VDNet: video deinterlacing network based on coarse adaptive module and deformable recurrent residual network
Neural Computing and Applications(2022)
摘要
Interlacing is the technique that overlaps odd lines from an odd frame and even lines from an even frame to increase the perceived frame rate in TV displays without increasing bandwidth. On the other hand, since original frames are not stored, deinterlacing is the technique introduced for reversing this process and restore the original or progressive video. Existing deinterlacing approaches focus on restoring a single interlaced frame without optimally leveraging the temporal information available. In this paper, we propose VDNet , which to the best of our knowledge, is the first deep learning-based deinterlacing framework that considers inter-frame correlation. Our proposed method addresses deinterlacing by splitting the frames and regenerating the missing lines using a simple coarse method (base image sequence) before combining them with the residual image sequence for refinement. For the deinterlaced base image sequence, our data module uses spatial and temporal information to fill in the missing areas and leverages our coarse adaptive module to interpolate them. The residual module then leverages our proposed Deformable Recurrent Residual Network to optimally enhance and aggregate the features extracted from the interlaced video. Our method then refines the base image sequence using the residual image sequence generated from the residual module. After reconstructing the progressive frames, our proposed Spatial-Temporal Correlation Loss uses the information provided by the existing interlaced video to further smooth and boost the deinterlaced output. We perform extensive experiments to demonstrate our proposed VDNet ’s incredible quantitative performance. Moreover, we design our model to be lightweight and efficient.
更多查看译文
关键词
Deinterlacing, Deep learning, Space-time video super-resolution, Video restoration
AI 理解论文
溯源树
样例
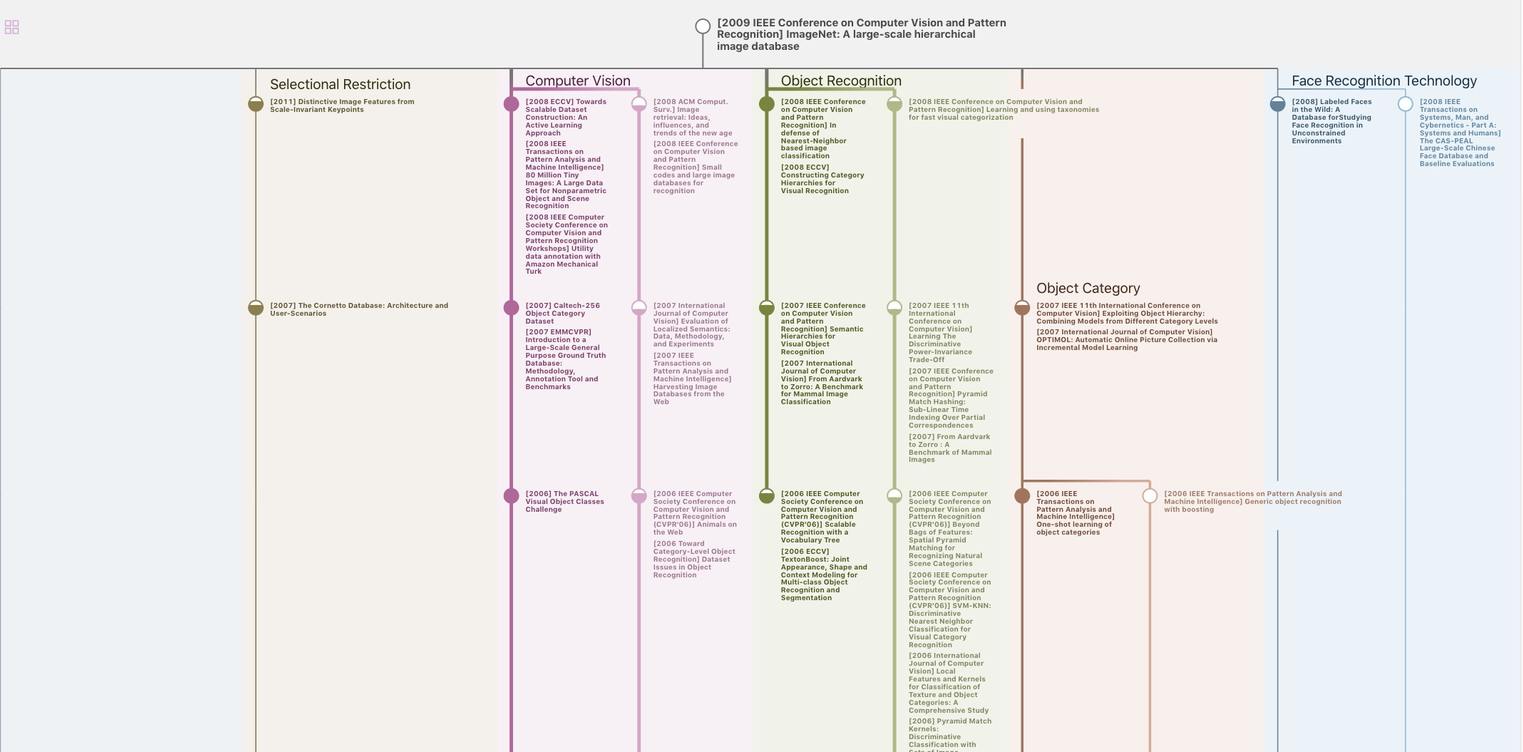
生成溯源树,研究论文发展脉络
Chat Paper
正在生成论文摘要