Association Hierarchical Representation Learning for Plant-Wide Process Monitoring by Using Multilevel Knowledge Graph.
IEEE transactions on artificial intelligence(2023)
摘要
In order to satisfy the safety requirements of plant-wide processes, distributed process monitoring methods are often used. However, few of them consider the problem on building multilevel knowledge blocks and the associations between different blocks, all of which are beneficial for plant-wide process monitoring in avoiding information conflict and even to improve monitoring accuracy. To handle these issues, a plant-wide process monitoring method is proposed, which is based on hierarchical graph representation learning with differentiable pooling by using a multilevel knowledge graph (MLKG). Specifically, the MLKG consists of device level, subprocess level, process level, etc. Each level has numerous blocks (nodes), which is first constructed by prior knowledge on monitoring variables to calculate the status of key components, such as the Hotelling’s statistics. Then, normalized mutual information is used to obtain the associations between monitoring variables, and the status of each block on each level can be updated. Based on this method, the MLKG can be completely constructed. In order to consider the association information of hierarchical representations of the MLKG, hierarchical graph representation learning is used to achieve plant-wide process monitoring. Results of case study on practical cobalt and nickel removal from zinc solution demonstrate the effectiveness and applicability of this method.
更多查看译文
关键词
Association hierarchical representation learning,association information,multilevel knowledge graph (MLKG),mutual information,plant-wide process monitoring
AI 理解论文
溯源树
样例
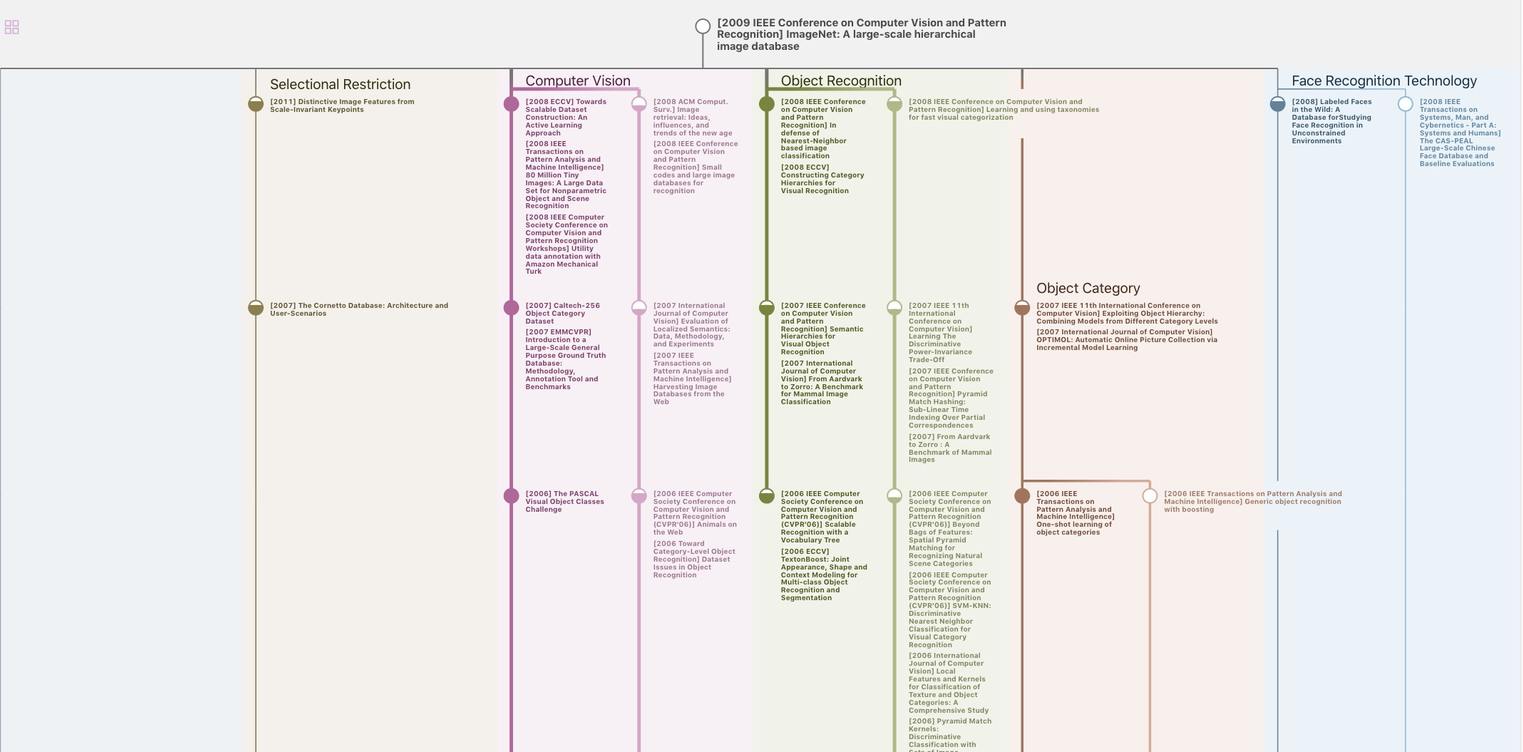
生成溯源树,研究论文发展脉络
Chat Paper
正在生成论文摘要