Meta-Learning for Medical Image Segmentation
semanticscholar(2020)
摘要
Human beings learn by building upon the prior experiences. We can learn a task quickly just by observing or experiencing few instances of the task. The quest of building an artificial system that mimics human, needs to be able to learn from prior tasks with few example points. In this thesis, we adopt the learning process based on this concept of learning to learn from prior experience with few shots in medical settings. The meta-learning , unlike the conventional learning from scratch, is based on learning from a number of task that are comprised of N-classes and K-shot instances. With the aim of adopting the meta-learning process in medical setting specially for polyp segmentation, previous works on the field of meta-learning and polyp segmentation were studied. The terminologies and problem setting for optimization based meta-learning processes were studied and applied to our task of polyp segmentation. The data pipeline for task generation process was constructed to sample tasks from four different sources of polyp images. Unlike prior approaches on polyp segmentation in which models were trained on a large number of data points from one particular data source, this work is based on using the prior learning and adapts to tasks comprised of few images from different sources. The implicit model agnostic meta-learning (iMAML) algorithm was adopted for meta-learn the segmentation problem. The results show that for the polyp segmentation problem, models metalearned on tasks with a few shot instances produced better result than the model trained by merging datasets from different sources. However, it was not able to match the results from a model that was trained on a single data source. The results also indicate that by increasing the number of instances and diversity of data class or source in a task, the meta-learner can exhibit better generalization capability. Finally, the thesis is concluded with some remarks and queries that came up during the experiments. The future research directions and the relevancy of meta-learning to improve the learning and generalization process in medical domain is also discussed.
更多查看译文
AI 理解论文
溯源树
样例
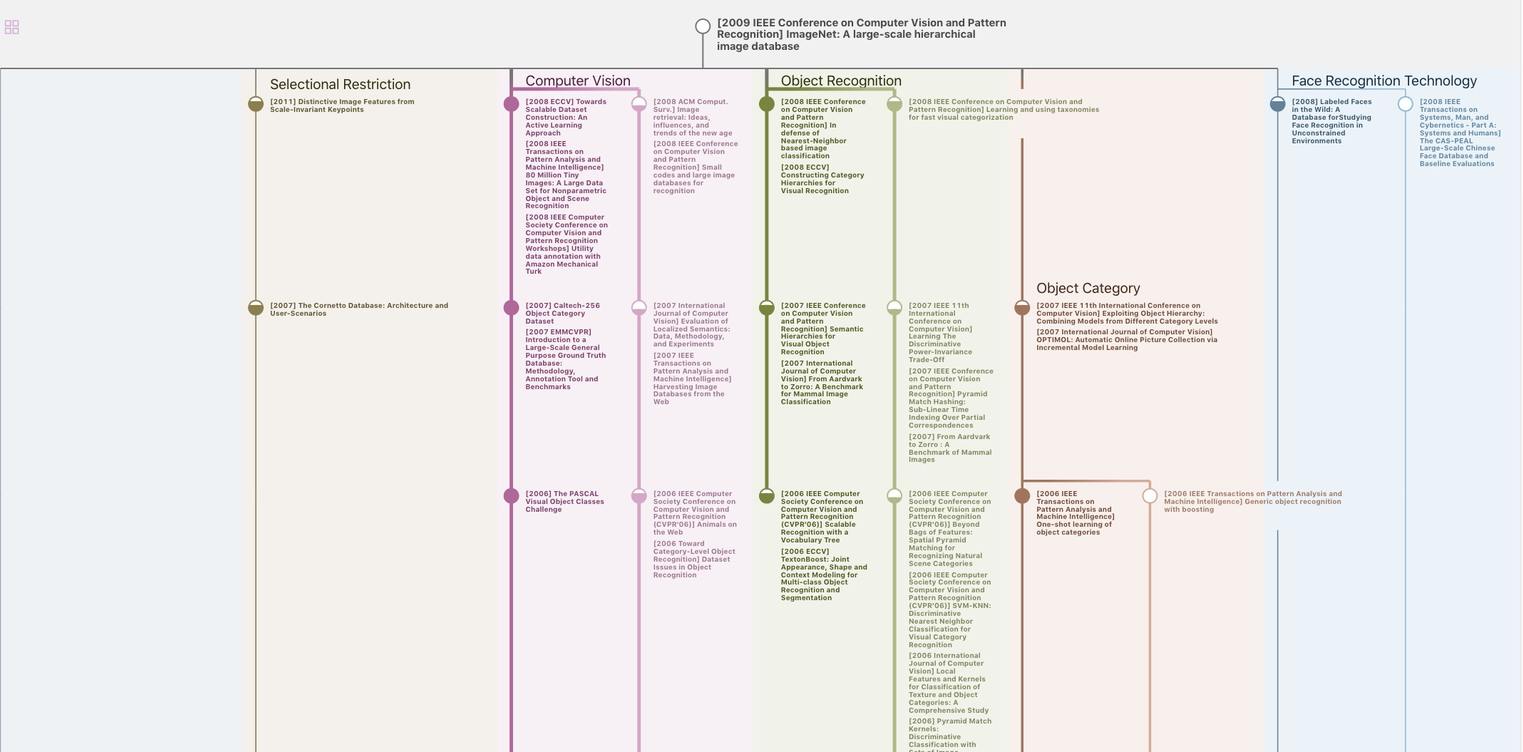
生成溯源树,研究论文发展脉络
Chat Paper
正在生成论文摘要