Supervised versus unsupervised learning: approaching optimal memory retrieval in Hopfield networks
semanticscholar(2022)
摘要
The Hebbian unlearning algorithm, i.e. an unsupervised local procedure used to improve the retrieval properties in Hopfield-like neural networks, is numerically compared to a supervised algorithm to train a linear symmetric perceptron. We analyze the stability of the stored memories, which naturally maps the problem into a constraint satisfaction problem. Basins of attraction obtained by the Hebbian unlearning technique are found to be comparable in size to those obtained in the symmetric perceptron, while the two algorithms are found to converge in the same region of Gardner’s space of interactions, having followed similar learning paths. A geometric interpretation of Hebbian unlearning is proposed to explain its optimal performances.
更多查看译文
AI 理解论文
溯源树
样例
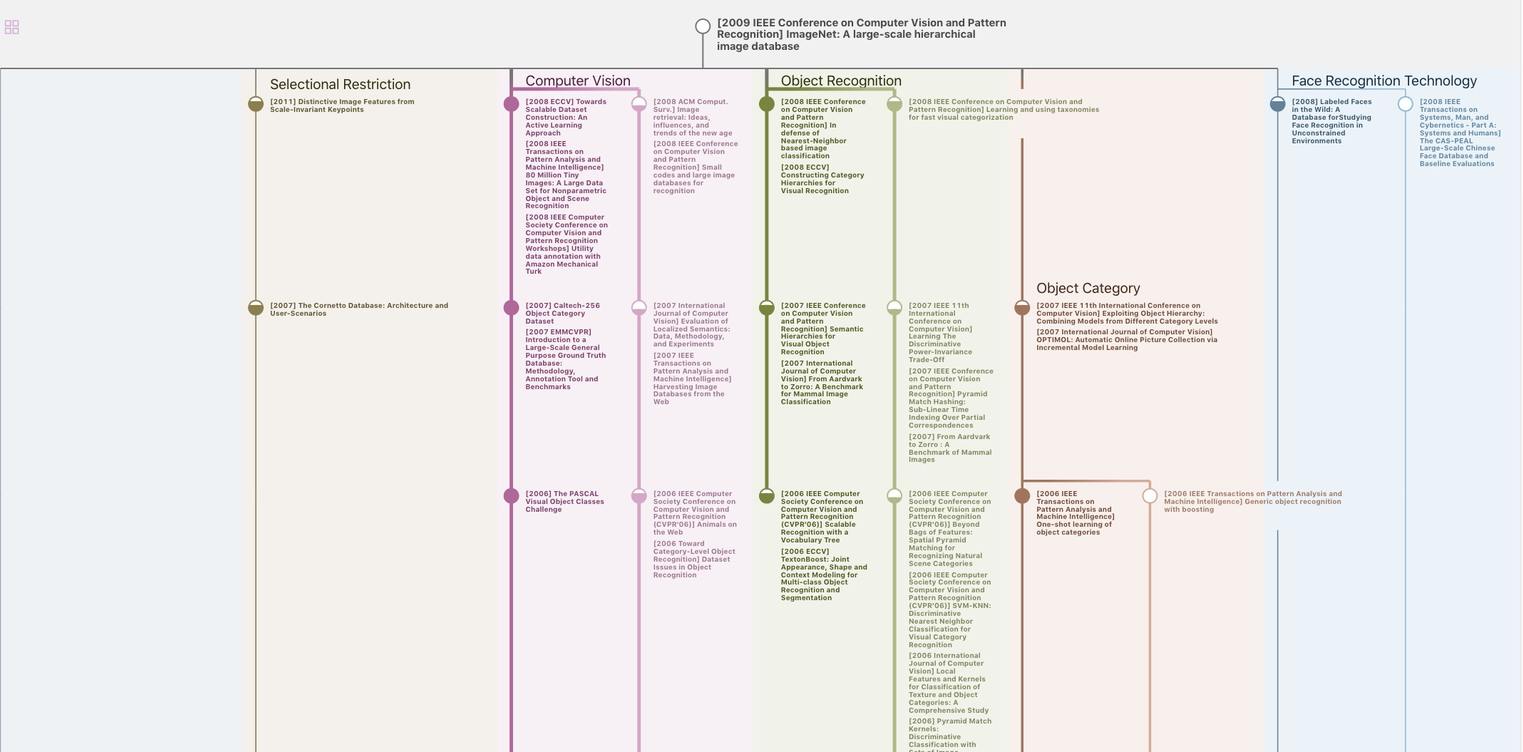
生成溯源树,研究论文发展脉络
Chat Paper
正在生成论文摘要