Nonconvex Nonlocal Tucker Decomposition for 3D Medical Image Super-Resolution
FRONTIERS IN NEUROINFORMATICS(2022)
摘要
Limited by hardware conditions, imaging devices, transmission efficiency, and other factors, high-resolution (HR) images cannot be obtained directly in clinical settings. It is expected to obtain HR images from low-resolution (LR) images for more detailed information. In this article, we propose a novel super-resolution model for single 3D medical images. In our model, nonlocal low-rank tensor Tucker decomposition is applied to exploit the nonlocal self-similarity prior knowledge of data. Different from the existing methods that use a convex optimization for tensor Tucker decomposition, we use a tensor folded-concave penalty to approximate a nonlocal low-rank tensor. Weighted 3D total variation (TV) is used to maintain the local smoothness across different dimensions. Extensive experiments show that our method outperforms some state-of-the-art (SOTA) methods on different kinds of medical images, including MRI data of the brain and prostate and CT data of the abdominal and dental.
更多查看译文
关键词
3D super-resolution, low rank tensor decomposition, nonlocal self-similarity, 3D total variation, medical image
AI 理解论文
溯源树
样例
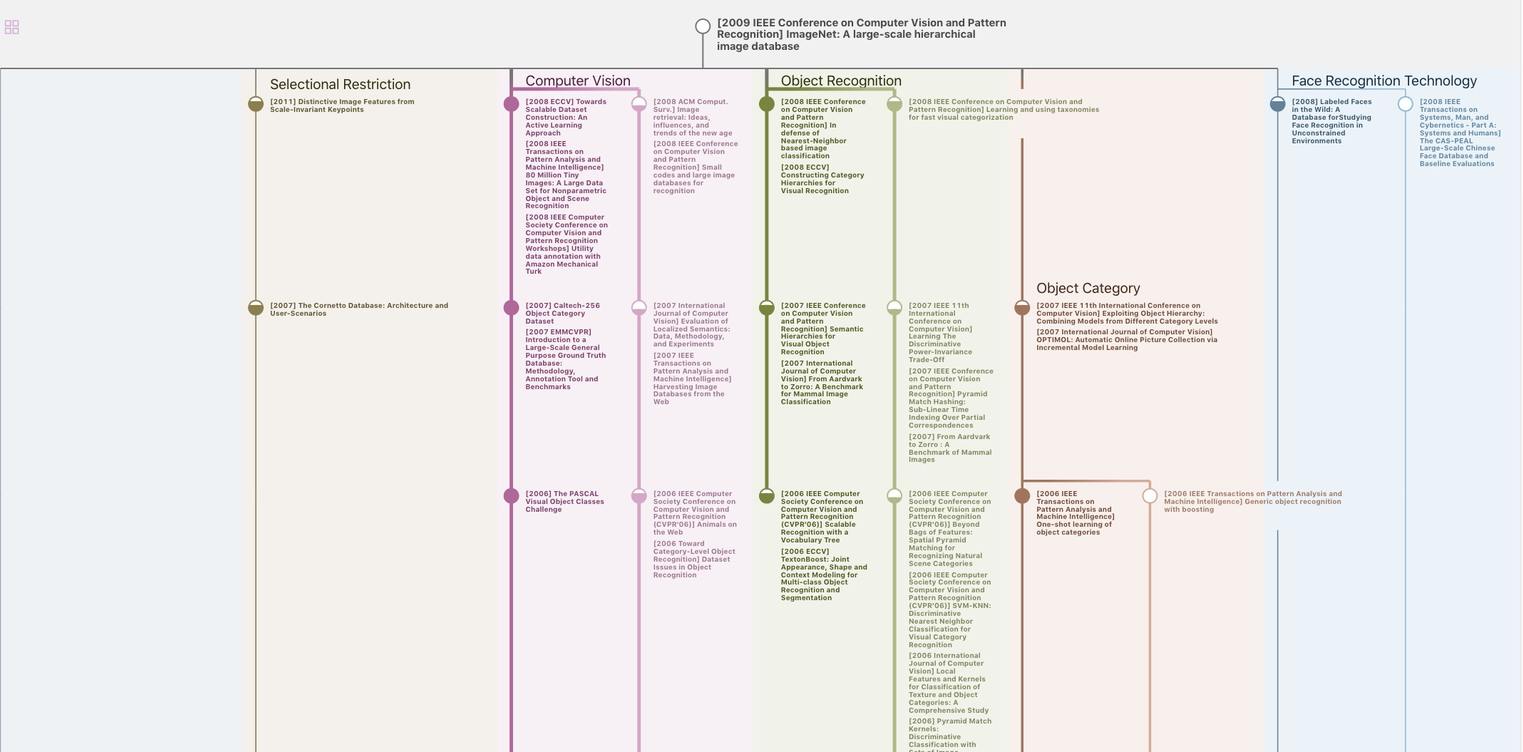
生成溯源树,研究论文发展脉络
Chat Paper
正在生成论文摘要