Human latent-state generalization through prototype learning with discriminative attention
semanticscholar(2021)
摘要
People cannot access the latent causes giving rise to experience. How then do they approximate the high-dimensional feature space of the external world with lower-dimensional internal models that generalize to novel examples or contexts? Here, we developed and tested a theoretical framework that internally identifies states by feature regularity (i.e., prototype states) and selectively attends to features according to their informativeness for discriminating between likely states. To test theoretical predictions, we developed experimental tasks where human subjects first learn through reward-feedback internal models of latent states governing actions associated with multi-feature stimuli. We then analyzed subjects’ response patterns to novel examples and contexts. These combined theoretical and experimental results reveal that the human ability to generalize actions involves the formation of prototype states with flexible deployment of top-down attention to discriminative features. These cognitive strategies underlie the human ability to generalize learned latent states in high-dimensional environments.
更多查看译文
AI 理解论文
溯源树
样例
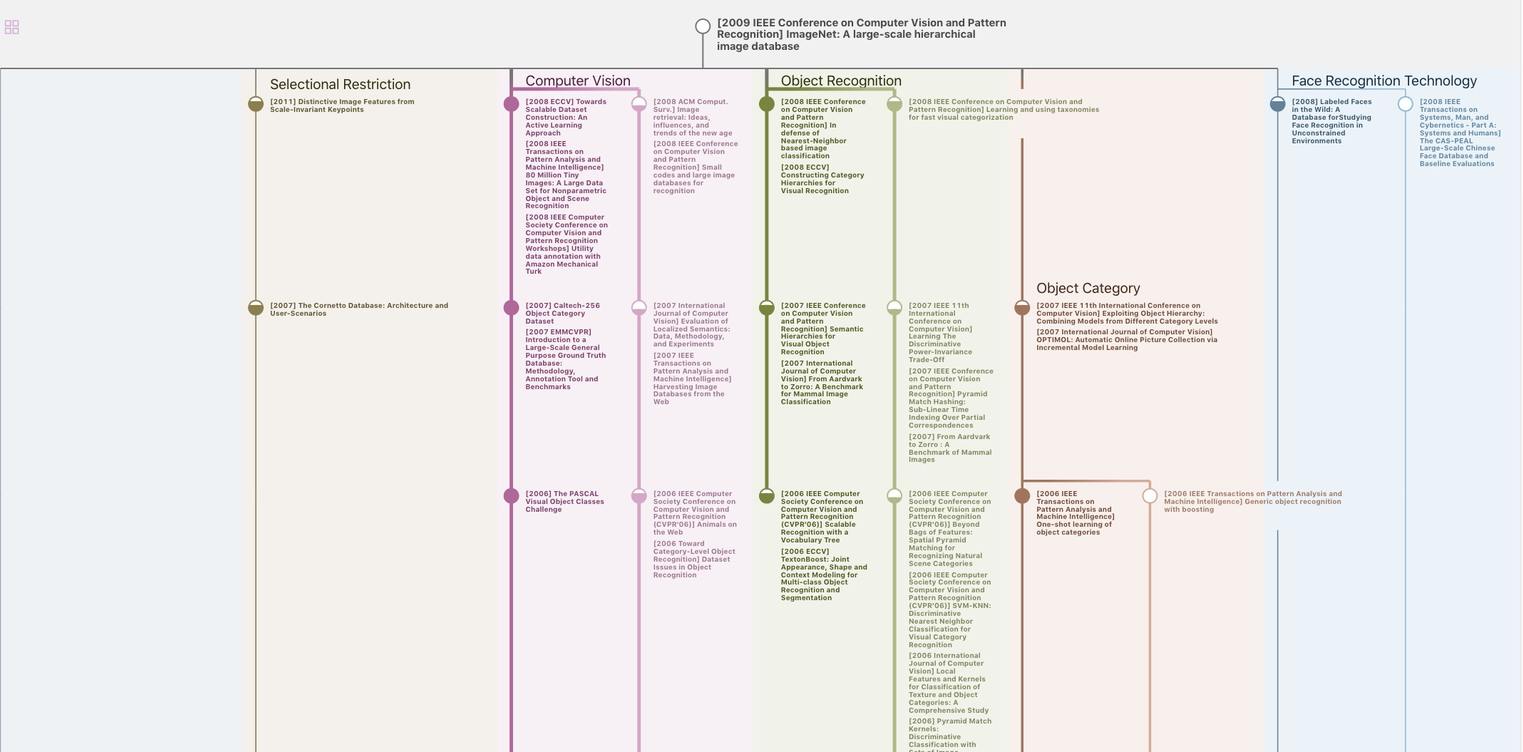
生成溯源树,研究论文发展脉络
Chat Paper
正在生成论文摘要