Depression Diagnosis and Forecast based on Mobile Phone Sensor Data.
Annual International Conference of the IEEE Engineering in Medicine and Biology Society (EMBC)(2022)
摘要
Previous studies have shown the correlation be-tween sensor data collected from mobile phones and human depression states. Compared to the traditional self-assessment questionnaires, the passive data collected from mobile phones is easier to access and less time-consuming. In particular, passive mobile phone data can be collected on a flexible time interval, thus detecting moment-by-moment psychological changes and helping achieve earlier interventions. Moreover, while previous studies mainly focused on depression diagnosis using mobile phone data, depression forecasting has not received sufficient attention. In this work, we extract four types of passive features from mobile phone data, including phone call, phone usage, user activity, and GPS features. We implement a long short-term memory (LSTM) network in a subject-independent 10-fold cross-validation setup to model both a diagnostic and a forecasting tasks. Experimental results show that the forecasting task achieves comparable results with the diagnostic task, which indicates the possibility of forecasting depression from mobile phone sensor data. Our model achieves an accuracy of 77.0 % for major depression forecasting (binary), an accuracy of 53.7 % for depression severity forecasting (5 classes), and a best RMSE score of 4.094 (PHQ-9, range from 0 to 27).
更多查看译文
关键词
Cell Phone,Depression,Depressive Disorder,Humans,Surveys and Questionnaires
AI 理解论文
溯源树
样例
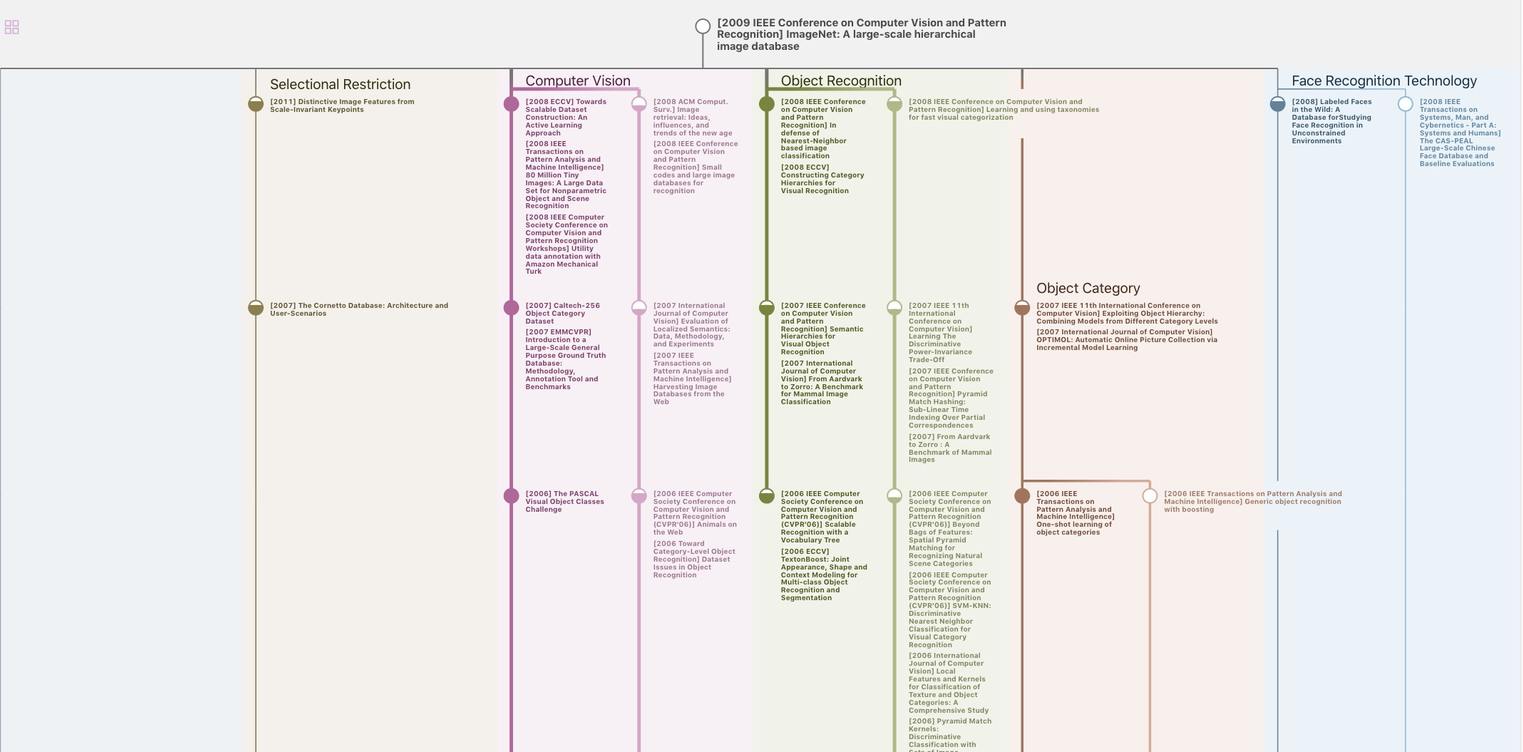
生成溯源树,研究论文发展脉络
Chat Paper
正在生成论文摘要