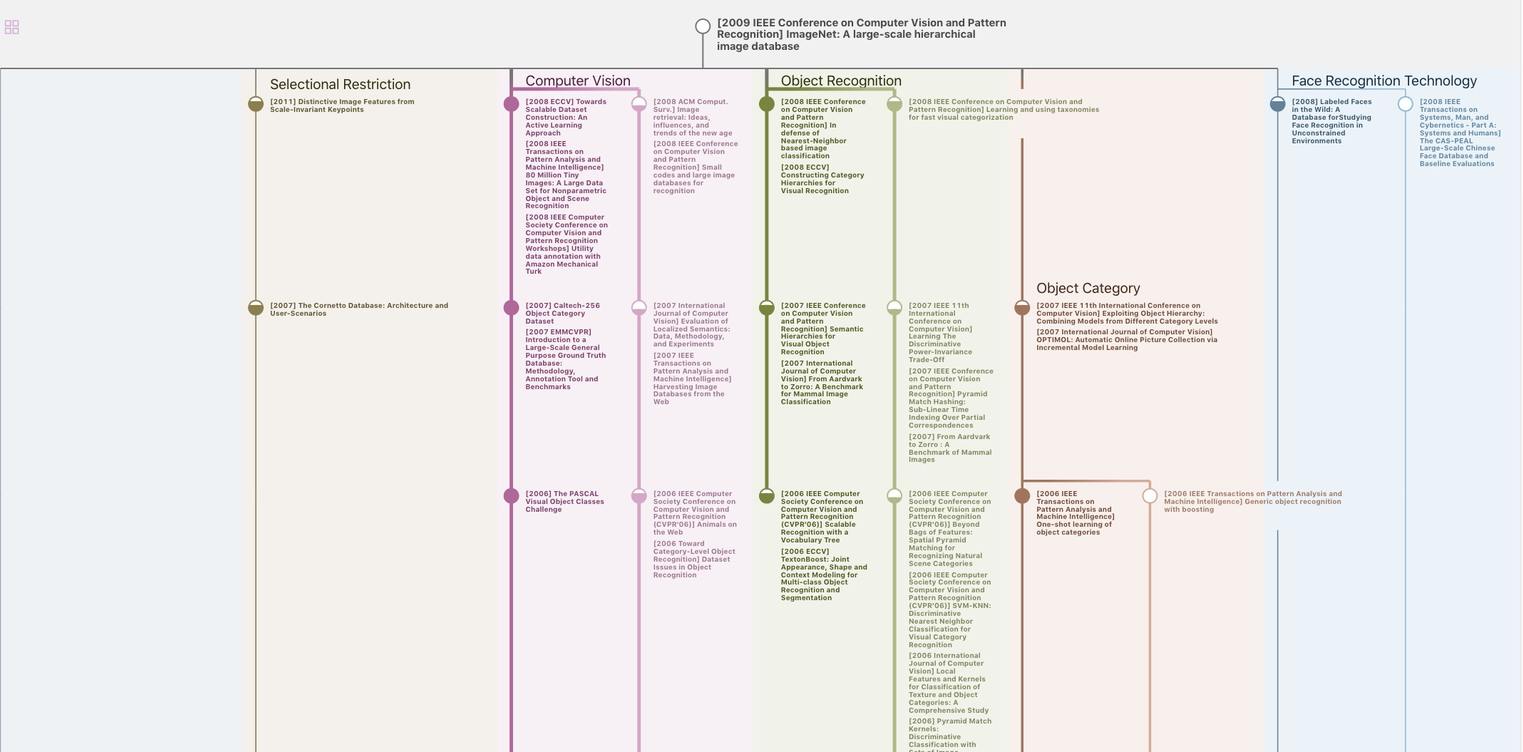
生成溯源树,研究论文发展脉络
Chat Paper
正在生成论文摘要