Molecular-fingerprint machine-learning-assisted design and prediction for high-performance MOFs for capture of NMHCs from air
Advanced powder materials(2022)
摘要
The capture of trace amounts of non-methane hydrocarbons (NMHCs) from air due to the toxicity of volatile organic compounds is a significant challenge. A total of 31399 hydrophobic metal–organic frameworks (MOFs) were first screened from 137953 hypothetical MOFs using high-throughput computational screening (HTCS), and their performance indices (adsorption capacity and selectivity) for the adsorption of NMHCs (C3–C6) were obtained by molecular simulations. The discovery of a “second peak” near twice the kinetic diameter of the corresponding NMHC provided more choices for excellent MOFs that adsorb NMHCs. Four machine learning (ML) classification and regression algorithms predicted the performance of MOFs, and the relative importance values of the six descriptors were determined. The combination of the Random Forests algorithm and Molecular ACCess Systems molecular fingerprint (MF) had an excellent predictive ability for MOFs. According to the performance, the fingerprint commonalities of the 100 top-performing MOFs were counted, and the excellent bits (EBs) that could promote the performance were defined. Finally, new substructures containing all of the EBs were designed for each NMHC to build a new MOF database. This work combined the HTCS, ML, and MF to provide a detailed insight into the design of efficient MOFs for adsorbing NMHCs.
更多查看译文
关键词
Non-methane hydrocarbons, Metal-organic framework, Adsorption, Molecular fingerprint
AI 理解论文
溯源树
样例
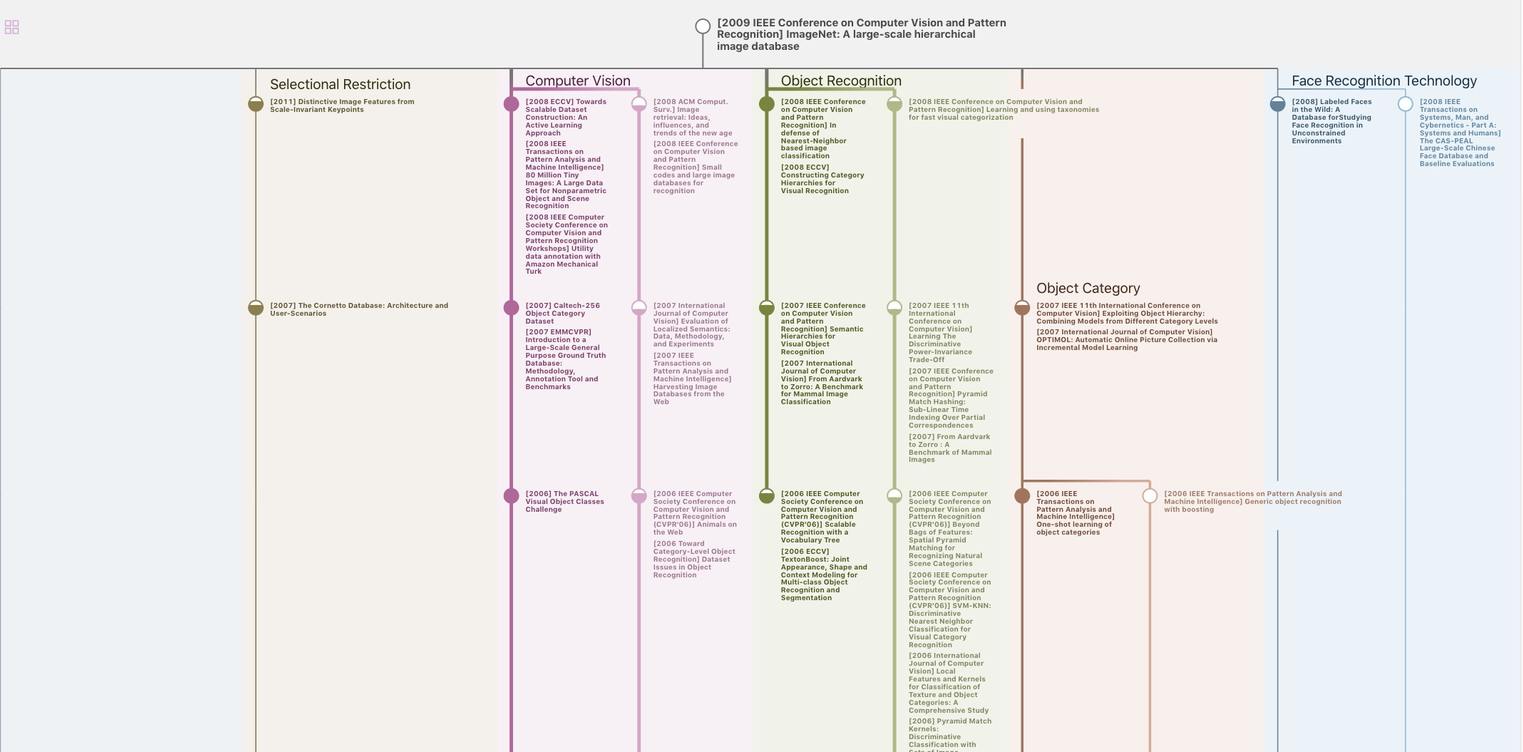
生成溯源树,研究论文发展脉络
Chat Paper
正在生成论文摘要