Investigating Hourly Global Horizontal Irradiance Forecasting Using Long Short-Term Memory
2021 IEEE Asia-Pacific Conference on Computer Science and Data Engineering (CSDE)(2021)
摘要
Solar energy is one of the cleanest renewable energy sources available. Several studies proposed models for Global Horizontal Irradiance (GHI) forecasting, which showed that deep learning models such as Long Short-Term Memory (LSTM) could be successful at this task. However, these models needed relatively large amounts of training data to reach excellent accuracy, which may be challenging for locations where only limited data are available. This experiment aims to determine the minimum amount of historical data needed by researchers to forecast GHI one hour ahead, using a deep learning LSTM model whilst maintaining an excellent accuracy range measured by nRMSE. To achieve this objective, we trained an LSTM model with different amounts of training data, starting with five years of training data then gradually reducing the amount by one year in each trial until using only one year of training data. The accuracy of the model in terms of nRMSE is reported in each trial. The experiments were conducted using historical GHI and meteorological data from three locations in Saudi Arabia. It is concluded that it is possible for an LSTM model to achieve excellent GHI forecasting accuracy (nRMSE<10%) using only two years of training data.
更多查看译文
关键词
solar irradiance,forecasting,GHI,prediction,timeseries,LSTM,deep learning
AI 理解论文
溯源树
样例
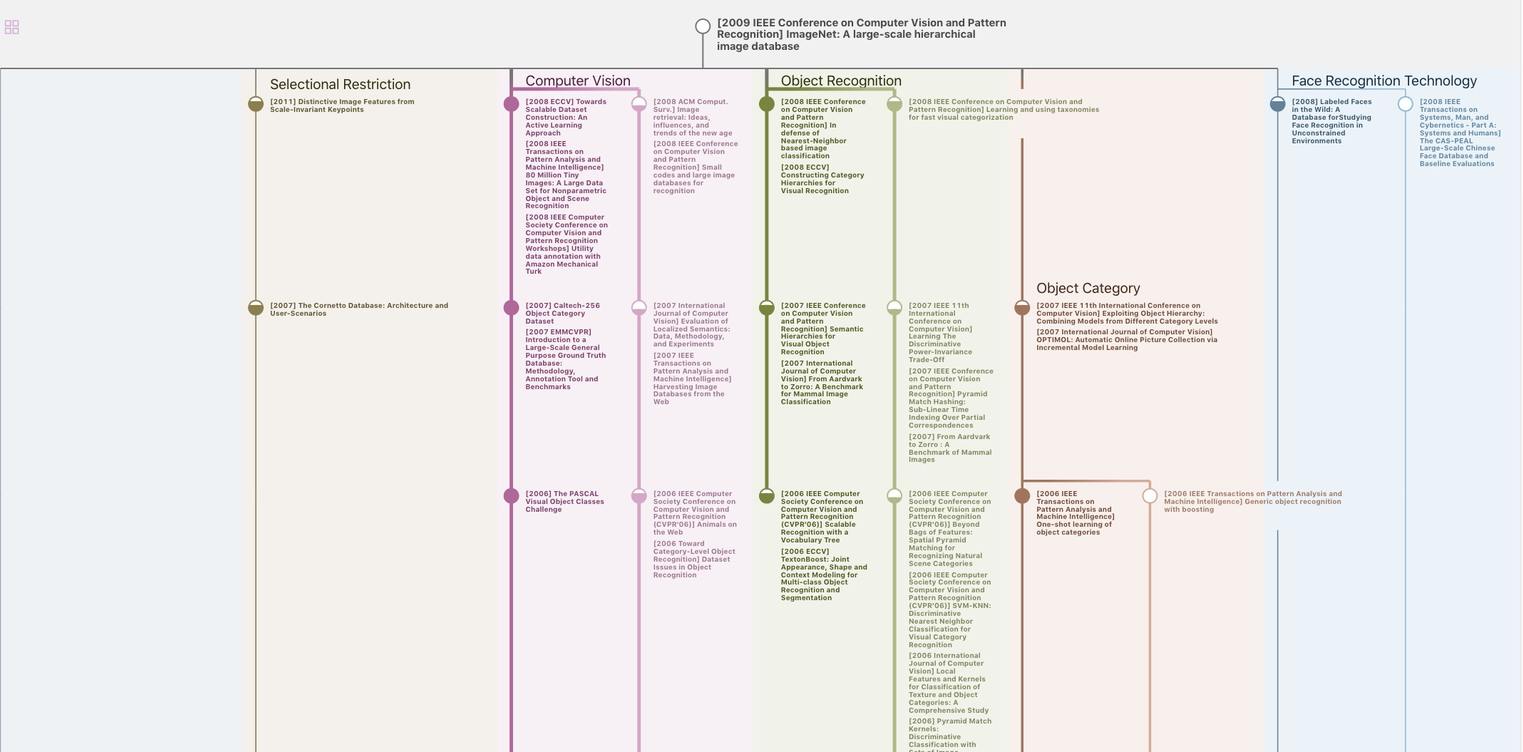
生成溯源树,研究论文发展脉络
Chat Paper
正在生成论文摘要