Autosegmentation based on different-sized training datasets of consistently-curated volumes and impact on rectal contours in prostate cancer radiation therapy
Physics and Imaging in Radiation Oncology(2022)
摘要
Background and purpose: Autosegmentation techniques are emerging as time-saving means for radiation therapy (RT) contouring, but the understanding of their performance on different datasets is limited. The aim of this study was to determine agreement between rectal volumes by an existing autosegmentation algorithm and manually-delineated rectal volumes in prostate cancer RT. We also investigated contour quality by differentsized training datasets and consistently-curated volumes for retrained versions of this same algorithm. Materials and methods: Single-institutional data from 624 prostate cancer patients treated to 50-70 Gy were used. Manually-delineated clinical rectal volumes (clinical) and consistently-curated volumes recontoured to one anatomical guideline (reference) were compared to autocontoured volumes by a commercial autosegmentation tool based on deep-learning (v1; n = 891, multiple-institutional data) and retrained versions using subsets of the curated volumes (v32/64/128/256; n = 32/64/128/256). Evaluations included dose-volume histogram metrics, Dice similarity coefficients, and Hausdorff distances; differences between groups were quantified using parametric or non-parametric hypothesis testing. Results: Volumes by v1-256 (76-78 cm(3)) were larger than reference (75 cm(3)) and clinical (76 cm(3)). Mean doses by v1-256 (24.2-25.2 Gy) were closer to reference (24.2 Gy) than to clinical (23.8 Gy). Maximum doses were similar for all volumes (65.7-66.0 Gy). Dice for v1-256 and reference (0.87-0.89) were higher than for v1-256 and clinical (0.86-0.87) with corresponding Hausdorff comparisons including reference smaller than comparisons including clinical (5-6 mm vs. 7-8 mm). Conclusion: Using small single-institutional RT datasets with consistently-defined rectal volumes when training autosegmentation algorithms created contours of similar quality as the same algorithm trained on large multiinstitutional datasets.
更多查看译文
关键词
Radiation therapy,Deep-learning,Autosegmentation,Prostate cancer,Rectum,CT
AI 理解论文
溯源树
样例
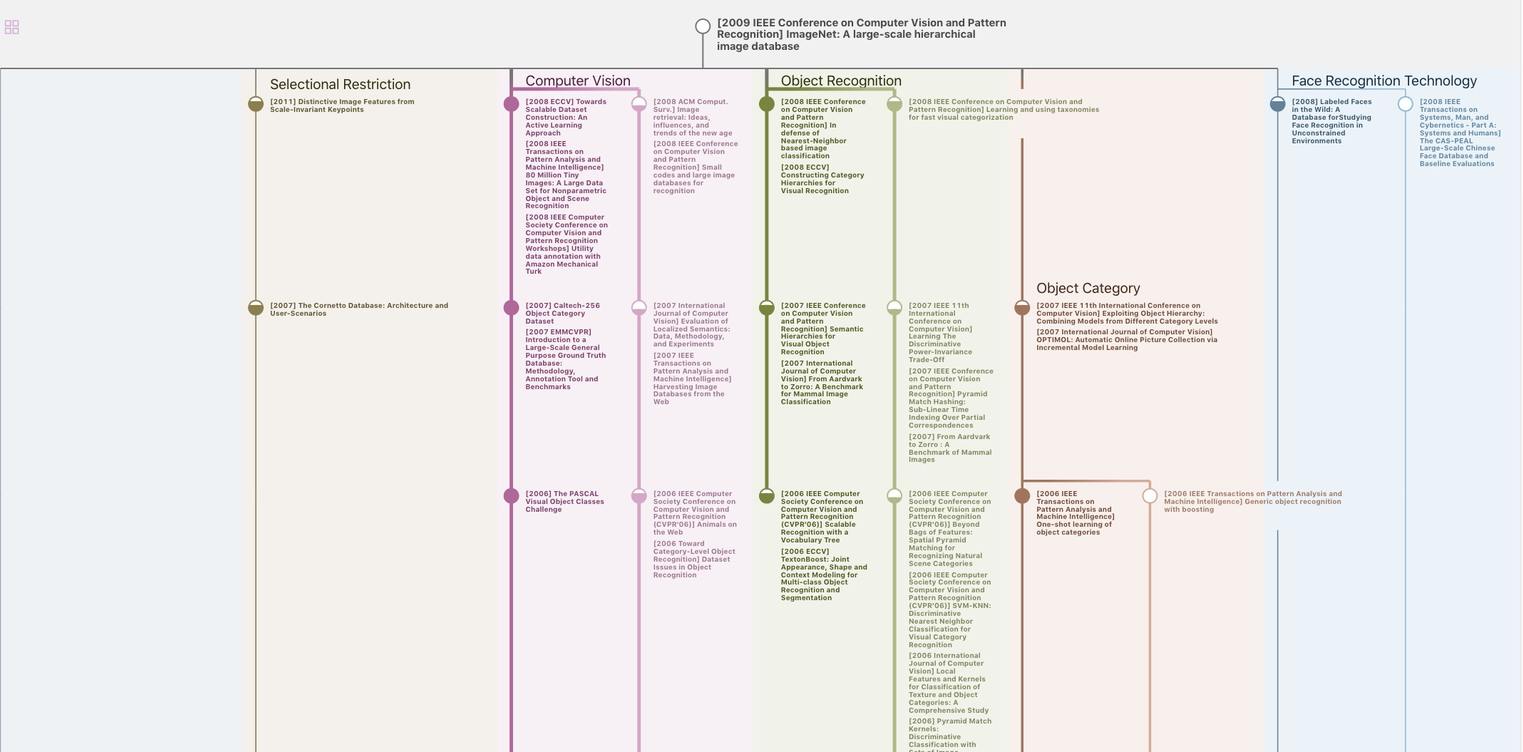
生成溯源树,研究论文发展脉络
Chat Paper
正在生成论文摘要