ColonFormer: An Efficient Transformer Based Method for Colon Polyp Segmentation
IEEE ACCESS(2022)
摘要
Identifying polyps is challenging for automatic analysis of endoscopic images in computer-aided clinical support systems. Models based on convolutional networks (CNN), transformers, and their combinations have been proposed to segment polyps with promising results. However, those approaches have limitations either in modeling the local appearance of the polyps only or lack of multi-level feature representation for spatial dependency in the decoding process. This paper proposes a novel network, namely ColonFormer, to address these limitations. ColonFormer is an encoder-decoder architecture capable of modeling long-range semantic information at both encoder and decoder branches. The encoder is a lightweight architecture based on transformers for modeling global semantic relations at multi scales. The decoder is a hierarchical network structure designed for learning multi-level features to enrich feature representation. Besides, a refinement module is added with a new skip connection technique to refine the boundary of polyp objects in the global map for accurate segmentation. Extensive experiments have been conducted on five popular benchmark datasets for polyp segmentation, including Kvasir, CVC-Clinic DB, CVC-ColonDB, CVC-T, and ETIS-Larib. Experimental results show that our ColonFormer outperforms other state-of-the-art methods on all benchmark datasets. Our code is available at: https://github.com/ducnt9907/ColonFormer.
更多查看译文
关键词
Transformers, Image segmentation, Computer architecture, Decoding, Feature extraction, Convolutional neural networks, Computational modeling, Polyp segmentation, deep learning, encoder-decoder network, hierarchical multi-scale CNN, computer-aided diagnosis
AI 理解论文
溯源树
样例
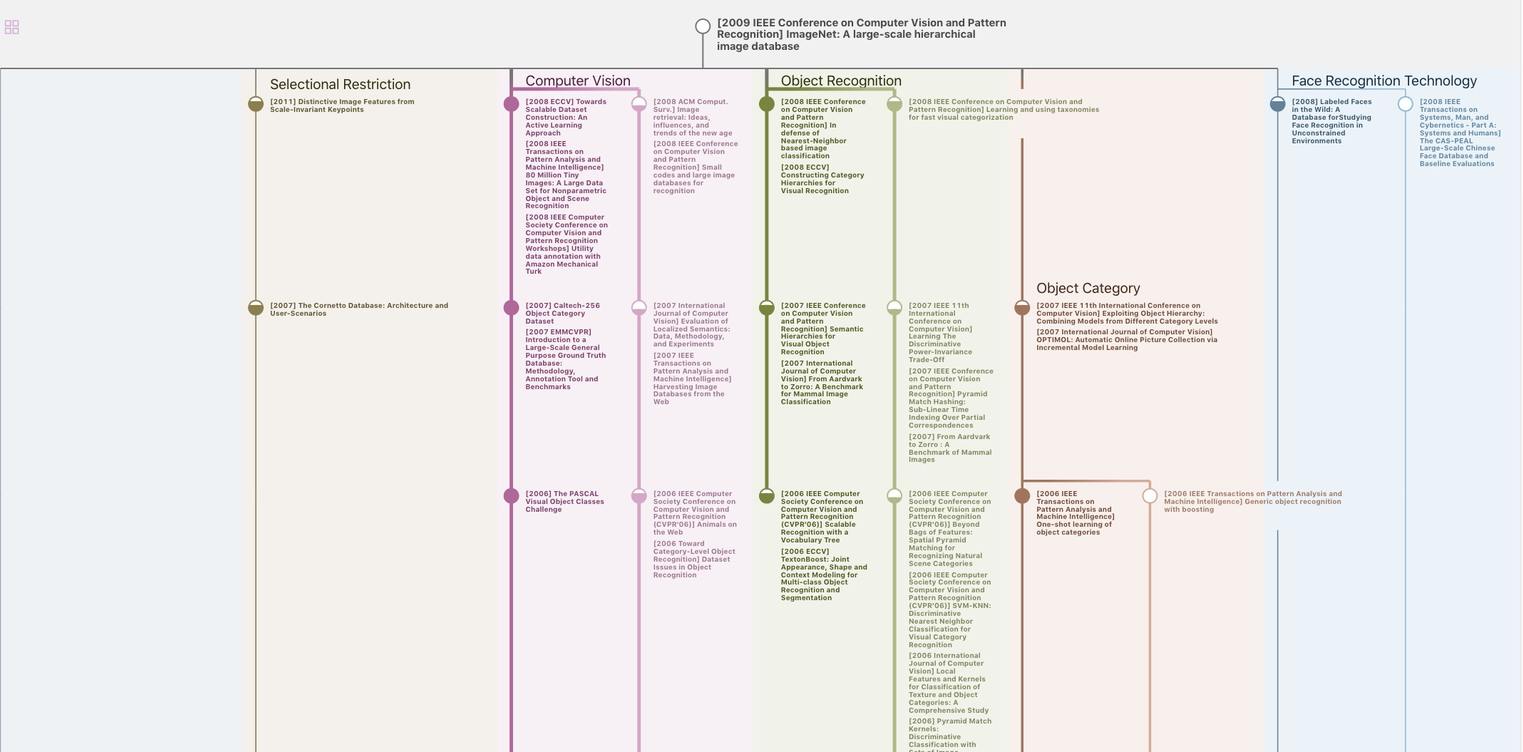
生成溯源树,研究论文发展脉络
Chat Paper
正在生成论文摘要