Efficient Boosted DC Algorithm for Nonconvex Image Restoration with Rician Noise
SIAM JOURNAL ON IMAGING SCIENCES(2022)
摘要
Image deblurring under Rician noise has attracted considerable attention in imaging science. Frequently appearing in medical imaging, Rician noise leads to an interesting nonconvex optimization problem, termed as the MAP-Rician model, which is based on the Maximum a Posteriori (MAP) estimation approach. As the MAP-Rician model is deeply rooted in Bayesian analysis, we want to understand its mathematical analysis carefully. Moreover, one needs to properly select a suitable algorithm for tackling this nonconvex problem to get the best performance. This paper investigates both issues. Indeed, we first present a theoretical result about the existence of a minimizer for the MAP-Rician model under mild conditions. Next, we aim to adopt an efficient boosted difference of convex functions algorithm (BDCA) to handle this challenging problem. Basically, BDCA combines the classical difference of convex functions algorithm (DCA) with a backtracking line search, which utilizes the point generated by DCA to define a search direction. In particular, we apply a smoothing scheme to handle the nonsmooth total variation (TV) regularization term in the discrete MAP-Rician model. Theoretically, using the Kurdyka-Lojasiewicz (KL) property, the convergence of the numerical algorithm can be guaranteed. We also prove that the sequence generated by the proposed algorithm converges to a stationary point with the objective function values decreasing monotonically. Numerical simulations are then reported to clearly illustrate that our BDCA approach outperforms some state-of-the-art methods for both medical and natural images in terms of image recovery capability and CPU-time cost.
更多查看译文
关键词
image restoration,Rician noise,MAP-Rician model,BDCA
AI 理解论文
溯源树
样例
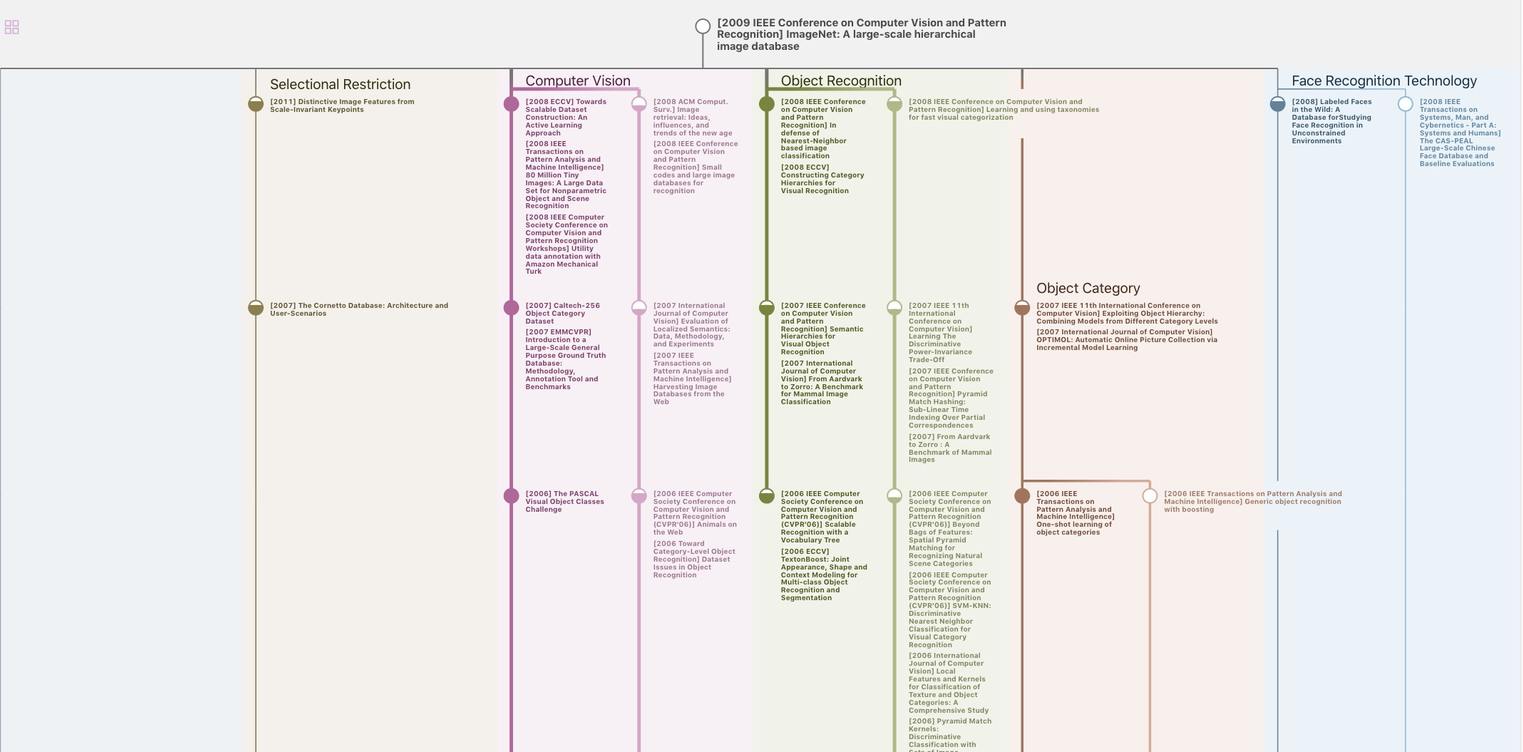
生成溯源树,研究论文发展脉络
Chat Paper
正在生成论文摘要