Wavelet based Machine Learning Approaches towards Precision Medicine in Diabetes Mellitus
FASEB journal : official publication of the Federation of American Societies for Experimental Biology(2022)
摘要
It is estimated that 422 million people around the world have diabetes mellitus (DM)-a devastating, complex, and highly heterogeneous disease-requesting better interventions based on disease subtyping. In this research, we utilize the discrete wavelet transform (DWT) to decompose and denoise DM data. Using DWT, we enhance heart rate variability (HRV) based DM diagnosis, data visualization of the disparities in Human Microbiome Project (HMP) data (gut bacteria, metabolomics, proteomics, RNA sequencing, targeted proteomics, and transcriptomics data) using demographic features, and insulin resistance prediction. We also attempt to forecast continuous glucose monitoring (CGM) ahead by 90 minutes because CGM is unable to provide real-time blood glucose measurements. We achieve 91.9% diagnosis accuracy for Type 1 DM using Random Forest on data transformed with DWT, holding the potential for usage in clinics. In addition, our DWT-based t-SNE and UMAP explorative analysis of HMP data support subtypes of prediabetic patients stratified by sex, race, and age. Moreover, DWT-based transformations provide multi-view clustering that any other methods would not provide on metabolomics, proteomics, RNA sequencing, targeted proteomics, and transcriptomics data and outperform those without DWT. Taken together, DWT-based machine learning approaches enable a fine resolution of subtyping DM towards precision medicine.
更多查看译文
关键词
Diabetes Mellitus,Discrete M-band Wavelet Transform,Machine Learning,Precision Medicine,Data Visualization,t-SNE,UMAP
AI 理解论文
溯源树
样例
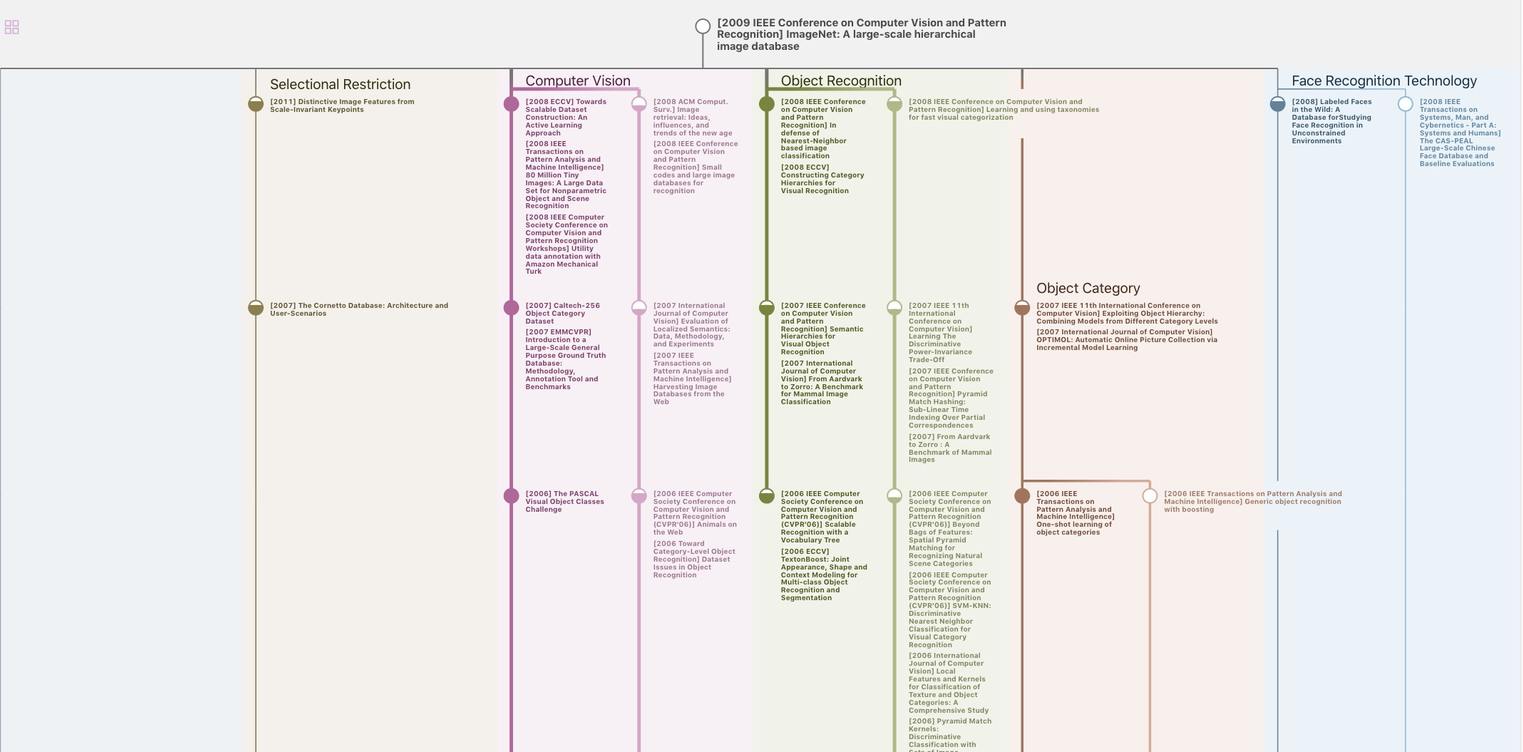
生成溯源树,研究论文发展脉络
Chat Paper
正在生成论文摘要