Ray Priors through Reprojection: Improving Neural Radiance Fields for Novel View Extrapolation
IEEE Conference on Computer Vision and Pattern Recognition(2022)
摘要
Neural Radiance Fields (NeRF) [22] have emerged as a potent paradigm for representing scenes and synthesizing photo-realistic images. A main limitation of conventional NeRFs is that they often fail to produce high-quality renderings under novel viewpoints that are significantly different from the training viewpoints. In this paper, instead of ex-ploiting few-shot image synthesis, we study the novel view extrapolation setting that (1) the training images can well describe an object, and (2) there is a notable discrepancy between the training and test viewpoints' distributions. We present RapNeRF (RAy Priors) as a solution. Our insight is that the inherent appearances of a 3D surface's arbitrary visible projections should be consistent. We thus propose a random ray casting policy that allows training unseen views using seen views. Furthermore, we show that a ray atlas pre-computed from the observed rays' viewing directions could further enhance the rendering quality for ex-trapolated views. A main limitation is that RapNeRF would remove the strong view-dependent effects because it lever-ages the multi-view consistency property.
更多查看译文
关键词
Image and video synthesis and generation, 3D from multi-view and sensors
AI 理解论文
溯源树
样例
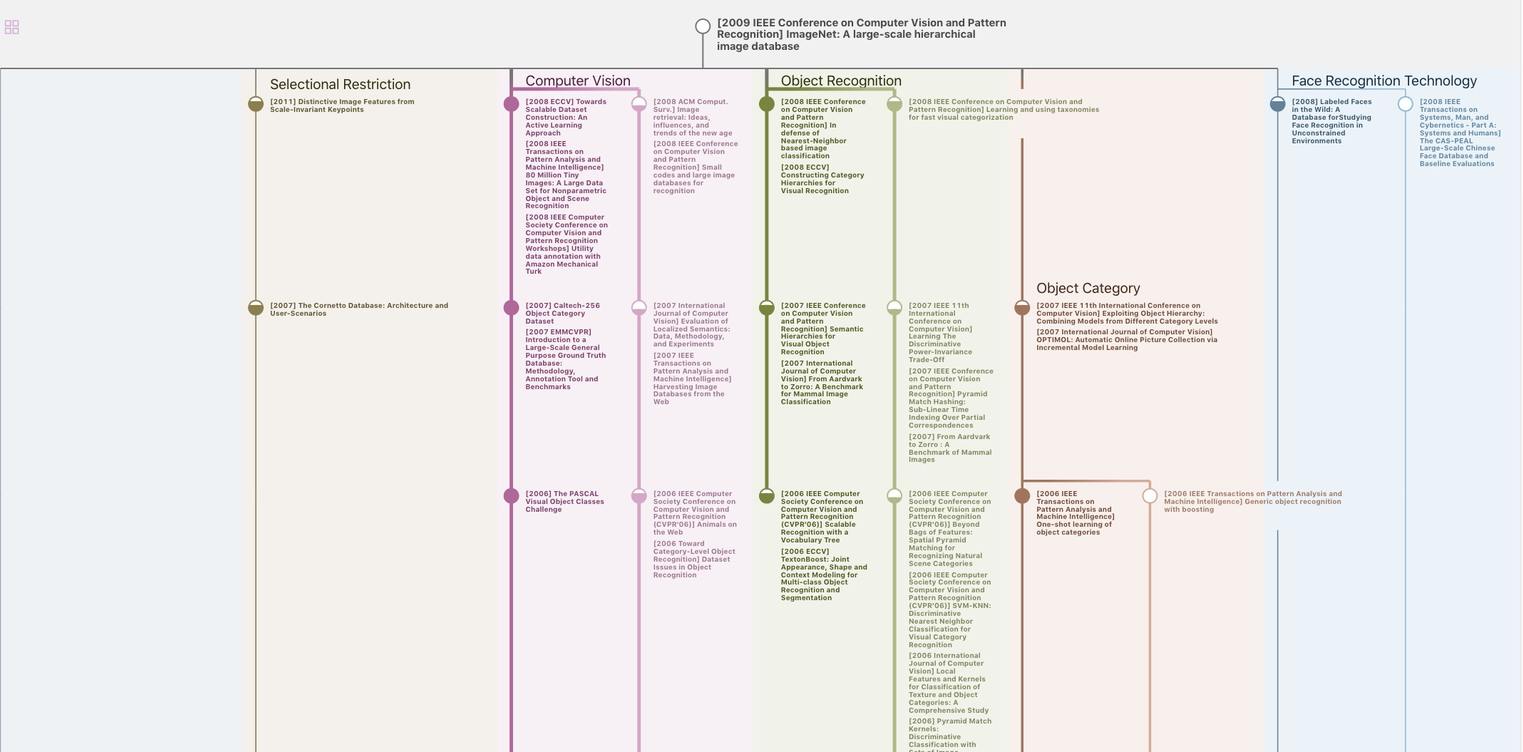
生成溯源树,研究论文发展脉络
Chat Paper
正在生成论文摘要