Improving 3D Metric GPR Imaging Using Automated Data Collection and Learning-Based Processing
IEEE Sensors Journal(2022)
摘要
Ground Penetrating Radar (GPR) is one of the most important non-destructive evaluation (NDE) devices to detect subsurface objects (i.e., rebars, utility pipes) and reconstruct the underground scene. There are two challenges for GPR-based inspection, which are GPR data collection and 3D subsurface object imaging. To address these challenges, we first propose a robotic solution that automates the GPR data collection process with a free motion pattern. It facilitates the 3D metric GPR imaging by tagging the pose with GPR measurement in real-time. Moreover, to improve the 3D GPR imaging, we introduce a learning-based GPR data analysis method, which includes a noise removal module to clear the background noise in raw GPR data and a Convolutional Recurrent Neural Network (CRNN) to estimate the dielectric value of subsurface medium in each GPR B-scan data. We use both field and synthetic data to verify the proposed methods. Experimental results demonstrate that our proposed methods can achieve higher performance and faster processing speed in 3D GPR imaging than baseline methods.
更多查看译文
关键词
Back-projection (BP) algorithm,ground penetrating radar (GPR),deep neural network (DNN),non-destructive evaluation (NDE),robotics
AI 理解论文
溯源树
样例
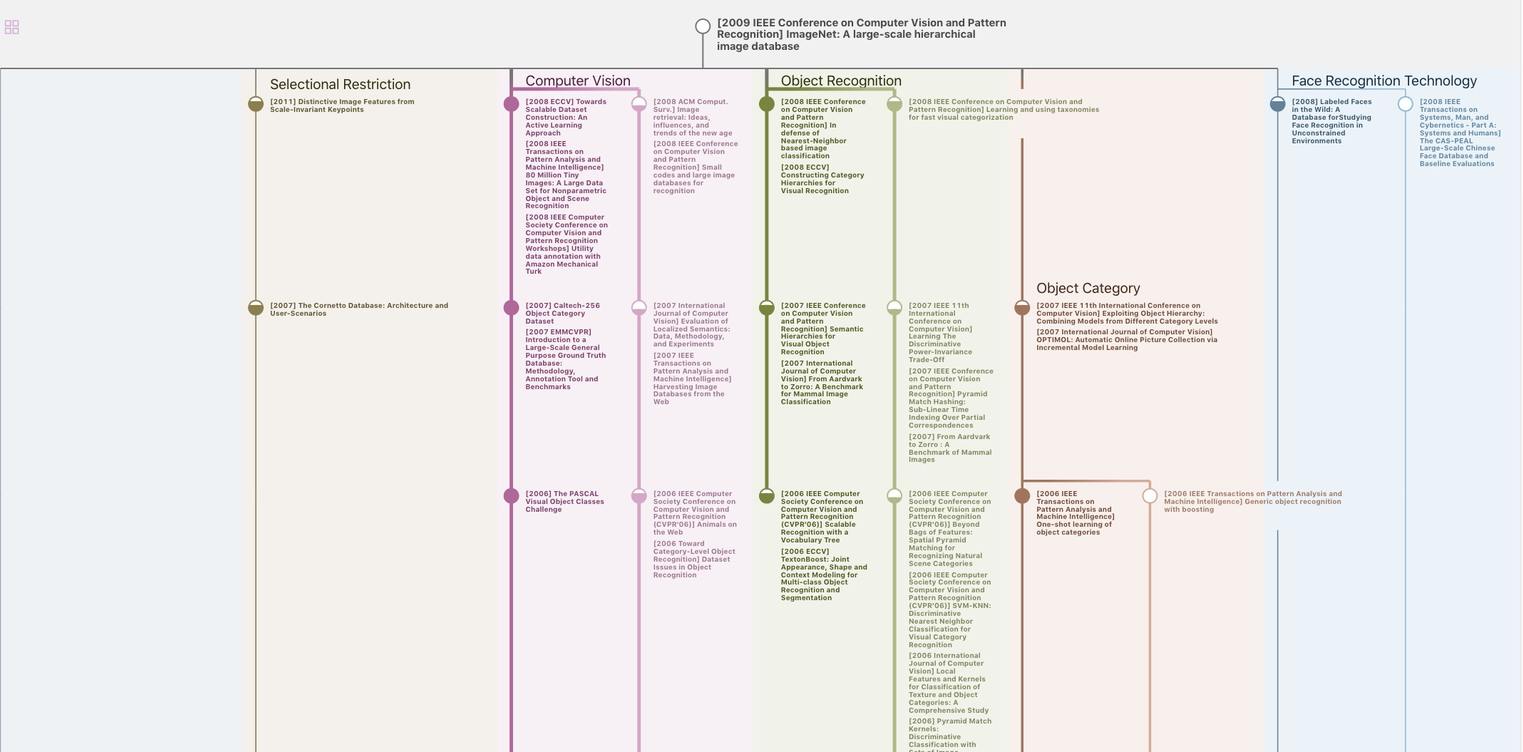
生成溯源树,研究论文发展脉络
Chat Paper
正在生成论文摘要