Distributed Task Scheduling in Serverless Edge Computing Networks for the Internet of Things: A Learning Approach
IEEE Internet of Things Journal(2022)
摘要
By delegating the infrastructure management, such as provisioning or scaling to third-party providers, serverless edge computing has recently been widely adopted in several applications, especially Internet of Things (IoT) applications. Task scheduling is a critical issue in serverless edge computing as it significantly impacts the quality of user experience. In contrast to the centralized scheduling in the cloud center, serverless edge task scheduling is more challenging due to the heterogeneous and resource-constrained nature of edge resources. This article aims to study the distributed task scheduling for the IoT in serverless edge computing networks, in which heterogeneous serverless edge computing nodes are rational individuals with interests to optimize their own scheduling utility while the nodes only have access to local observations. The task scheduling competition process is formulated as a partially observable stochastic game (POSG) to enable serverless edge computing nodes to noncooperatively schedule tasks and allocate computing resources depending on their locally observed system state, which takes into account the associated task generation state, data queue state, communication channel state, and previous computing resource allocation state. To solve the proposed POSG and deal with the partial observability, a multiagent task scheduling algorithm based on the dueling double deep recurrent
$Q$
-network (D3RQN) method is developed to approximate the optimal task scheduling and resource allocation solution. Finally, extensive simulation experiments are conducted to validate the effectiveness and superiority of the proposed scheme.
更多查看译文
关键词
Deep reinforcement learning (DRL),distributed task scheduling,Internet of Things (IoT),serverless edge computing,stochastic game
AI 理解论文
溯源树
样例
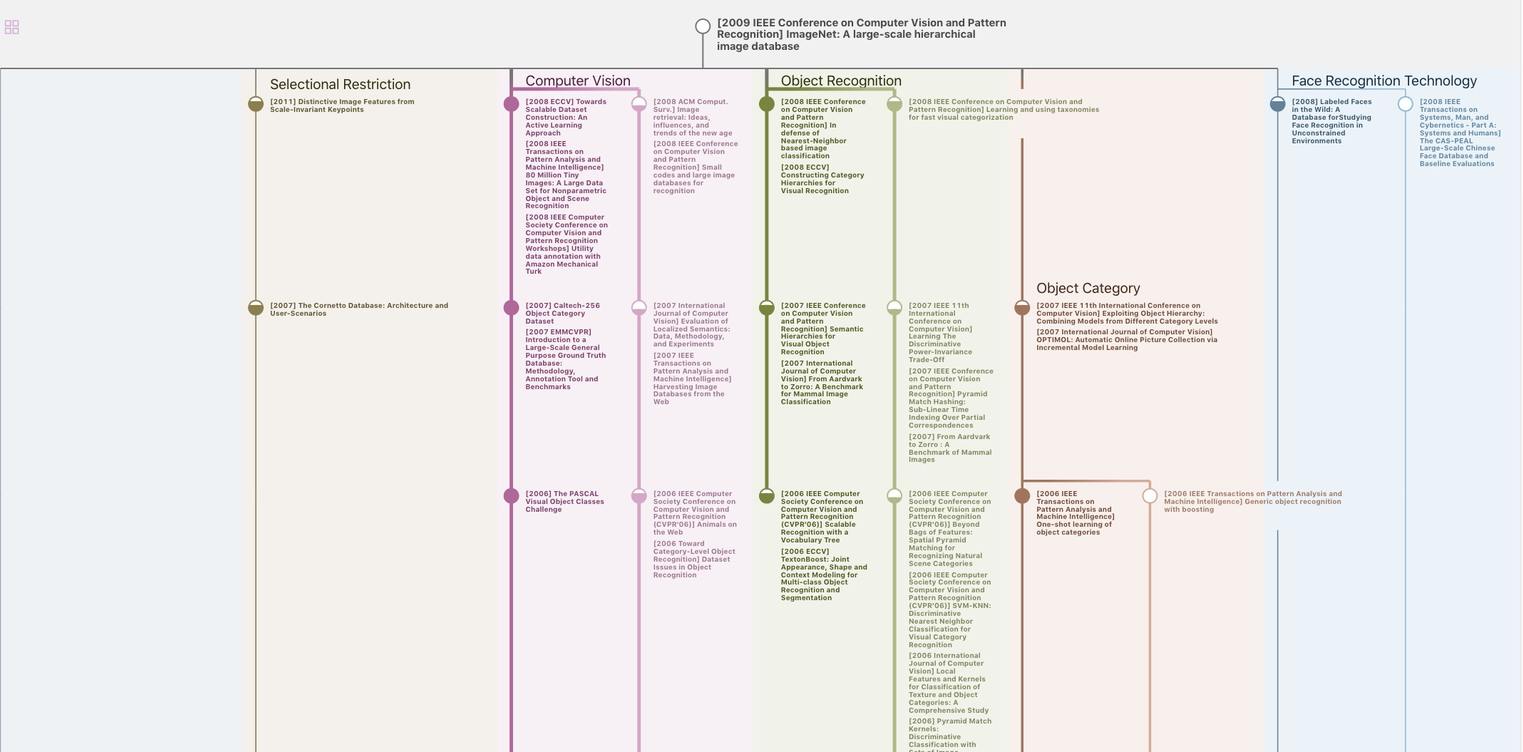
生成溯源树,研究论文发展脉络
Chat Paper
正在生成论文摘要