Multi-Environment Meta-Learning in Stochastic Linear Bandits.
International Symposium on Information Theory (ISIT)(2022)
摘要
In this work we investigate meta-learning (or learning-to-learn) approaches in multi-task linear stochastic bandit problems that can originate from multiple environments. Inspired by the work of [1] on meta-learning in a sequence of linear bandit problems whose parameters are sampled from a single distribution (i.e., a single environment), here we consider the feasibility of meta-learning when task parameters are drawn from a mixture distribution instead. For this problem, we propose a regularized version of the OFUL algorithm that, when trained on tasks with labeled environments, achieves low regret on a new task without requiring knowledge of the environment from which the new task originates. Specifically, our regret bound for the new algorithm captures the effect of environment misclassification and highlights the benefits over learning each task separately or meta-learning without recognition of the distinct mixture components.
更多查看译文
关键词
Meta-learning,Linear Stochastic Bandit,Sequential Decision Making
AI 理解论文
溯源树
样例
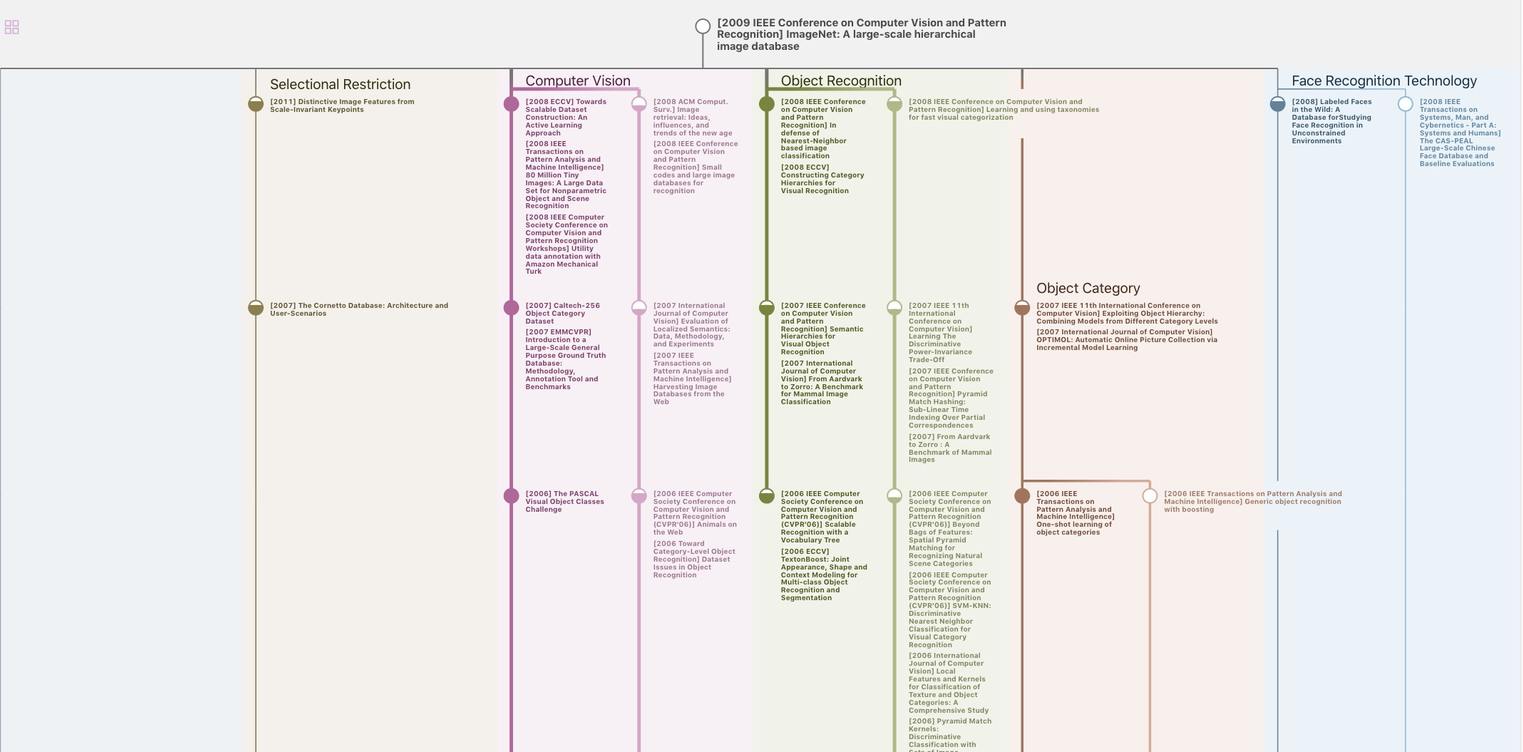
生成溯源树,研究论文发展脉络
Chat Paper
正在生成论文摘要