Analytical Needs for Machine Learning-based Image Analysis of Brainstem Astrocyte Cell Function.
FASEB journal : official publication of the Federation of American Societies for Experimental Biology(2022)
摘要
Reactive astrogliosis represents a neuropathogical finding in most brain diseases across the lifespan. Despite its near ubiquitous presence in CNS pathologies, the consequences of reactive astrogliosis on cell function are poorly characterized. We address the capability gap within the glial biology community by applying machine learning image segmentation and multivariate analyses to understand the consequences of gliosis on astrocyte cell function. We utilized live cell imaging of astrocytic intracellular calcium transients as a measure of physiological responses to external stimuli (i.e., exposure to lipopolysaccharide and/or hypoxic (100% N ) gas challenges). After obtaining 300 GBytes of live cell imaging footage, we developed an efficient, unbiased image segmentation workflow to capture astrocytes during physiological challenges. In contrast to prior methodologies which extracted only mean fluorescence intensity of segmented objects, we utilized pixel textures and fluorescent propagation in our analysis. Following the recommendations set forth by the recent consensus statement (Escartin et al., 2021), we performed multivariate and clustering analyses to identify distinct groups based on intracellular calcium transients waveforms under physiological challenges. These analyses reveal a diversity of cellular processes amongst brainstem astrocytes.
更多查看译文
AI 理解论文
溯源树
样例
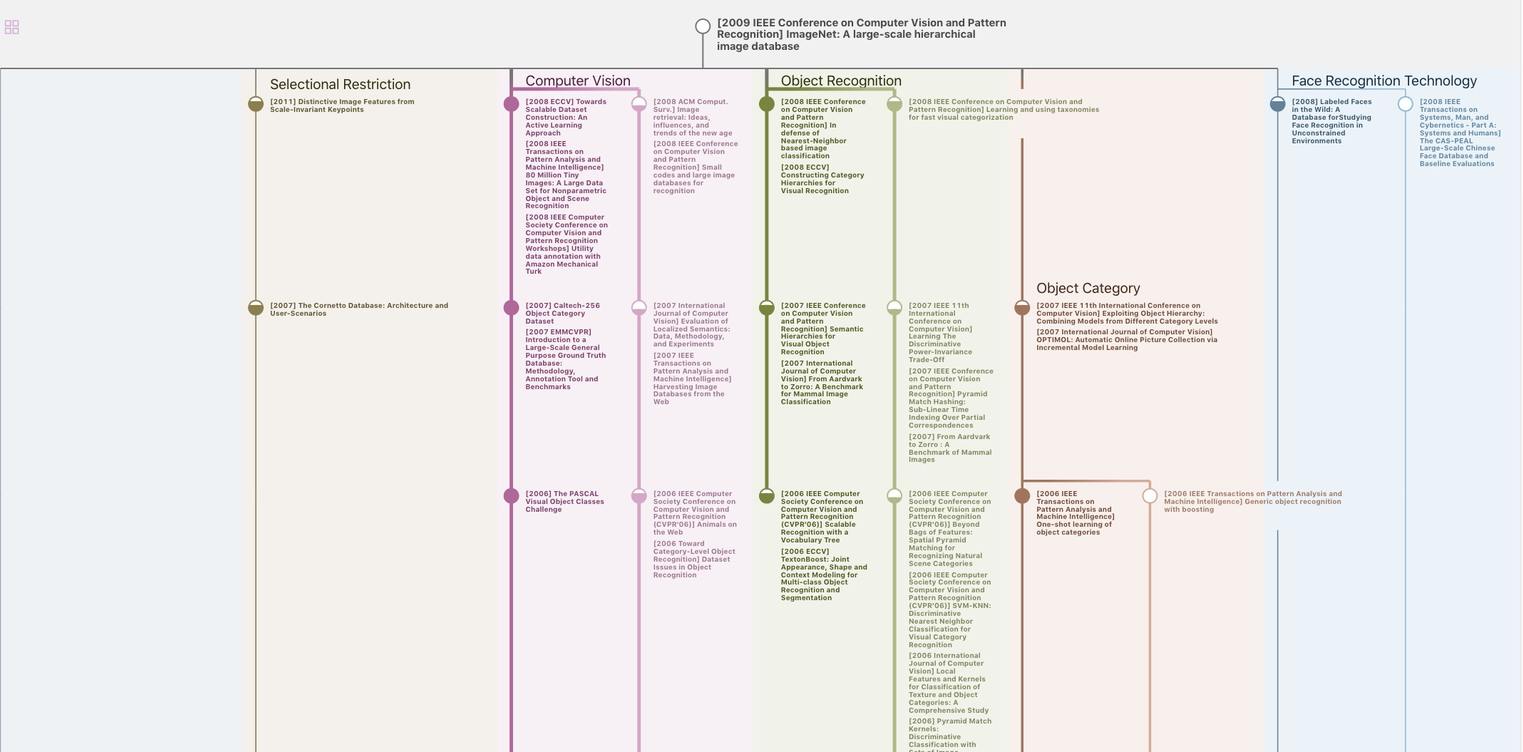
生成溯源树,研究论文发展脉络
Chat Paper
正在生成论文摘要