Beyond Static Models and Test Sets: Benchmarking the Potential of Pre-trained Models Across Tasks and Languages
PROCEEDINGS OF THE FIRST WORKSHOP ON EFFICIENT BENCHMARKING IN NLP (NLP POWER 2022)(2022)
摘要
Although recent Massively Multilingual Language Models (MMLMs) like mBERT and XLMR support around 100 languages, most existing multilingual NLP benchmarks provide evaluation data in only a handful of these languages with little linguistic diversity. We argue that this makes the existing practices in multilingual evaluation unreliable and does not provide a full picture of the performance of MMLMs across the linguistic landscape. We propose that the recent work done in Performance Prediction for NLP tasks can serve as a potential solution in fixing benchmarking in Multilingual NLP by utilizing features related to data and language typology to estimate the performance of an MMLM on different languages. We compare performance prediction with translating test data with a case study on four different multilingual datasets, and observe that these methods can provide reliable estimates of the performance that are often on-par with the translation based approaches, without the need for any additional translation as well as evaluation costs.
更多查看译文
关键词
benchmarking,static models,test sets,languages,pre-trained
AI 理解论文
溯源树
样例
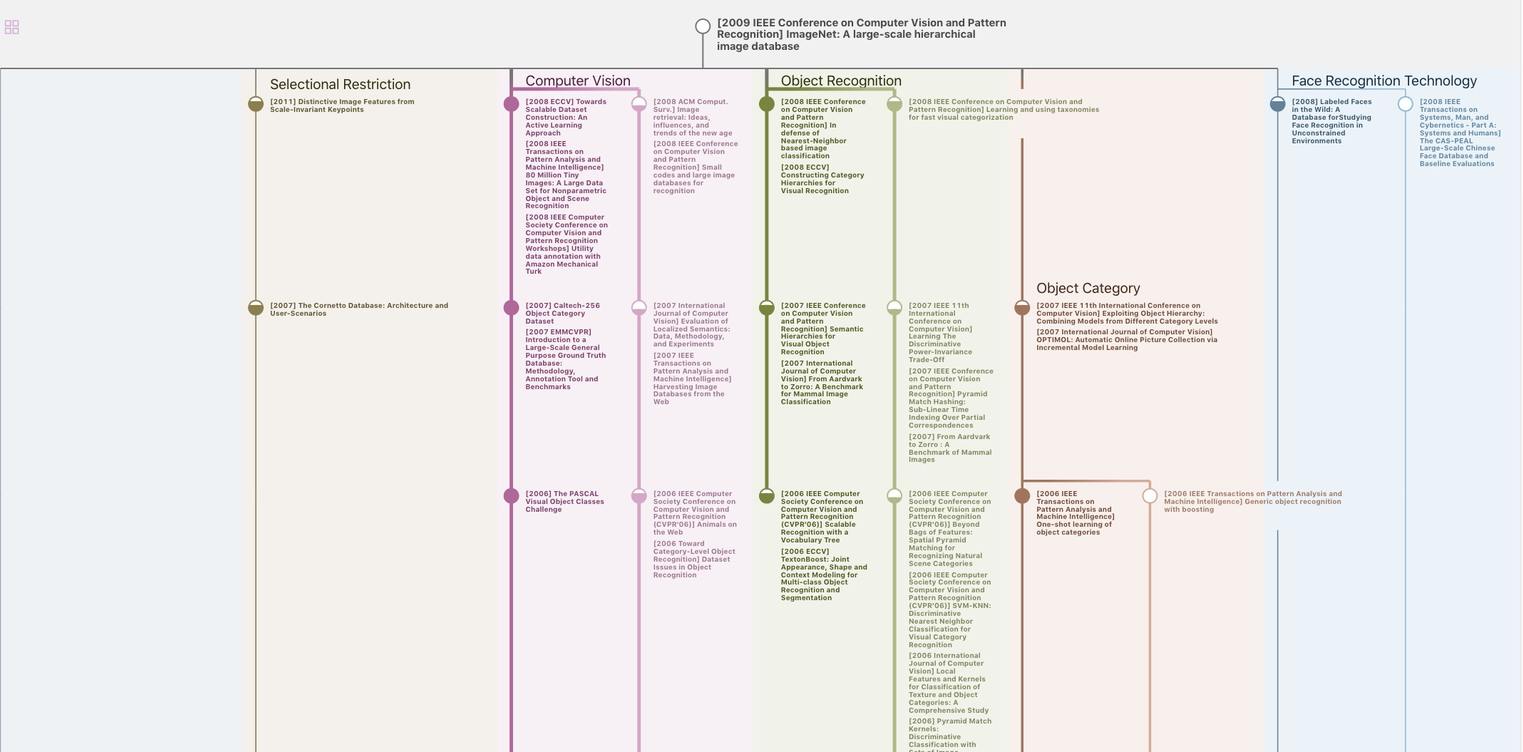
生成溯源树,研究论文发展脉络
Chat Paper
正在生成论文摘要