Submodular Maximization in Clean Linear Time
NeurIPS 2022(2020)
摘要
In this paper, we provide the first deterministic algorithm that achieves the tight 1 − 1 /e approximation guarantee for submodular maximization under a cardinality (size) constraint while making a number of queries that scales only linearly with the size of the ground set n . To complement our result, we also show strong information-theoretic lower bounds. More specifically, we show that when the maximum cardinality allowed for a solution is constant, no algorithm making a sub-linear number of function evaluations can guarantee any constant approximation ratio. Furthermore, when the constraint allows the selection of a constant fraction of the ground set, we show that any algorithm making fewer than Ω( n/ log( n )) function evaluations cannot perform better than an algorithm that simply outputs a uniformly random subset of the ground set of the right size. We then provide a variant of our deterministic algorithm for the more general knapsack constraint, which is the first linear-time algorithm that achieves 1 / 2-approximation guarantee for this constraint. Finally, we extend our results to the general case of maximizing a monotone submodular function subject to the intersection of a p -set system and multiple knapsack constraints. We extensively evaluate the performance of our algorithms on multiple real-life machine learning applications, including movie recommendation, location summarization, twitter text summarization and video summarization.
更多查看译文
关键词
Submodular maximization,query complexity,cardinality constraint,Knapsack constraint,p-set system constraint,information-theoretic lower bound
AI 理解论文
溯源树
样例
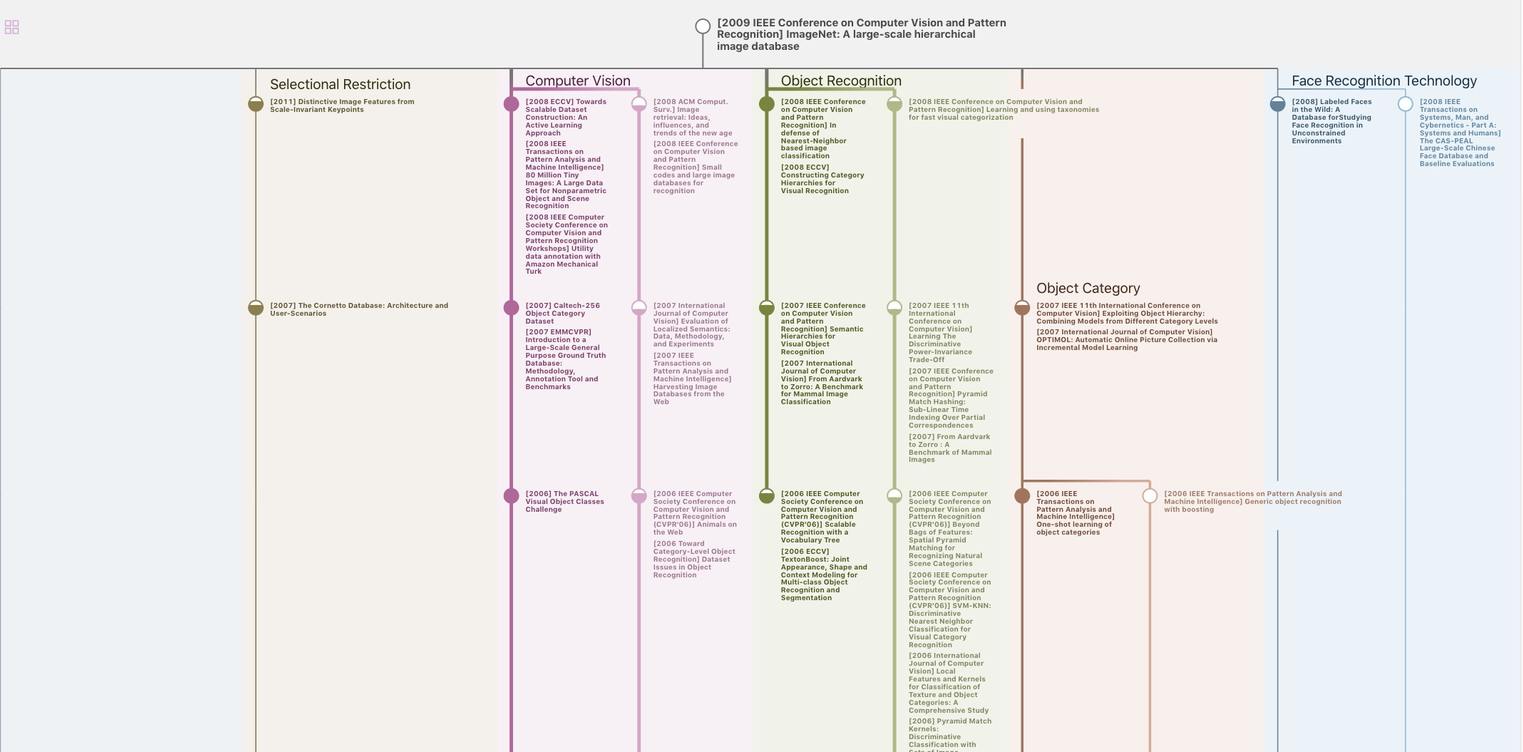
生成溯源树,研究论文发展脉络
Chat Paper
正在生成论文摘要