Learning Bi-Typed Multi-Relational Heterogeneous Graph Via Dual Hierarchical Attention Networks
IEEE Transactions on Knowledge and Data Engineering(2023)
摘要
Bi-typed multi-relational heterogeneous graph (BMHG) is one of the most common graphs in practice, for example, academic networks, e-commerce user behavior graph and enterprise knowledge graph. It is a critical and challenge problem on how to learn the numerical representation for each node to characterize subtle structures. However, most previous studies treat all node relations in BMHG as the same class of relation without distinguishing the different characteristics between the intra-type relations and inter-type relations of the bi-typed nodes, causing the loss of significant structure information. To address this issue, we propose a novel Dual Hierarchical Attention Networks (DHAN) based on the bi-typed multi-relational heterogeneous graphs to learn comprehensive node representations with the intra-type and inter-type attention-based encoder under a hierarchical mechanism. Specifically, the former encoder aggregates information from the same type of nodes, while the latter aggregates node representations from its different types of neighbors. Moreover, to sufficiently model node multi-relational information in BMHG, we adopt a newly proposed hierarchical mechanism. By doing so, the proposed dual hierarchical attention operations enable our model to fully capture the complex structures of the bi-typed multi-relational heterogeneous graphs. Experimental results on various tasks against the state-of-the-arts sufficiently confirm the capability of DHAN in learning node representations on the BMHGs.
更多查看译文
关键词
Bi-typed multi-relational heterogeneous graph,graph learning,dual hierarchical attention networks,GNNs
AI 理解论文
溯源树
样例
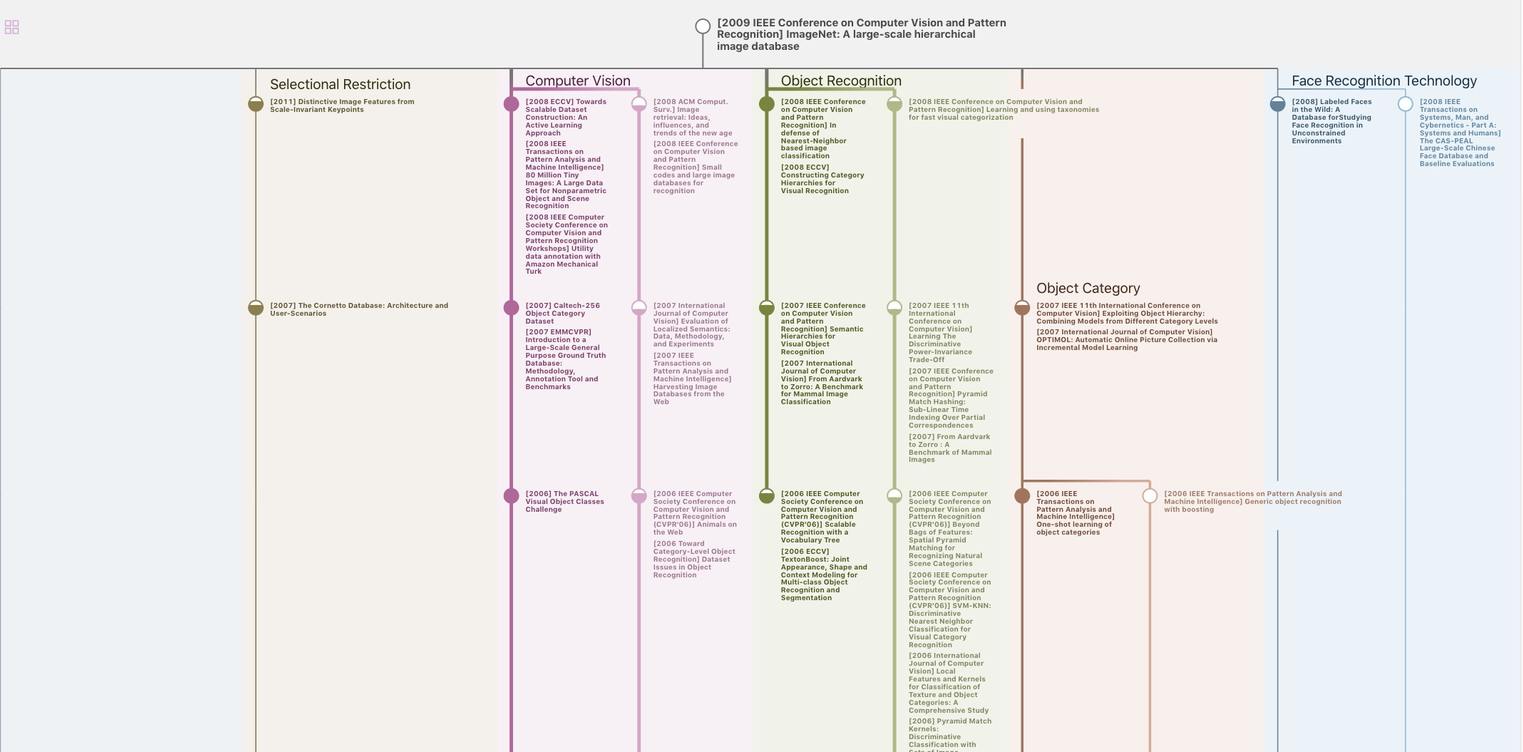
生成溯源树,研究论文发展脉络
Chat Paper
正在生成论文摘要