Bankrupting DoS Attackers Despite Uncertainty
arxiv(2022)
摘要
On-demand provisioning in the cloud allows for services to remain available despite massive denial-of-service (DoS) attacks. Unfortunately, on-demand provisioning is expensive and must be weighed against the costs incurred by an adversary. This leads to a recent threat known as {\it economic denial-of-sustainability (EDoS)}, where the cost for defending a service is higher than that of attacking. A natural tool for combating EDoS is to impose costs via resource burning (RB). Here, a client must verifiably consume resources -- for example, by solving a computational challenge -- before service is rendered. However, prior RB-based defenses with security guarantees do not account for the cost of on-demand provisioning. Another common approach is the use of heuristics -- such as a client's reputation score or the geographical location -- to identify and discard spurious job requests. However, these heuristics may err and existing approaches do not provide security guarantees when this occurs. Here, we propose an EDoS defense, LCharge, that uses resource burning while accounting for on-demand provisioning. LCharge leverages an estimate of the number of job requests from honest clients (i.e., good jobs) in any set $S$ of requests to within an $O(\alpha)$-factor, for any unknown $\alpha>0$, but retains a strong security guarantee despite the uncertainty of this estimate. Specifically, against an adversary that expends $B$ resources to attack, the total cost for defending is $O( \alpha^{5/2}\sqrt{B\,(g+1)} + \alpha^3(g+\alpha))$ where $g$ is the number of good jobs. Notably, for large $B$ relative to $g$ and $\alpha$, the adversary has higher cost, implying that the algorithm has an economic advantage. Finally, we prove a lower bound for our problem of $\Omega(\sqrt{\alpha B g})$, showing that the cost of LCharge is asymptotically tight for $\alpha=\Theta(1)$.
更多查看译文
AI 理解论文
溯源树
样例
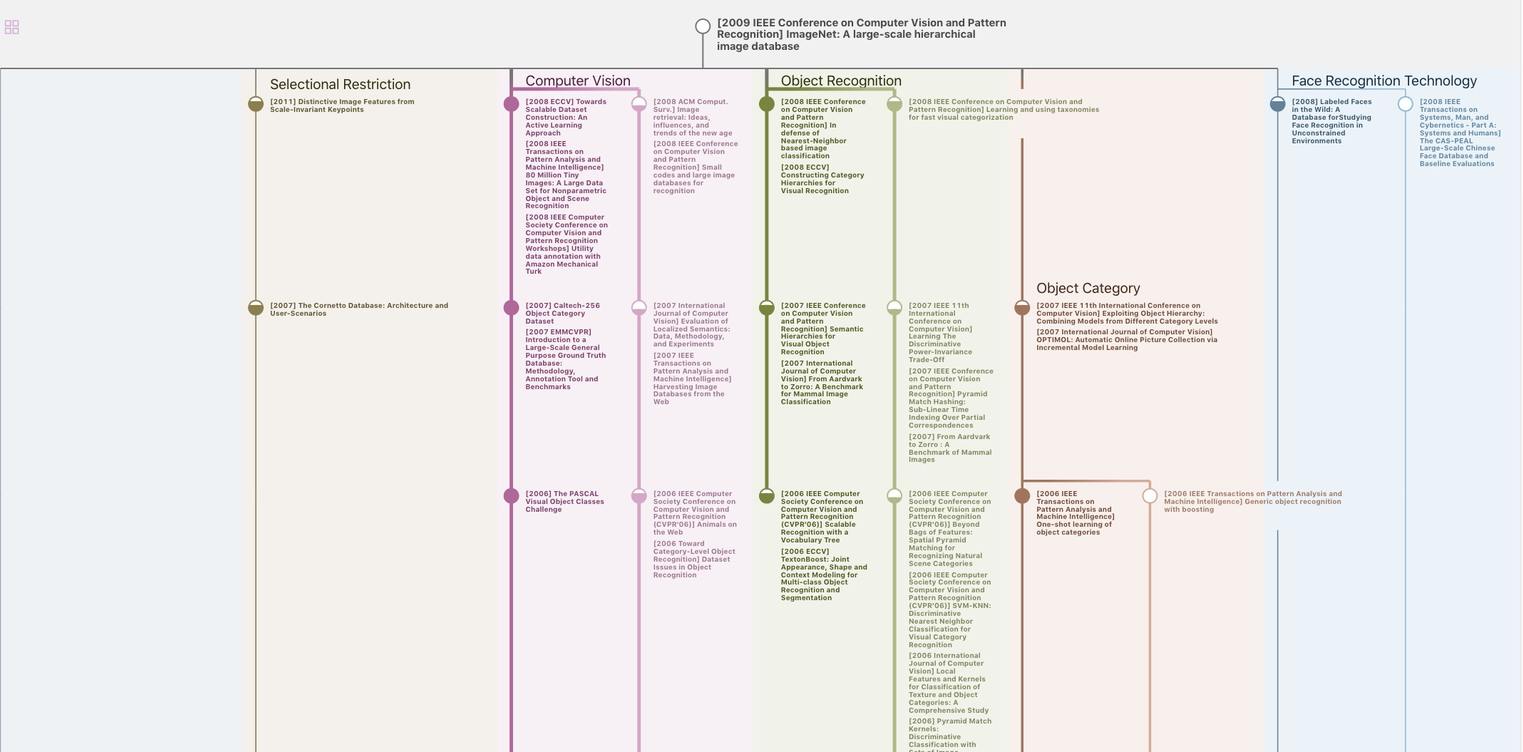
生成溯源树,研究论文发展脉络
Chat Paper
正在生成论文摘要