Transformer Grammars: Augmenting Transformer Language Models with Syntactic Inductive Biases at Scale.
CoRR(2022)
摘要
Transformer language models that are trained on vast amounts of data have achieved remarkable success at various NLP benchmarks. Intriguingly, this success is achieved by models that lack an explicit modeling of hierarchical syntactic structures, which were hypothesized by decades of linguistic research to be necessary for good generalization. This naturally leaves a question: to what extent can we further improve the performance of Transformer language models, through an inductive bias that encourages the model to explain the data through the lens of recursive syntactic compositions? Although the benefits of modeling recursive syntax have been shown at the small data and model scales, it remains an open question whether—and to what extent—a similar design principle is still beneficial in the case of powerful Transformer language models that work well at scale. To answer these questions, we introduce Transformer Grammars—a novel class of Transformer language models that combine: (i) the expressive power, scalability, and strong performance of Transformers, and (ii) recursive syntactic compositions, which here are implemented through a special attention mask. We find that Transformer Grammars outperform various strong baselines on multiple syntaxsensitive language modeling evaluation metrics, in addition to sentence-level language modeling perplexity. Nevertheless, we find that the recursive syntactic composition bottleneck harms perplexity on document-level modeling, providing evidence that a different kind of memory mechanism—that works independently of syntactic structures—plays an important role in the processing of long-form text.
更多查看译文
关键词
transformer language models,syntactic inductive biases
AI 理解论文
溯源树
样例
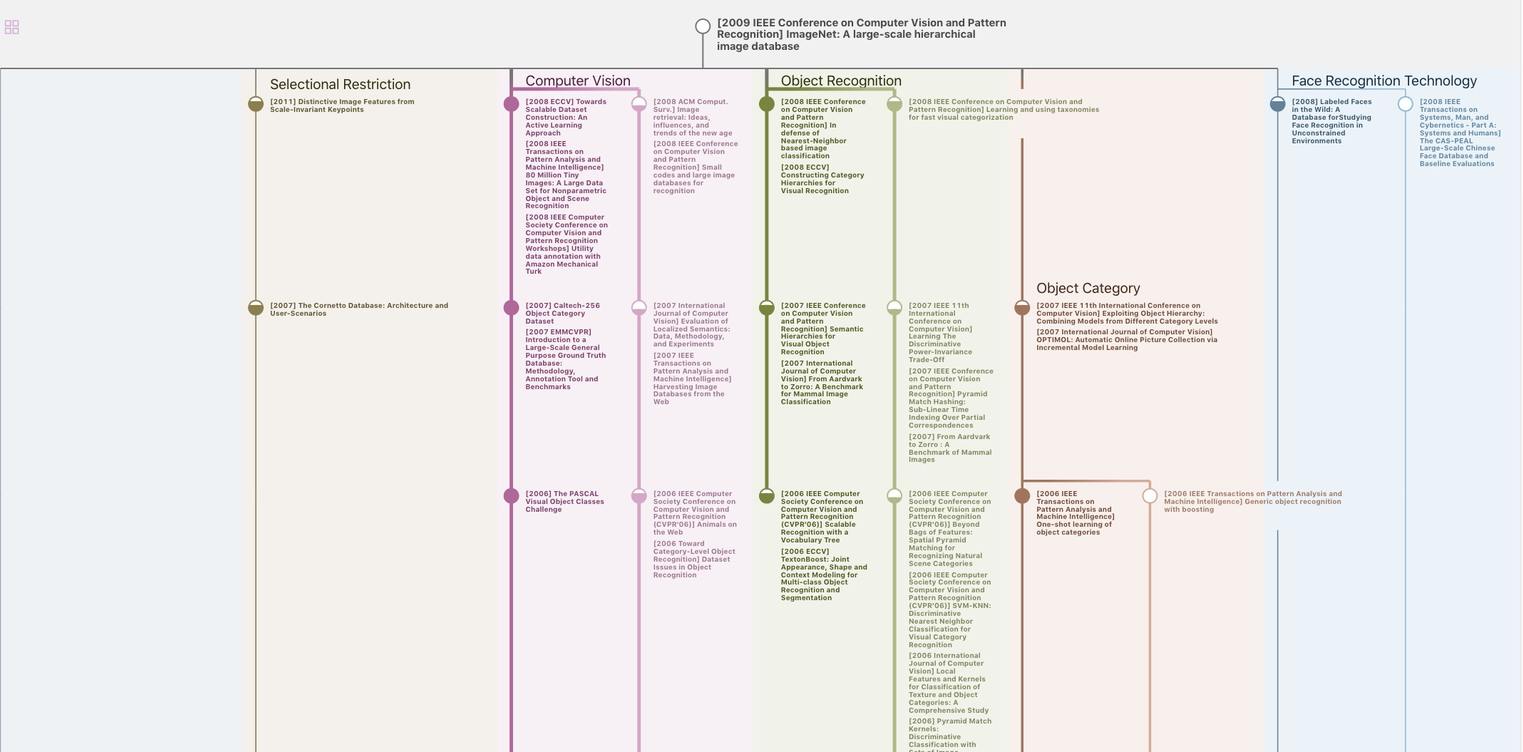
生成溯源树,研究论文发展脉络
Chat Paper
正在生成论文摘要