Sequential Decisions from Sampling:
Cambridge University Press eBooks(2023)
摘要
Sequential decisions from sampling are common in daily life: we often explore alternatives sequentially, decide when to stop such exploration process, and use the experience acquired during sampling to make a choice for what is expected to be the best option. In decisions from experience, theories of sampling and experiential choice are unable to explain the decision of when to stop the sequential exploration of alternatives. In this chapter, we propose a mechanism to inductively generate stopping decisions, and we demonstrate its plausibility in a large and diverse human data set of the binary choice sampling paradigm. Our proposed stopping mechanism relies on the choice process of a theory of experiential choice, Instance-Based Learning Theory (IBLT). The new stopping mechanism tracks the relative prediction errors of the two options during sampling, and stops when such difference is close to zero. Our results from simulation are able to accurately predict human stopping decisions distributions in the dataset. This model provides an integrated theoretical account of decisions from experience, where the stopping decisions are generated inductively from the sampling process.
更多查看译文
关键词
decisions
AI 理解论文
溯源树
样例
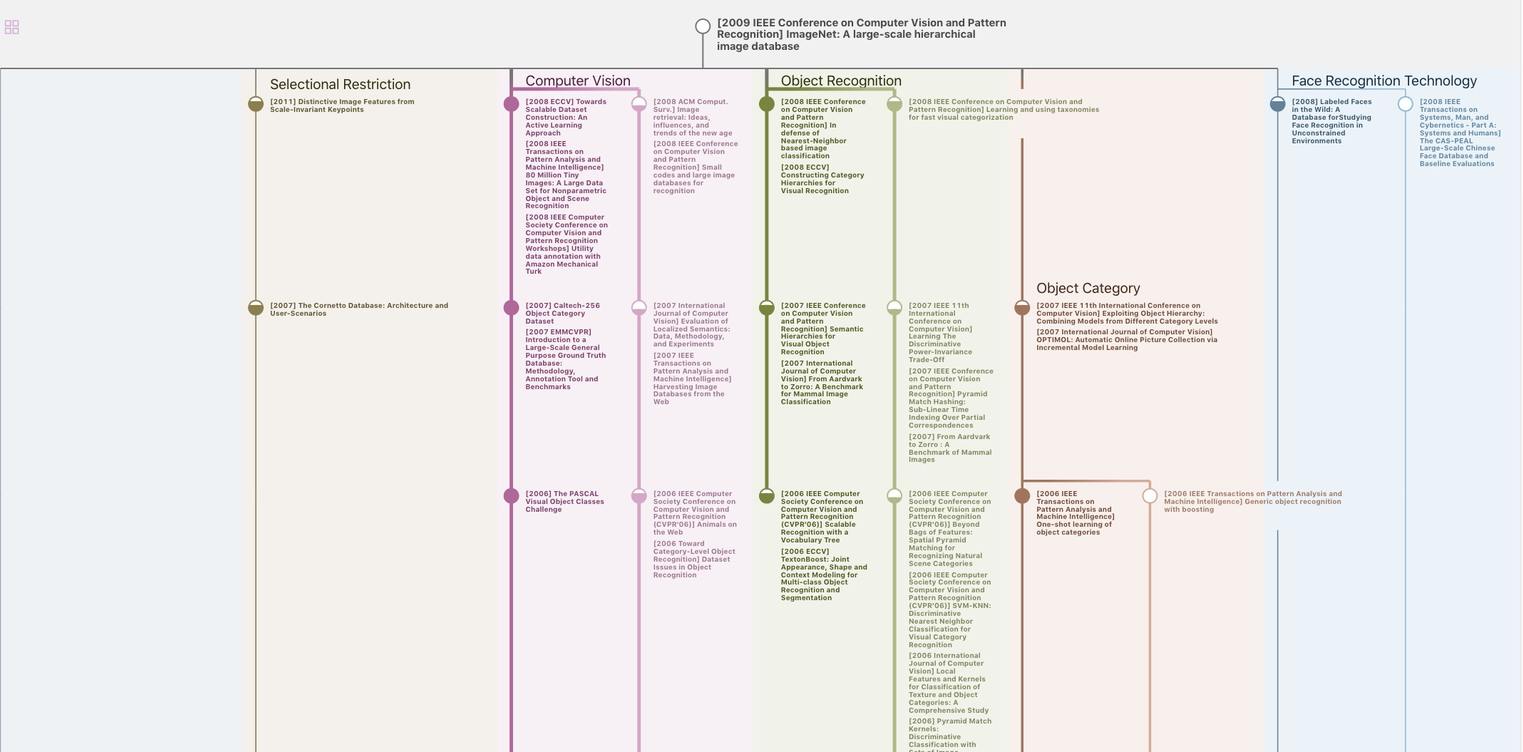
生成溯源树,研究论文发展脉络
Chat Paper
正在生成论文摘要