Pretraining Approaches for Spoken Language Recognition: TalTech Submission to the OLR 2021 Challenge.
The Speaker and Language Recognition Workshop (Odyssey)(2022)
摘要
This paper investigates different pretraining approaches to spoken language identification. The paper is based on our submission to the Oriental Language Recognition 2021 Challenge. We participated in two tracks of the challenge: constrained and unconstrained language recognition. For the constrained track, we first trained a Conformer-based encoder-decoder model for multilingual automatic speech recognition (ASR), using the provided training data that had transcripts available. The shared encoder of the multilingual ASR model was then finetuned for the language identification task. For the unconstrained task, we relied on both externally available pretrained models as well as external data: the multilingual XLSR-53 wav2vec2.0 model was finetuned on the VoxLingua107 corpus for the language recognition task, and finally finetuned on the provided target language training data, augmented with CommonVoice data. Our primary metric $C_{\rm avg}$ values on the Test set are 0.0079 for the constrained task and 0.0119 for the unconstrained task which resulted in the second place in both rankings. In post-evaluation experiments, we study the amount of target language data needed for training an accurate backend model, the importance of multilingual pretraining data, and compare different models as finetuning starting points.
更多查看译文
关键词
spoken language recognition,taltech
AI 理解论文
溯源树
样例
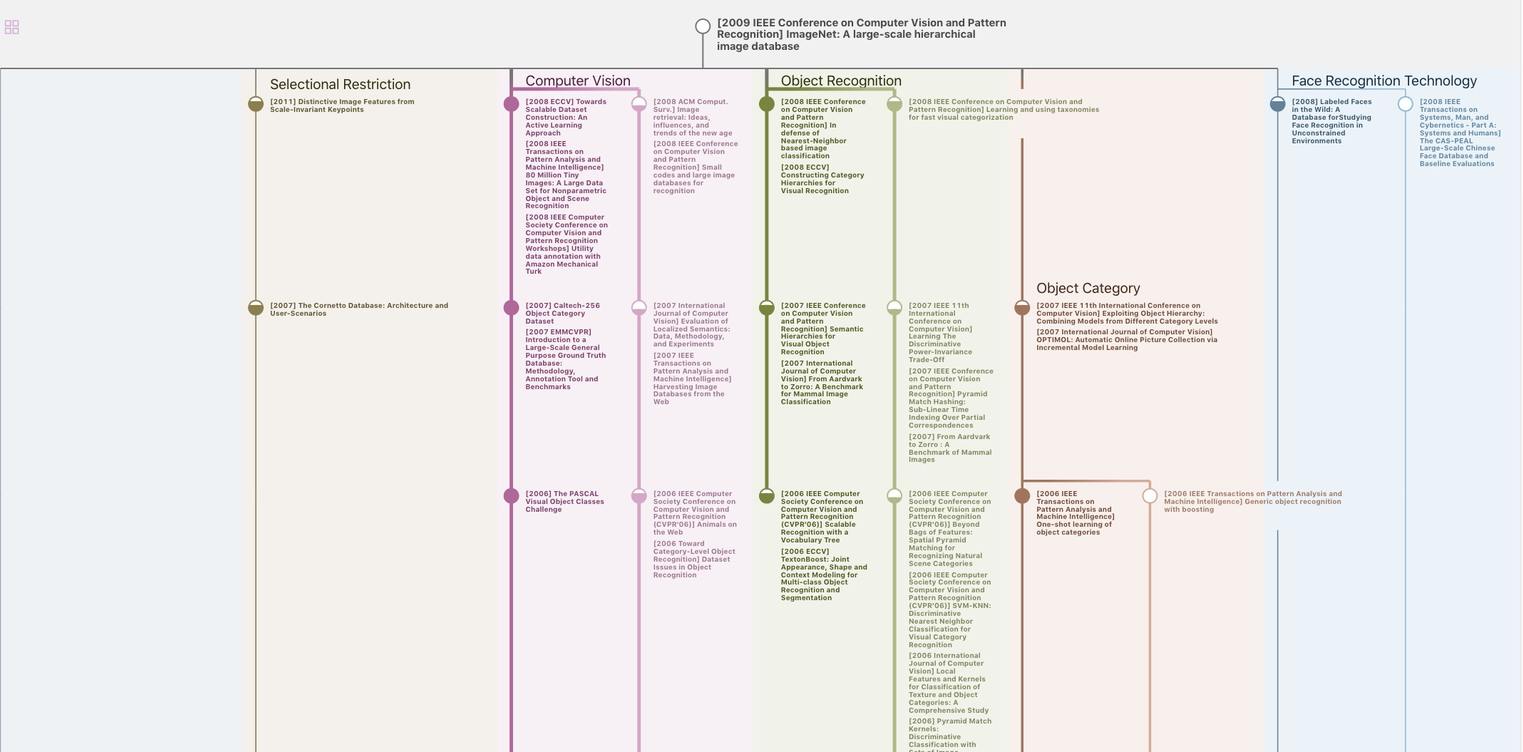
生成溯源树,研究论文发展脉络
Chat Paper
正在生成论文摘要