Chex-Mix: Combining Homomorphic Encryption with Trusted Execution Environments for Oblivious Inference in the Cloud.
IACR Cryptol. ePrint Arch.(2023)
摘要
Data, when coupled with state-of-the-art machine learning models, can enable remarkable applications. But, there exists an underlying tension: users wish to keep their data private, and model providers wish to protect their intellectual property. Homomorphic encryption (HE) and multi-party computation (MPC) techniques have been proposed as solutions to this problem; however, both techniques require model providers to fully trust the server performing the machine learning computation. This limits the scale of inference applications, since it prevents model providers from leveraging shared public cloud infrastructures. In this work, we present CHEX- MIX, a solution to the problem of privacy-preserving machine learning between two mutually distrustful parties in an untrusted cloud setting. CHEX-MIX relies on a combination of HE and trusted execution environments (TEEs), using HE to provide clients with confidentiality guarantees, and TEEs to provide model providers with confidentiality guarantees and protect the integrity of computation from malicious cloud adversaries. Unlike prior solutions to this problem, such as multi-key HE, single-key HE, MPC, or TEE-only techniques, our solution assumes that both the client and the cloud can be malicious, makes no collusion assumptions, and frees model providers from needing to maintain private online infrastructures. Our results show that CHEX- MIX can execute at high efficiency, with low communication cost, while providing security guarantees unaddressed by prior work. Compared to a recent multi-key HE work that allows partial cloud offload, for example, CHEX- MIX achieves a 3x lower communication cost and a 3x faster computation time.
更多查看译文
关键词
Chex-Mix,confidentiality guarantees,homomorphic encryption,machine learning computation,malicious cloud adversaries,MPC,multi-key HE,multiparty computation techniques,privacy-preserving machine learning,public cloud infrastructures,single-key HE,TEE-only techniques,trusted execution environments
AI 理解论文
溯源树
样例
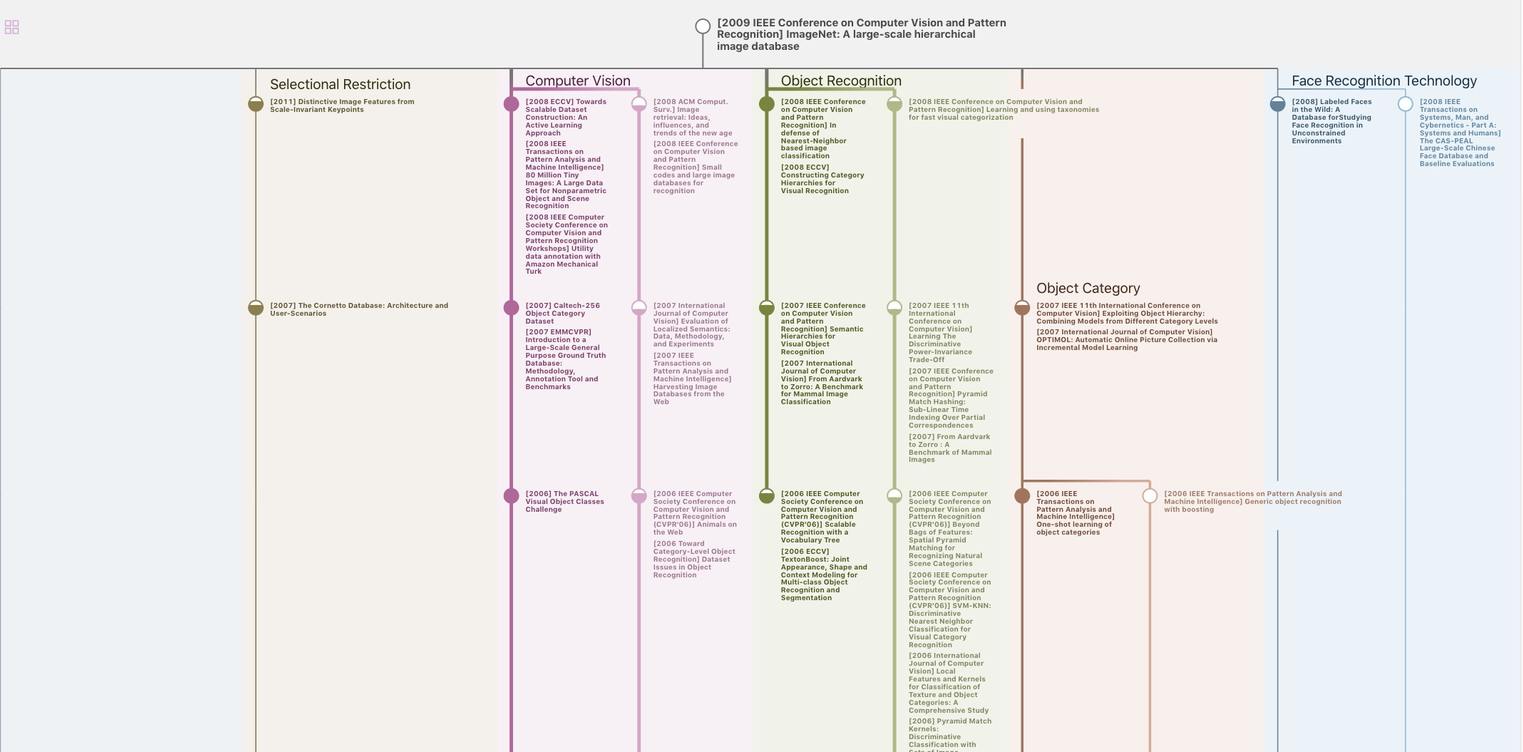
生成溯源树,研究论文发展脉络
Chat Paper
正在生成论文摘要